II-Bench: an Image Implication Understanding Benchmark for Multimodal Large Language Models
arXiv (Cornell University)(2024)
摘要
The rapid advancements in the development of multimodal large language models(MLLMs) have consistently led to new breakthroughs on various benchmarks. Inresponse, numerous challenging and comprehensive benchmarks have been proposedto more accurately assess the capabilities of MLLMs. However, there is a dearthof exploration of the higher-order perceptual capabilities of MLLMs. To fillthis gap, we propose the Image Implication understanding Benchmark, II-Bench,which aims to evaluate the model's higher-order perception of images. Throughextensive experiments on II-Bench across multiple MLLMs, we have madesignificant findings. Initially, a substantial gap is observed between theperformance of MLLMs and humans on II-Bench. The pinnacle accuracy of MLLMsattains 74.898suggesting limitations in their ability to understand high-level semantics andcapture image details. Finally, it is observed that most models exhibitenhanced accuracy when image sentiment polarity hints are incorporated into theprompts. This observation underscores a notable deficiency in their inherentunderstanding of image sentiment. We believe that II-Bench will inspire thecommunity to develop the next generation of MLLMs, advancing the journeytowards expert artificial general intelligence (AGI). II-Bench is publiclyavailable at https://huggingface.co/datasets/m-a-p/II-Bench.
更多查看译文
AI 理解论文
溯源树
样例
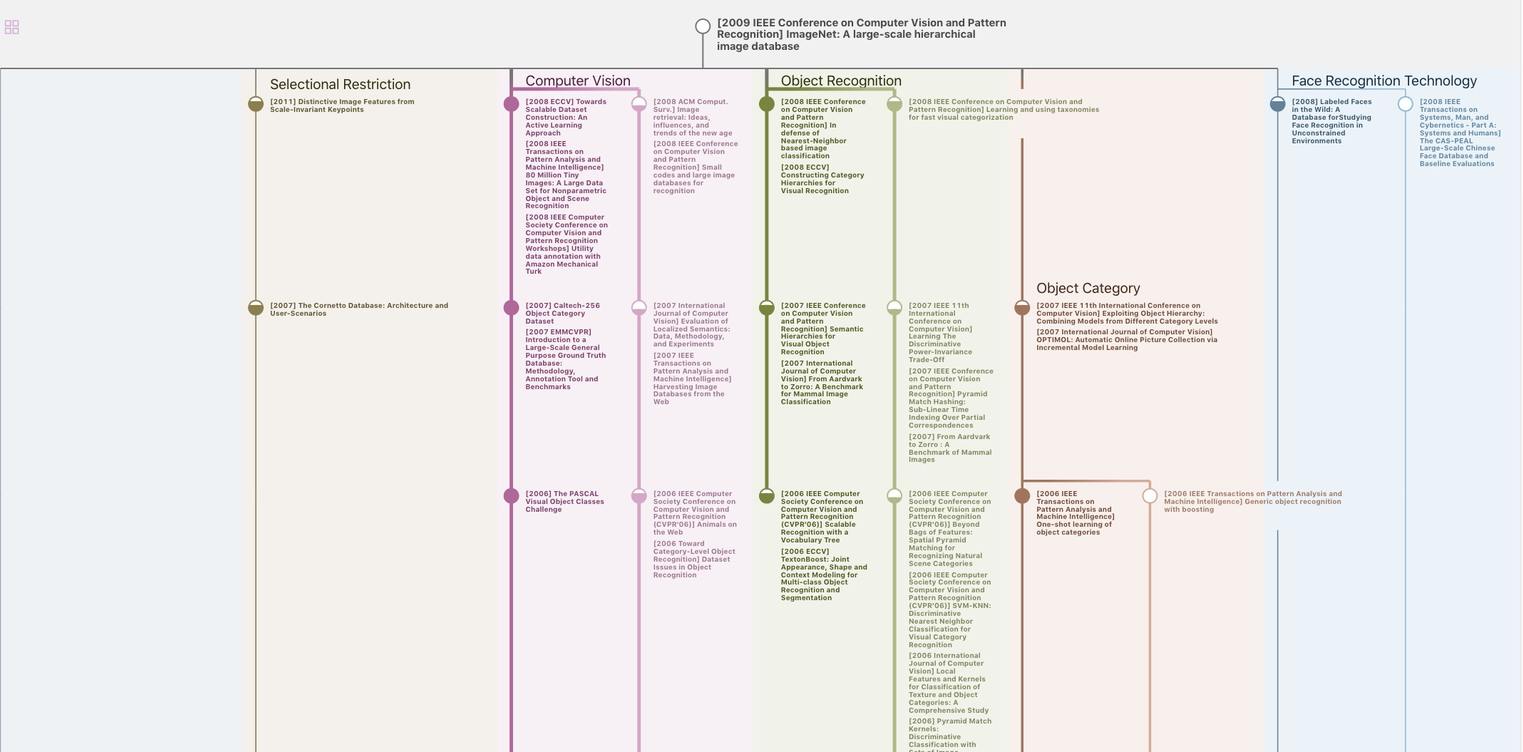
生成溯源树,研究论文发展脉络
数据免责声明
页面数据均来自互联网公开来源、合作出版商和通过AI技术自动分析结果,我们不对页面数据的有效性、准确性、正确性、可靠性、完整性和及时性做出任何承诺和保证。若有疑问,可以通过电子邮件方式联系我们:report@aminer.cn