AICoderEval: Improving AI Domain Code Generation of Large Language Models
CoRR(2024)
摘要
Automated code generation is a pivotal capability of large language models
(LLMs). However, assessing this capability in real-world scenarios remains
challenging. Previous methods focus more on low-level code generation, such as
model loading, instead of generating high-level codes catering for real-world
tasks, such as image-to-text, text classification, in various domains.
Therefore, we construct AICoderEval, a dataset focused on real-world tasks in
various domains based on HuggingFace, PyTorch, and TensorFlow, along with
comprehensive metrics for evaluation and enhancing LLMs' task-specific code
generation capability. AICoderEval contains test cases and complete programs
for automated evaluation of these tasks, covering domains such as natural
language processing, computer vision, and multimodal learning. To facilitate
research in this area, we open-source the AICoderEval dataset at
. After that, we
propose CoderGen, an agent-based framework, to help LLMs generate codes related
to real-world tasks on the constructed AICoderEval. Moreover, we train a more
powerful task-specific code generation model, named AICoder, which is refined
on llama-3 based on AICoderEval. Our experiments demonstrate the effectiveness
of CoderGen in improving LLMs' task-specific code generation capability (by
12.00% on pass@1 for original model and 9.50% on pass@1 for ReAct Agent).
AICoder also outperforms current code generation LLMs, indicating the great
quality of the AICoderEval benchmark.
更多查看译文
AI 理解论文
溯源树
样例
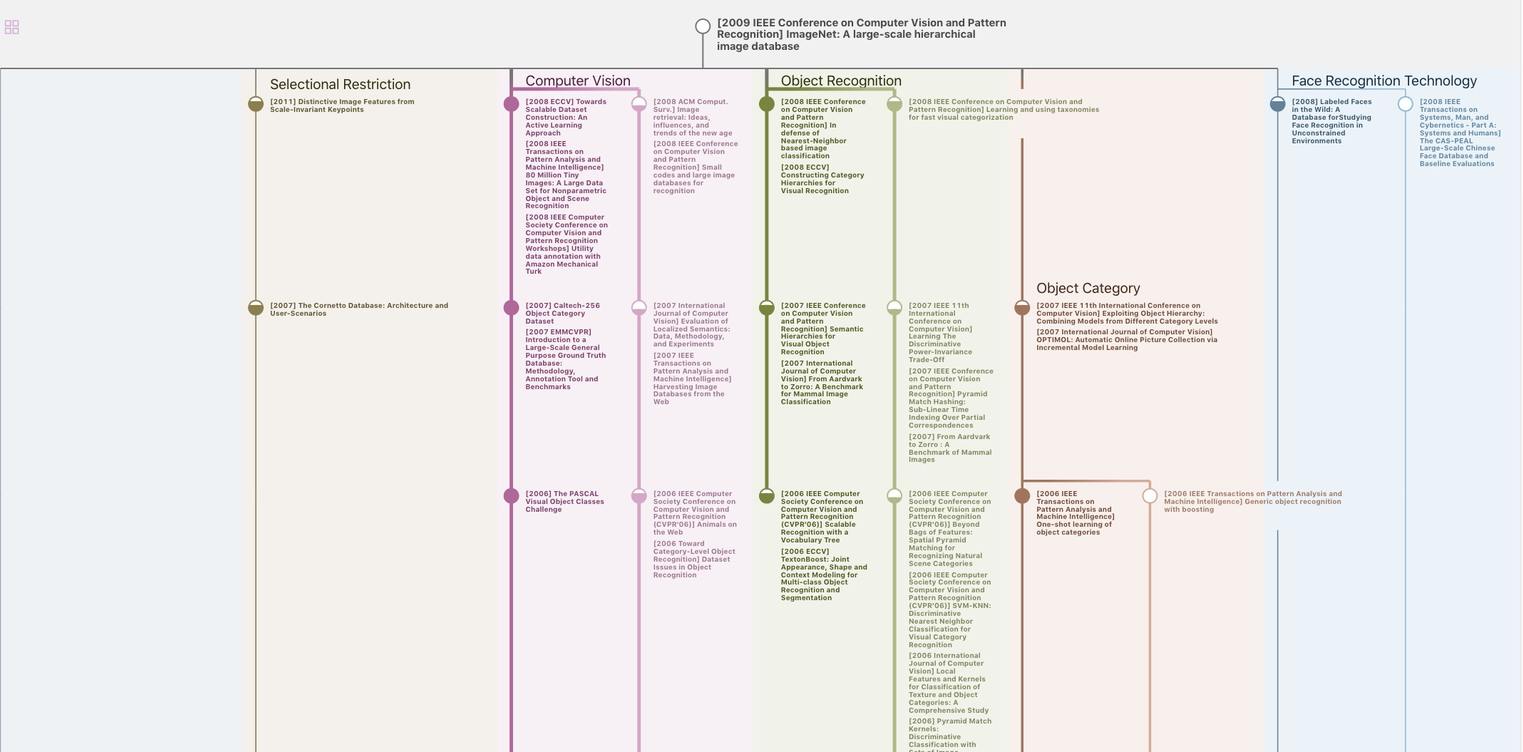
生成溯源树,研究论文发展脉络
数据免责声明
页面数据均来自互联网公开来源、合作出版商和通过AI技术自动分析结果,我们不对页面数据的有效性、准确性、正确性、可靠性、完整性和及时性做出任何承诺和保证。若有疑问,可以通过电子邮件方式联系我们:report@aminer.cn