Learning Cooperative Strategies in Multi-Agent Encirclement Games with Faster Prey Using Prior Knowledge
Neural Computing and Applications(2024)
摘要
Multi-agent encirclement with collision avoidance constitutes a common challenge in the multi-agent confrontation domain, wherein the focus lies in the development of cooperative strategies among agents. Previous studies encountered difficulties in addressing the dynamic encirclement of faster prey in obstacles environment. This paper introduces a novel multi-agent deep reinforcement learning approach based on prior knowledge. It is dedicated to exploring the multi-agent encirclement with collision avoidance task involving slower multiple pursuers collaboratively encircling faster prey in an obstacles environment. Firstly, the utilization of the classic Apollonius circle theory as prior knowledge guides agent action selection, narrows the exploratory action space, and accelerates the learning of strategies. Subsequently, the variance descriptor restricts the motion direction of pursuers, thus ensuring that pursuers continuously narrow the encirclement until the prey is successfully encircled. Finally, experiments in an obstacles environment were conducted to validate the proposed method. The results indicate that our method can acquire an effective encirclement strategy, with an encirclement success rate exceeding that of previous methods by more than 10
更多查看译文
关键词
Multi-agent encirclement with collision avoidance,Apollonius circle,Prior knowledge,Variance descriptor,Multi-agent deep reinforcement learning
AI 理解论文
溯源树
样例
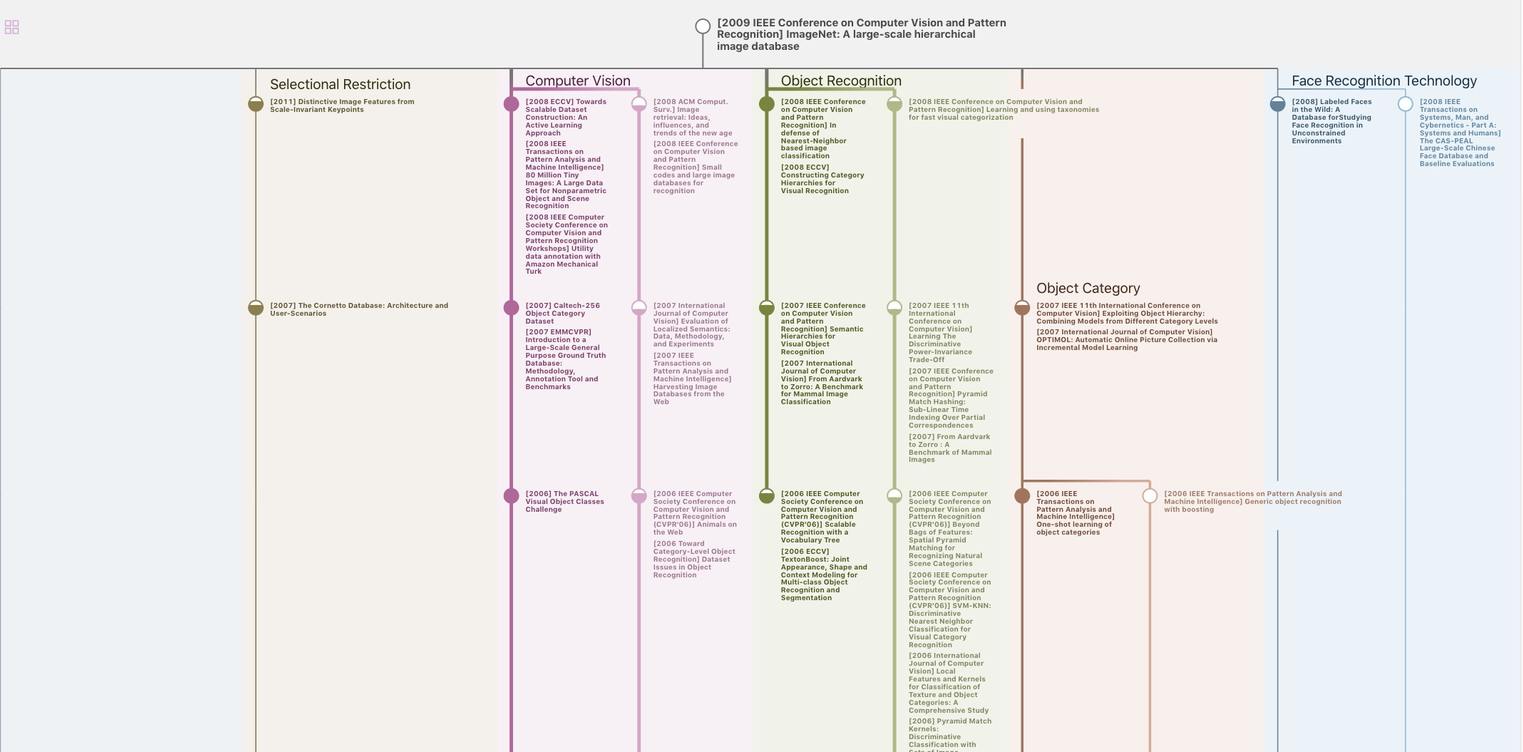
生成溯源树,研究论文发展脉络
数据免责声明
页面数据均来自互联网公开来源、合作出版商和通过AI技术自动分析结果,我们不对页面数据的有效性、准确性、正确性、可靠性、完整性和及时性做出任何承诺和保证。若有疑问,可以通过电子邮件方式联系我们:report@aminer.cn