Why In-Context Learning Transformers Are Tabular Data Classifiers
CoRR(2024)
摘要
The recently introduced TabPFN pretrains an In-Context Learning (ICL)transformer on synthetic data to perform tabular data classification. Assynthetic data does not share features or labels with real-world data, theunderlying mechanism that contributes to the success of this method remainsunclear. This study provides an explanation by demonstrating thatICL-transformers acquire the ability to create complex decision boundariesduring pretraining. To validate our claim, we develop a novel forest datasetgenerator which creates datasets that are unrealistic, but have complexdecision boundaries. Our experiments confirm the effectiveness ofICL-transformers pretrained on this data. Furthermore, we create TabForestPFN,the ICL-transformer pretrained on both the original TabPFN synthetic datasetgenerator and our forest dataset generator. By fine-tuning this model, we reachthe current state-of-the-art on tabular data classification. Code is availableat https://github.com/FelixdenBreejen/TabForestPFN.
更多查看译文
AI 理解论文
溯源树
样例
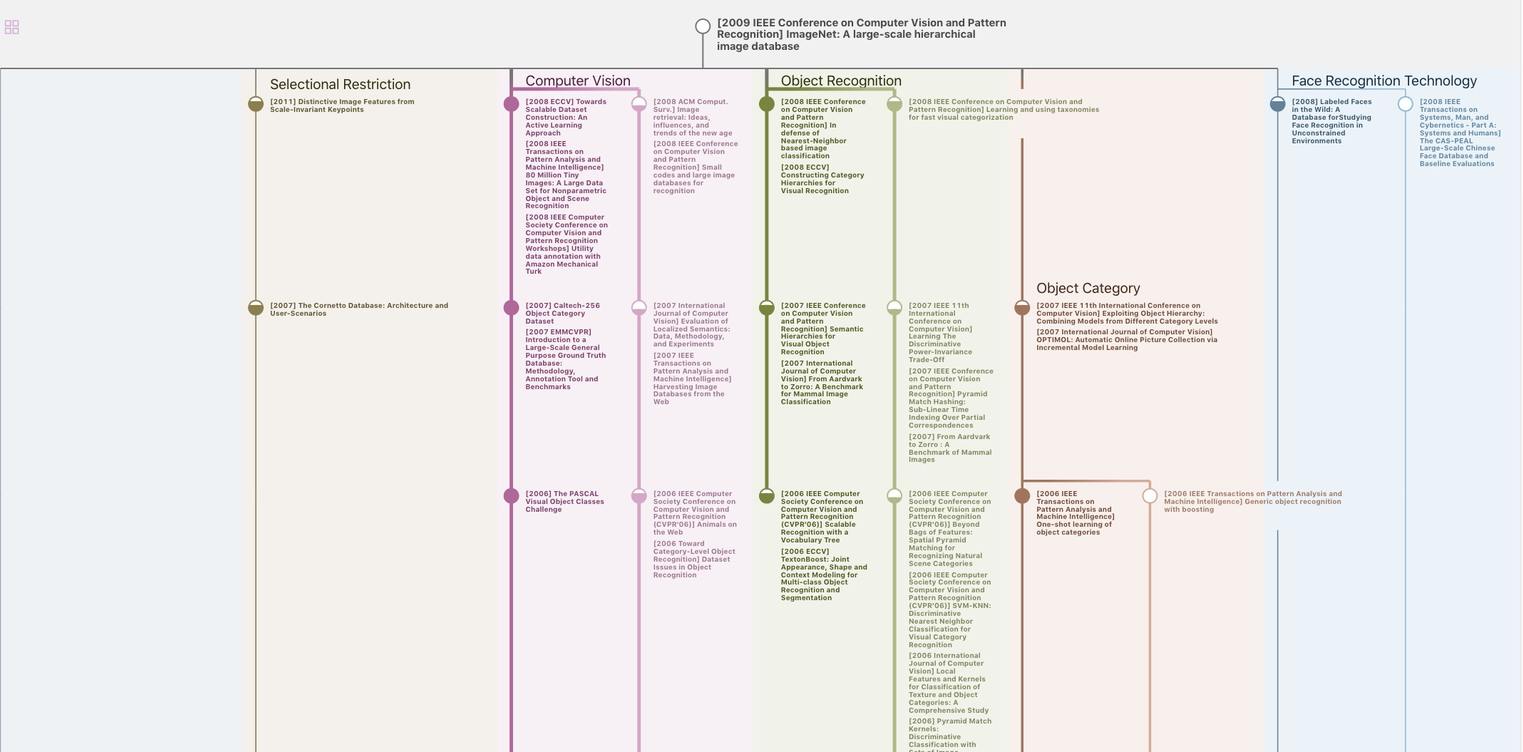
生成溯源树,研究论文发展脉络
数据免责声明
页面数据均来自互联网公开来源、合作出版商和通过AI技术自动分析结果,我们不对页面数据的有效性、准确性、正确性、可靠性、完整性和及时性做出任何承诺和保证。若有疑问,可以通过电子邮件方式联系我们:report@aminer.cn