Deep Embedding Based Tensor Incomplete Multi-view Clustering
DIGITAL SIGNAL PROCESSING(2024)
摘要
The majority of multi-view data are extracted from real life, which often lose information in some views. To solve this problem, existing incomplete multi-view clustering algorithms explore the valuable information from incomplete data while populating the missing information. Nevertheless, they suffer from the following two limitations: 1) The non-linear relationships of high-dimensional available data are not considered. 2) Although some methods utilize information from different views to fill in missing information, they still cannot precisely explore the missing information of each view. To this end, this article proposes a novel one-step incomplete multi-view framework, referred to as deep embedding based tensor incomplete multi-view clustering (DETIMC). Concretely, in this framework, the high-dimensional available data are projected into the low-dimensional embedding space by deep non-negative matrix factorization (NMF), which can obtain a clean space while capturing the complex non-linear relationship of available data. Moreover, a novel double-enhanced missing-view inferring strategy is developed, in which the weighted higher-order information of different views and the clustering structure information are simultaneously exploited. Comprehensive experiments on several benchmark datasets exhibit the superiority of DETIMC over conventional and state-of-the-art algorithms.
更多查看译文
关键词
Deep NMF,Incomplete view,Multi-view subspace clustering,Double-enhanced missing-view inferring
AI 理解论文
溯源树
样例
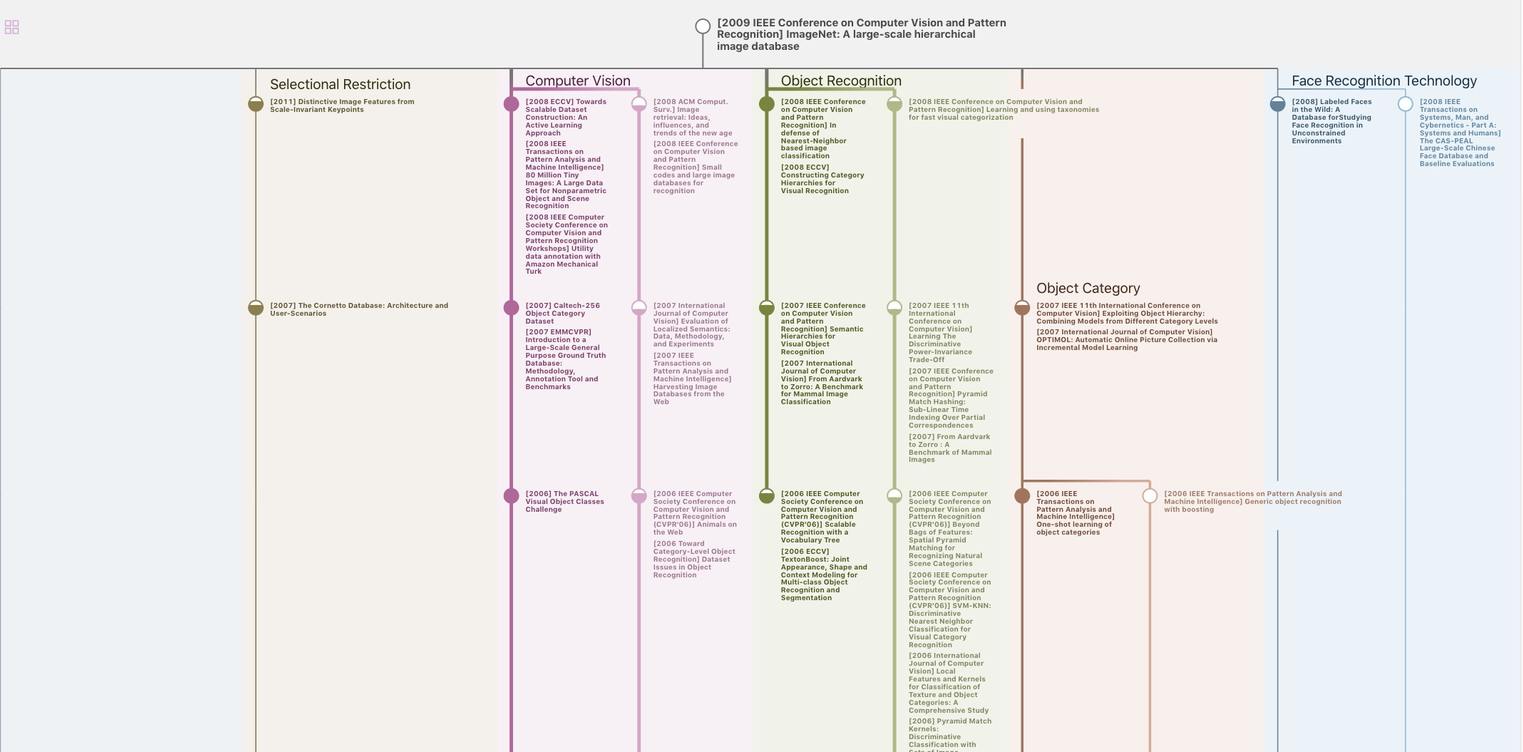
生成溯源树,研究论文发展脉络
数据免责声明
页面数据均来自互联网公开来源、合作出版商和通过AI技术自动分析结果,我们不对页面数据的有效性、准确性、正确性、可靠性、完整性和及时性做出任何承诺和保证。若有疑问,可以通过电子邮件方式联系我们:report@aminer.cn