Machine Learning Provides Insights for Spatially Explicit Pest Management Strategies by Integrating Information on Population Connectivity and Habitat Use in a Key Agricultural Pest.
PEST MANAGEMENT SCIENCE(2024)
摘要
BACKGROUNDInsect pests have garnered increasing interest because of anthropogenic global change, and their sustainable management requires knowledge of population habitat use and spread patterns. To enhance this knowledge for the prevalent tea pest Empoasca onukii, we utilized a random forest algorithm and a bivariate map to develop and integrate models of its habitat suitability and genetic connectivity across China.RESULTSOur modeling revealed heterogeneous spatial patterns in suitability and connectivity despite the common key environmental predictor of isothermality. Analyses indicated that tea cultivation in areas surrounding the Tibetan Plateau and the southern tip of China may be at low risk of population outbreaks because of their predicted low suitability and connectivity. However, regions along the middle and lower reaches of the Yangtze River should consider the high abundance and high recolonization potential of E. onukii, and thus the importance of control measures. Our results also emphasized the need to prevent dispersal from outside regions in the areas north of the Yangtze River and highlighted the effectiveness of internal management efforts in southwestern China and along the southeastern coast. Further projections under future conditions suggested the potential for increased abundance and spread in regions north of the Yangtze River and the southern tip of China, and indicated the importance of long-term monitoring efforts in these areas.CONCLUSIONThese findings highlighted the significance of combining information on habitat use and spread patterns for spatially explicit pest management planning. In addition, the approaches we used have potential applications in the management of other pest systems and the conservation of endangered biological resources. (c) 2024 Society of Chemical Industry. Diverse categories of regions that were expected to exhibit varying risks of Empoasca onukii population occurrence and spread were revealed using a random forest algorithm and a bivariate map. The results contribute to the formation of spatially explicit strategies for E. onukii management under current and future climate conditions. image
更多查看译文
关键词
pest management,gene flow,habitat use,random forest,spatial modeling
AI 理解论文
溯源树
样例
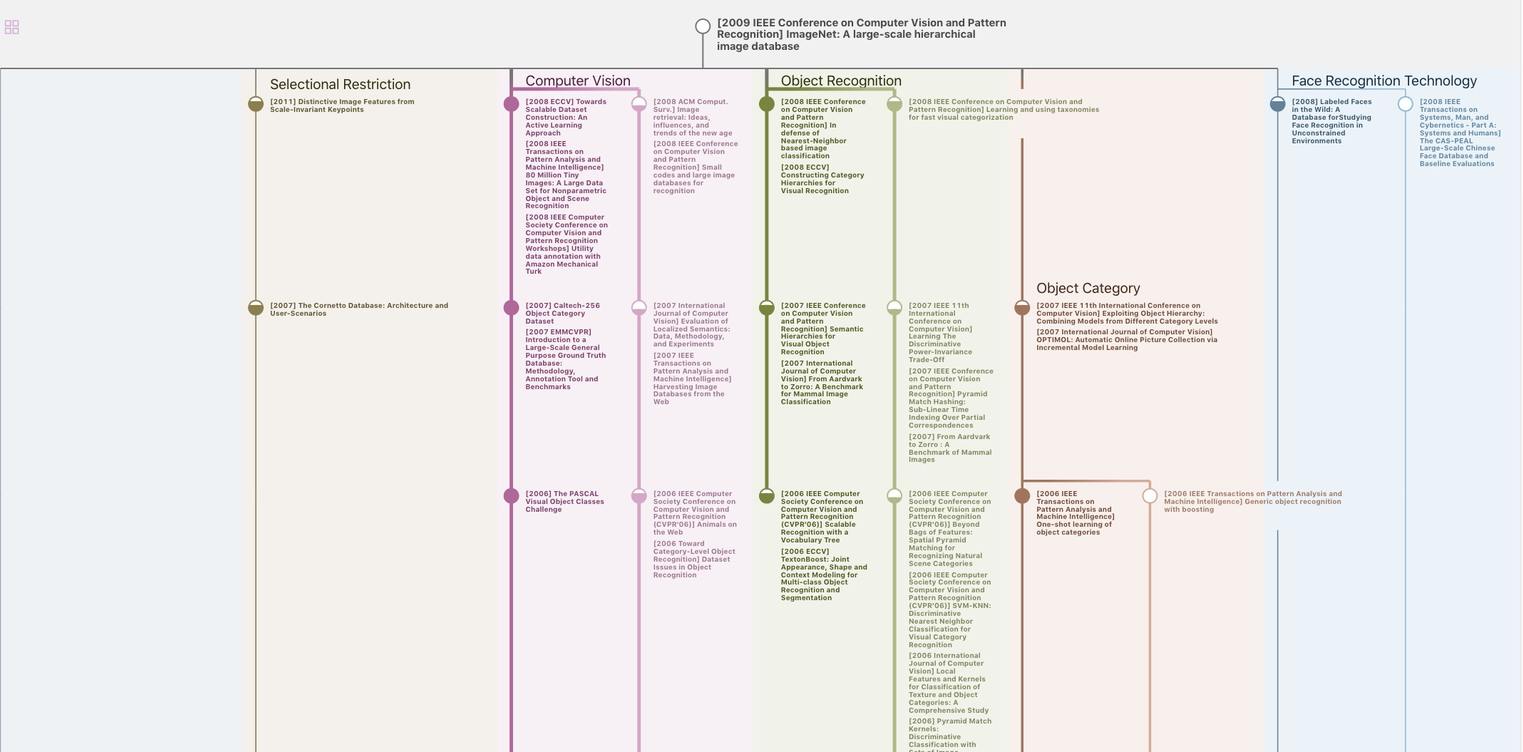
生成溯源树,研究论文发展脉络
数据免责声明
页面数据均来自互联网公开来源、合作出版商和通过AI技术自动分析结果,我们不对页面数据的有效性、准确性、正确性、可靠性、完整性和及时性做出任何承诺和保证。若有疑问,可以通过电子邮件方式联系我们:report@aminer.cn