FedDr+: Stabilizing Dot-regression with Global Feature Distillation for Federated Learning
CoRR(2024)
摘要
Federated Learning (FL) has emerged as a pivotal framework for the
development of effective global models (global FL) or personalized models
(personalized FL) across clients with heterogeneous, non-iid data distribution.
A key challenge in FL is client drift, where data heterogeneity impedes the
aggregation of scattered knowledge. Recent studies have tackled the client
drift issue by identifying significant divergence in the last classifier layer.
To mitigate this divergence, strategies such as freezing the classifier weights
and aligning the feature extractor accordingly have proven effective. Although
the local alignment between classifier and feature extractor has been studied
as a crucial factor in FL, we observe that it may lead the model to
overemphasize the observed classes within each client. Thus, our objectives are
twofold: (1) enhancing local alignment while (2) preserving the representation
of unseen class samples. This approach aims to effectively integrate knowledge
from individual clients, thereby improving performance for both global and
personalized FL. To achieve this, we introduce a novel algorithm named FedDr+,
which empowers local model alignment using dot-regression loss. FedDr+ freezes
the classifier as a simplex ETF to align the features and improves aggregated
global models by employing a feature distillation mechanism to retain
information about unseen/missing classes. Consequently, we provide empirical
evidence demonstrating that our algorithm surpasses existing methods that use a
frozen classifier to boost alignment across the diverse distribution.
更多查看译文
AI 理解论文
溯源树
样例
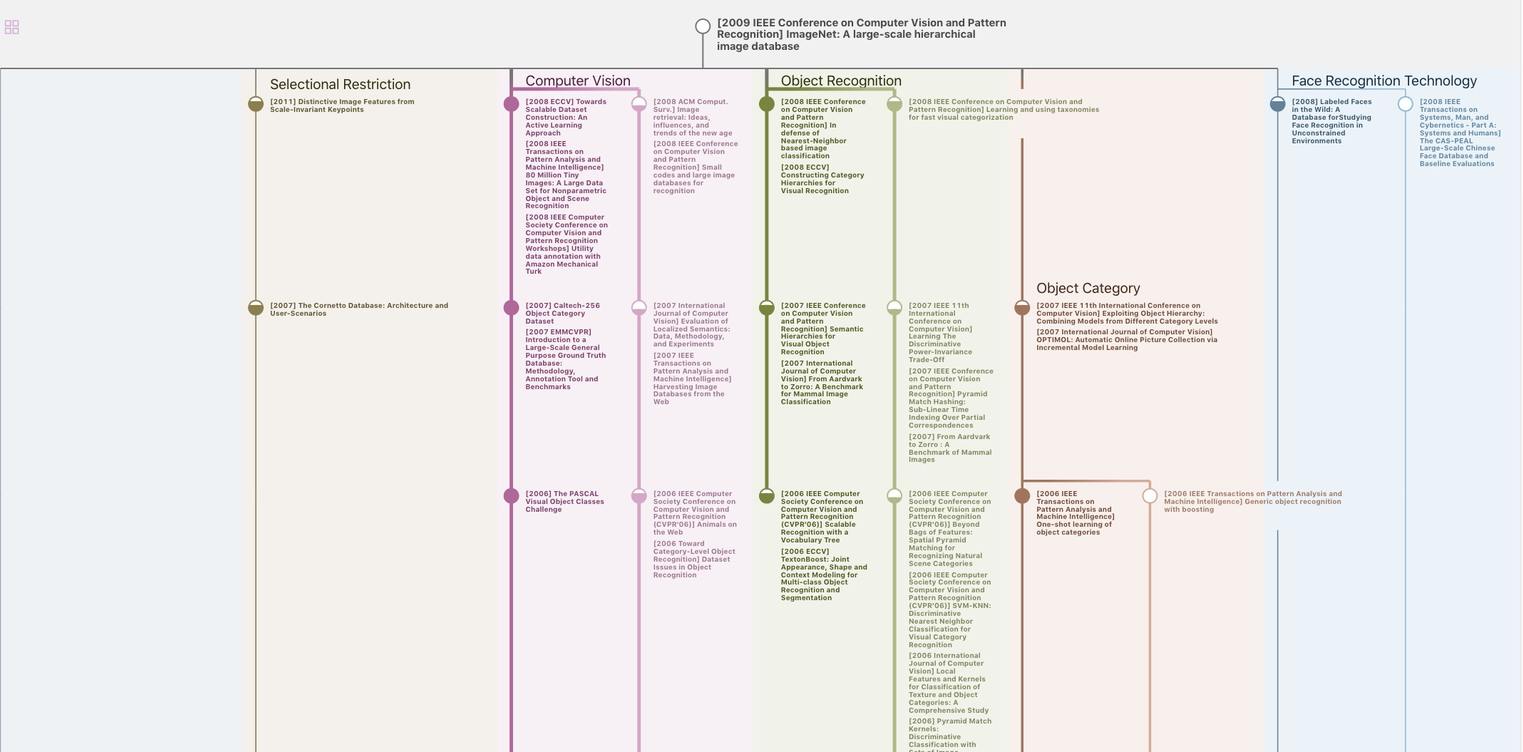
生成溯源树,研究论文发展脉络
数据免责声明
页面数据均来自互联网公开来源、合作出版商和通过AI技术自动分析结果,我们不对页面数据的有效性、准确性、正确性、可靠性、完整性和及时性做出任何承诺和保证。若有疑问,可以通过电子邮件方式联系我们:report@aminer.cn