Exploring the Design Space of Discontinuous Metal Matrix Composites Through Domain-Knowledge Enhanced Machine Learning
Extreme Mechanics Letters(2024)
摘要
Tailored reinforcement architectures in discontinuous metal matrix composites (DMMCs) offer superior mechanical performance with broad scientific and financial interests. This study presents a domain-knowledge enhanced machine learning approach to efficiently explore the design space of Al-SiC DMMCs for optimization. A substantial dataset containing 140,000 instances, resembling characteristic reinforcement configurations and variants, is generated using a series of algorithms. Employing high-throughput finite element analysis, the elastic properties of each configuration are estimated. Statistical analysis reveals that a more homogeneous distributed reinforcement contributes to mechanical stability, whereas configurations with extreme performance tend to have inhomogeneous reinforcement distribution. A deep residual neural network trained on this dataset accurately learns the structure-property correlations. Coupled with a genetic algorithm, the framework identifies optimal configurations across different volume fractions for maximizing/minimizing properties including tensile modulus, shear modulus, and Poisson's ratio. Comparative analysis shows the incorporation of domain knowledge improves data quality, facilitating more effective design space exploration. This study contributes to advancing composite materials design, particularly for next-generation high-performance DMMCs.
更多查看译文
关键词
Discontinuous metal matrix composites,Machine learning,Domain knowledge,Finite elements method,Optimization
AI 理解论文
溯源树
样例
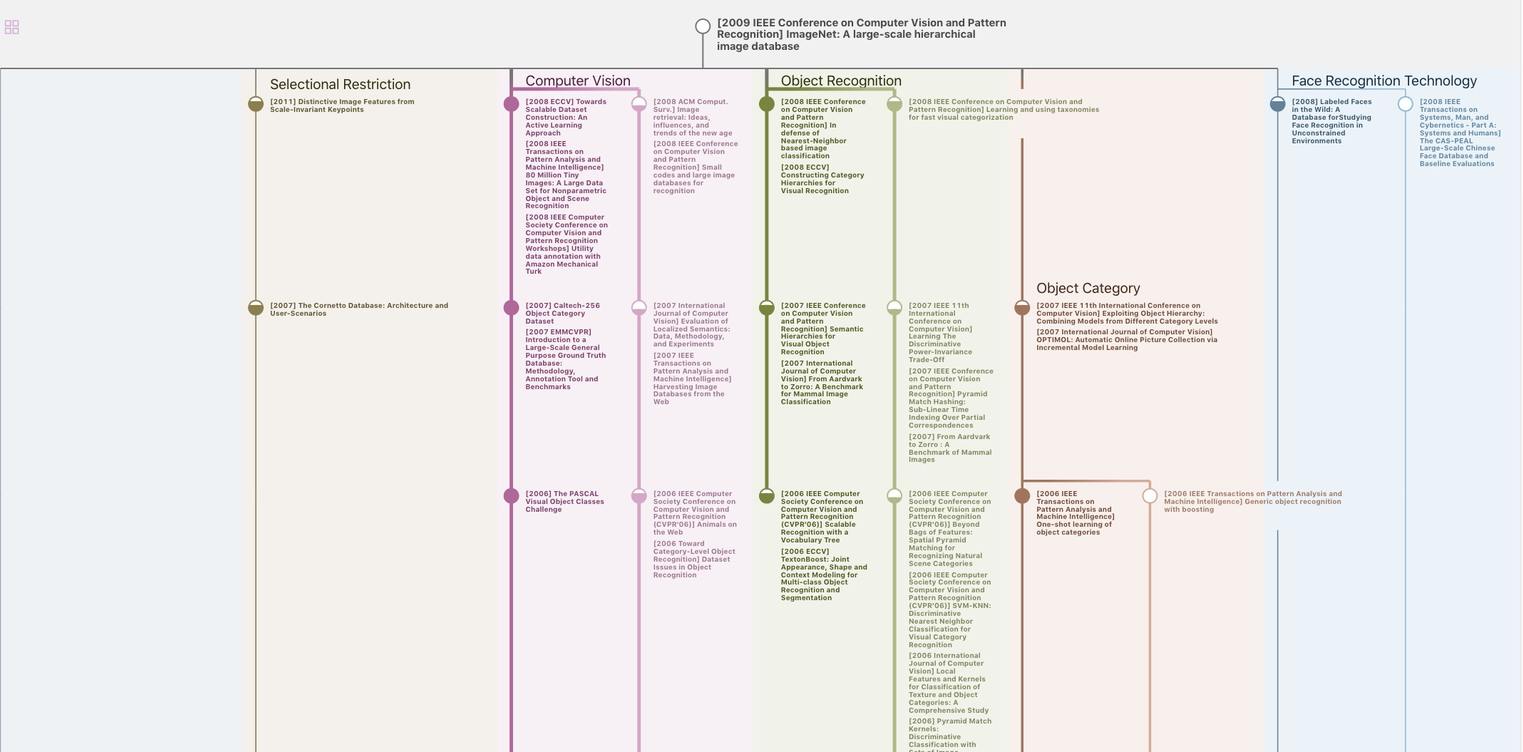
生成溯源树,研究论文发展脉络
数据免责声明
页面数据均来自互联网公开来源、合作出版商和通过AI技术自动分析结果,我们不对页面数据的有效性、准确性、正确性、可靠性、完整性和及时性做出任何承诺和保证。若有疑问,可以通过电子邮件方式联系我们:report@aminer.cn