Adaptive Rentention Correction for Continual Learning
CoRR(2024)
摘要
Continual learning, also known as lifelong learning or incremental learning,refers to the process by which a model learns from a stream of incoming dataover time. A common problem in continual learning is the classification layer'sbias towards the most recent task. Traditionally, methods have relied onincorporating data from past tasks during training to mitigate this issue.However, the recent shift in continual learning to memory-free environments hasrendered these approaches infeasible. In this study, we propose a solutionfocused on the testing phase. We first introduce a simple Out-of-Task Detectionmethod, OTD, designed to accurately identify samples from past tasks duringtesting. Leveraging OTD, we then propose: (1) an Adaptive Retention mechanismfor dynamically tuning the classifier layer on past task data; (2) an AdaptiveCorrection mechanism for revising predictions when the model classifies datafrom previous tasks into classes from the current task. We name our approachAdaptive Retention Correction (ARC). While designed for memory-freeenvironments, ARC also proves effective in memory-based settings. Extensiveexperiments show that our proposed method can be plugged in to virtually anyexisting continual learning approach without requiring any modifications to itstraining procedure. Specifically, when integrated with state-of-the-artapproaches, ARC achieves an average performance increase of 2.7the CIFAR-100 and Imagenet-R datasets, respectively.
更多查看译文
AI 理解论文
溯源树
样例
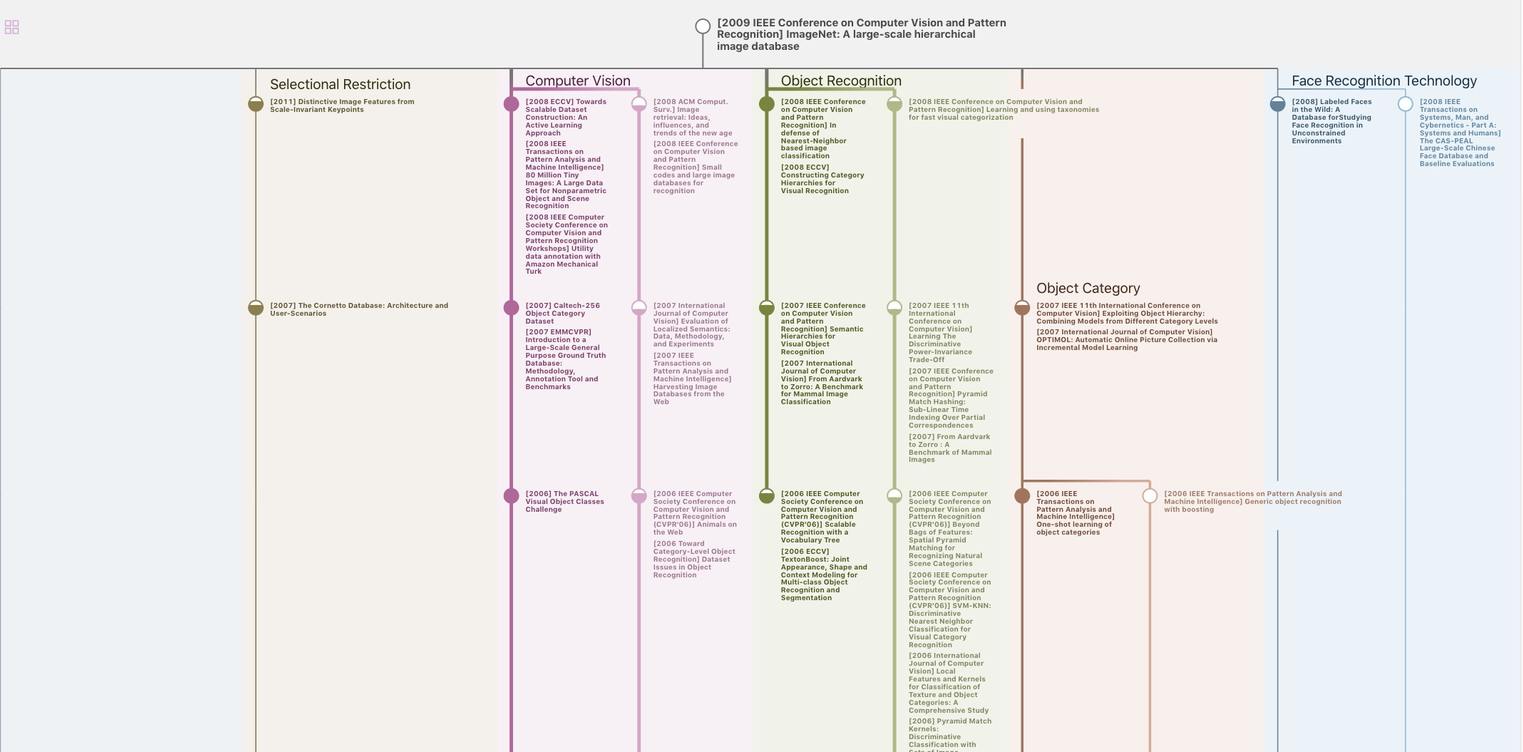
生成溯源树,研究论文发展脉络
数据免责声明
页面数据均来自互联网公开来源、合作出版商和通过AI技术自动分析结果,我们不对页面数据的有效性、准确性、正确性、可靠性、完整性和及时性做出任何承诺和保证。若有疑问,可以通过电子邮件方式联系我们:report@aminer.cn