Large Language Models can Deliver Accurate and Interpretable Time Series Anomaly Detection
CoRR(2024)
摘要
Time series anomaly detection (TSAD) plays a crucial role in various
industries by identifying atypical patterns that deviate from standard trends,
thereby maintaining system integrity and enabling prompt response measures.
Traditional TSAD models, which often rely on deep learning, require extensive
training data and operate as black boxes, lacking interpretability for detected
anomalies. To address these challenges, we propose LLMAD, a novel TSAD method
that employs Large Language Models (LLMs) to deliver accurate and interpretable
TSAD results. LLMAD innovatively applies LLMs for in-context anomaly detection
by retrieving both positive and negative similar time series segments,
significantly enhancing LLMs' effectiveness. Furthermore, LLMAD employs the
Anomaly Detection Chain-of-Thought (AnoCoT) approach to mimic expert logic for
its decision-making process. This method further enhances its performance and
enables LLMAD to provide explanations for their detections through versatile
perspectives, which are particularly important for user decision-making.
Experiments on three datasets indicate that our LLMAD achieves detection
performance comparable to state-of-the-art deep learning methods while offering
remarkable interpretability for detections. To the best of our knowledge, this
is the first work that directly employs LLMs for TSAD.
更多查看译文
AI 理解论文
溯源树
样例
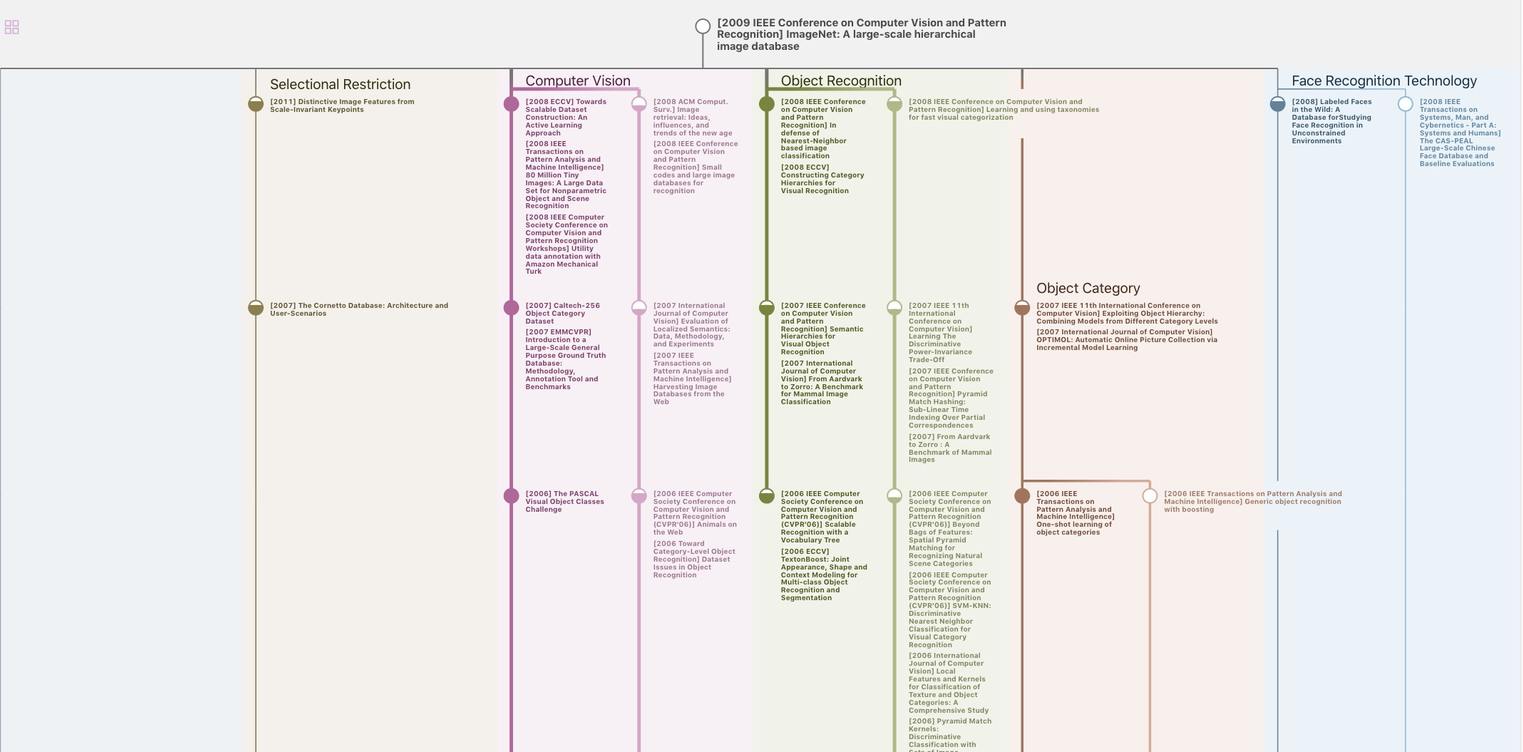
生成溯源树,研究论文发展脉络
数据免责声明
页面数据均来自互联网公开来源、合作出版商和通过AI技术自动分析结果,我们不对页面数据的有效性、准确性、正确性、可靠性、完整性和及时性做出任何承诺和保证。若有疑问,可以通过电子邮件方式联系我们:report@aminer.cn