Sparse Agent Transformer for Unified Voxel and Image Feature Extraction and Fusion
INFORMATION FUSION(2024)
摘要
Current 3D multi-modal perception methods have a shortage of the capability to efficiently summarize and simplify information when extracting features from extensive sparse 3D data, which results in challenges in achieving a balance between accuracy and speed. In this paper, we propose a novel multi-modal transformer backbone named Sparse Agent Transformer (SAT), which is based on an agent-based approach from the perspective of information abstraction and interaction. In the context of extracting sparse features from a single modality, we suggest a sparse agent attention approach that does not rely on conventional grouping token attention. This method initially compresses features from the token to the agent, followed by interactions between the agents and feedback to the token. To speed up the process of merging cross-model data, we investigated the use of agent-based cross-modal fusion techniques between voxels and images, which uses agent-based cross-modal fusion techniques instead of using tokens directly to speed up the fusion process. Extensive experiments on the Nuscenes dataset show that our model achieves state-of-the-art performance in 3D detection and bird’s eye view (BEV) segmentation.
更多查看译文
关键词
3D feature extraction,Multi-modal perception,Sparse agent,Transformer
AI 理解论文
溯源树
样例
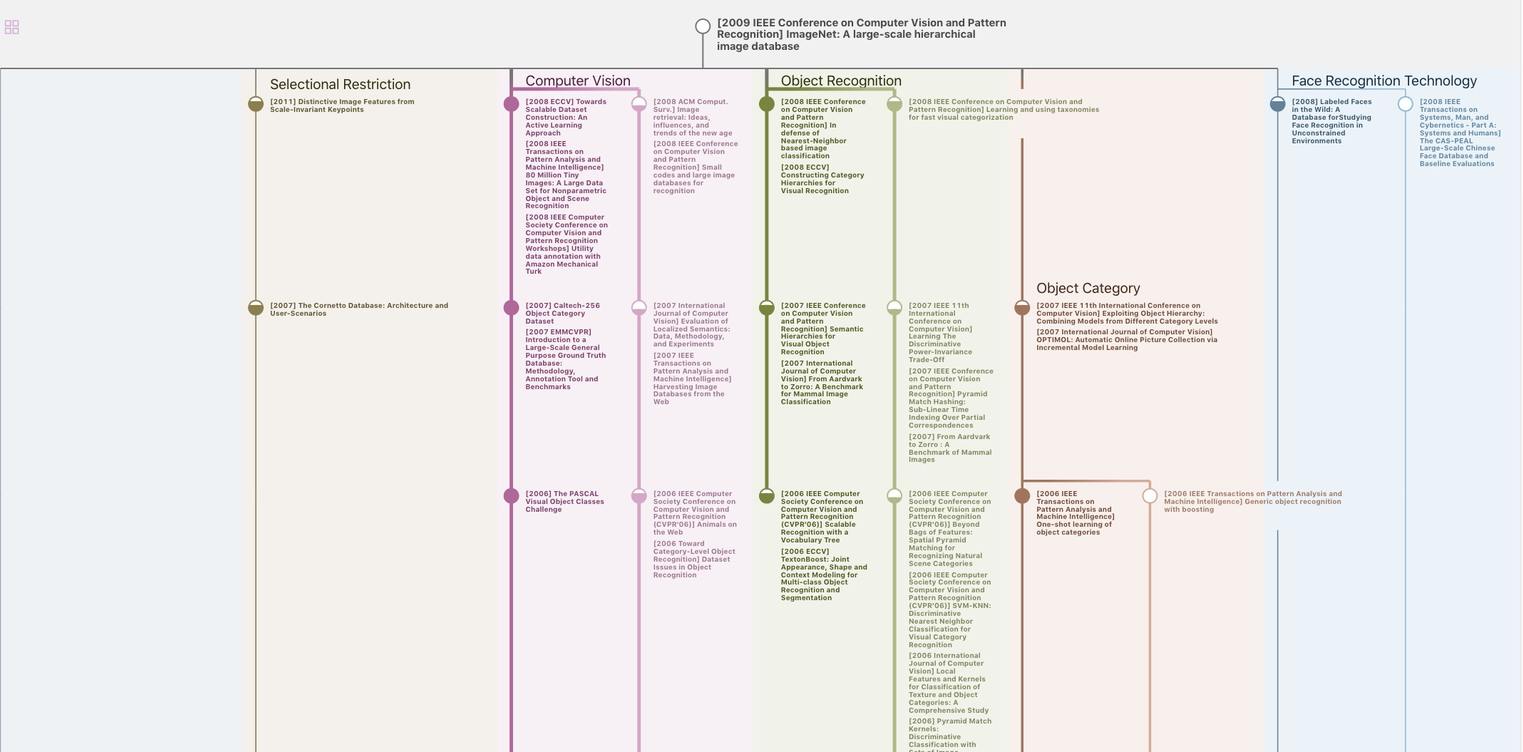
生成溯源树,研究论文发展脉络
数据免责声明
页面数据均来自互联网公开来源、合作出版商和通过AI技术自动分析结果,我们不对页面数据的有效性、准确性、正确性、可靠性、完整性和及时性做出任何承诺和保证。若有疑问,可以通过电子邮件方式联系我们:report@aminer.cn