CorDA: Context-Oriented Decomposition Adaptation of Large Language Models for Task-Aware Parameter-Efficient Fine-tuning
NeurIPS 2024(2024)
摘要
Current parameter-efficient fine-tuning (PEFT) methods build adapters widely agnostic of the context of downstream task to learn, or the context of important knowledge to maintain. As a result, there is often a performance gap compared to full-parameter fine-tuning, and meanwhile the fine-tuned model suffers from catastrophic forgetting of the pre-trained world knowledge. In this paper, we propose **CorDA**, a Context-oriented Decomposition Adaptation method that builds learnable **task-aware adapters** from weight decomposition oriented by the context of downstream task or the world knowledge to maintain. Concretely, we collect a few data samples, and perform singular value decomposition for each linear layer of a pre-trained LLM multiplied by the covariance matrix of the input activation using these samples. The inverse of the covariance matrix is multiplied with the decomposed components to reconstruct the original weights. By doing so, the context of the representative samples is captured through deciding the factorizing orientation. Our method enables two options, the **knowledge-preserved adaptation** and the **instruction-previewed adaptation**. For the former, we use question-answering samples to obtain the covariance matrices, and use the decomposed components with the smallest $r$ singular values to initialize a learnable adapter, with the others frozen such that the world knowledge is better preserved. For the latter, we use the instruction data from the fine-tuning task, such as math or coding, to orientate the decomposition and train the largest $r$ components that most correspond to the task to learn. We conduct extensive experiments on Math, Code, and Instruction Following tasks. Our knowledge-preserved adaptation not only achieves better performance than LoRA on fine-tuning tasks, but also mitigates the forgetting of world knowledge. Our instruction-previewed adaptation is able to further enhance the fine-tuning performance to be comparable with full fine-tuning, surpassing the state-of-the-art PEFT methods such as LoRA, DoRA, and PiSSA.
更多查看译文
关键词
parameter-efficient fine-tuning,LLM,knowledge forgetting
AI 理解论文
溯源树
样例
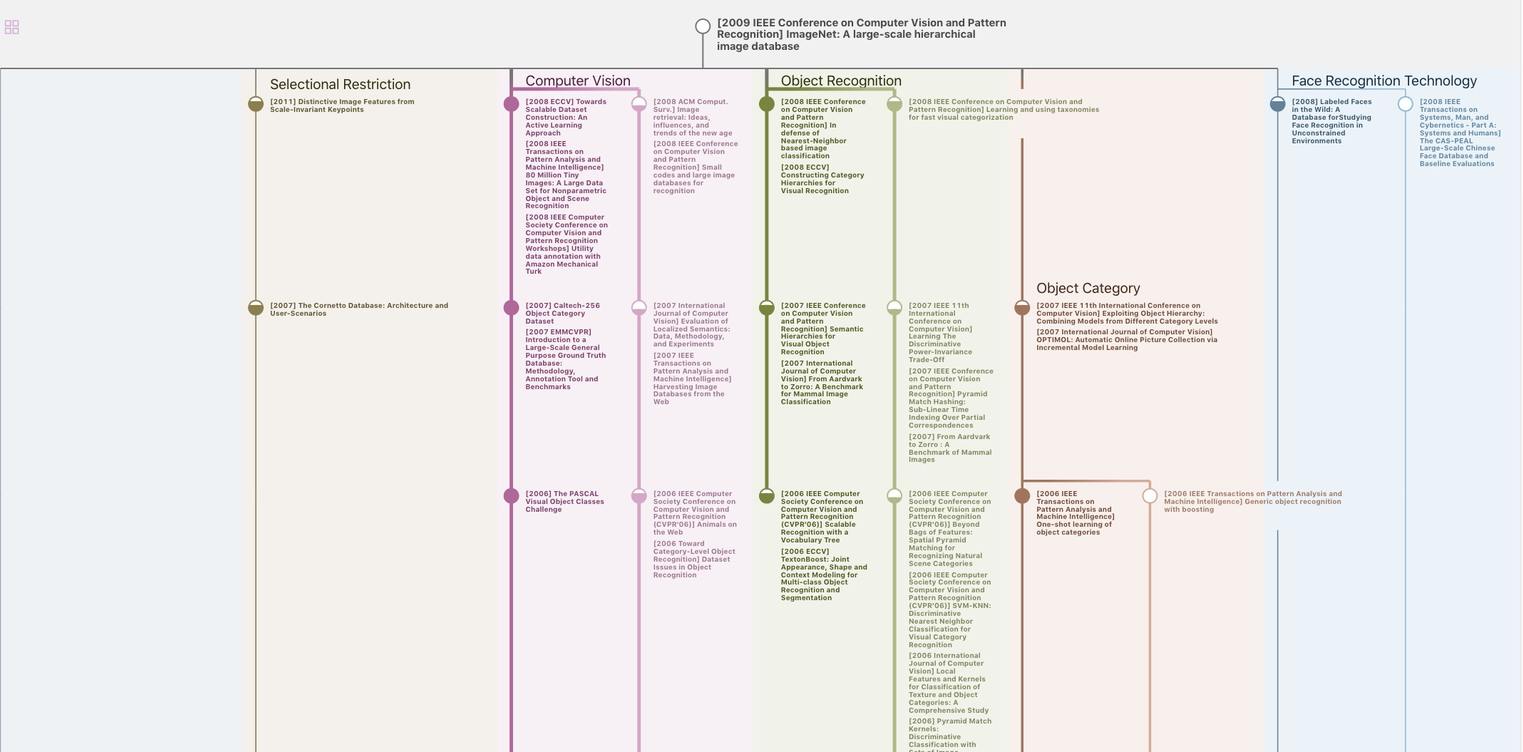
生成溯源树,研究论文发展脉络
数据免责声明
页面数据均来自互联网公开来源、合作出版商和通过AI技术自动分析结果,我们不对页面数据的有效性、准确性、正确性、可靠性、完整性和及时性做出任何承诺和保证。若有疑问,可以通过电子邮件方式联系我们:report@aminer.cn