Label Smoothing Improves Machine Unlearning
CoRR(2024)
摘要
The objective of machine unlearning (MU) is to eliminate previously learned
data from a model. However, it is challenging to strike a balance between
computation cost and performance when using existing MU techniques. Taking
inspiration from the influence of label smoothing on model confidence and
differential privacy, we propose a simple gradient-based MU approach that uses
an inverse process of label smoothing. This work introduces UGradSL, a simple,
plug-and-play MU approach that uses smoothed labels. We provide theoretical
analyses demonstrating why properly introducing label smoothing improves MU
performance. We conducted extensive experiments on six datasets of various
sizes and different modalities, demonstrating the effectiveness and robustness
of our proposed method. The consistent improvement in MU performance is only at
a marginal cost of additional computations. For instance, UGradSL improves over
the gradient ascent MU baseline by 66
unlearning efficiency.
更多查看译文
AI 理解论文
溯源树
样例
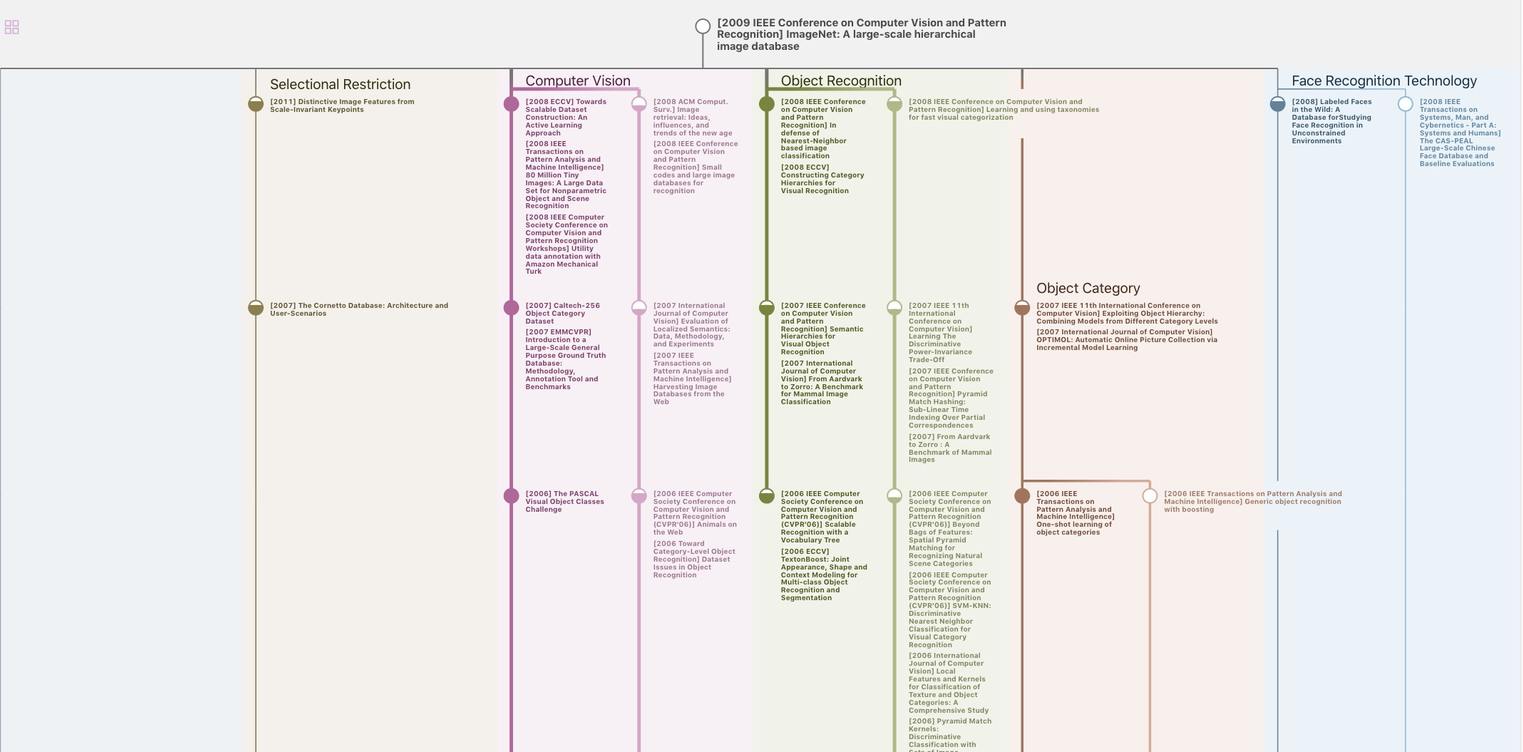
生成溯源树,研究论文发展脉络
数据免责声明
页面数据均来自互联网公开来源、合作出版商和通过AI技术自动分析结果,我们不对页面数据的有效性、准确性、正确性、可靠性、完整性和及时性做出任何承诺和保证。若有疑问,可以通过电子邮件方式联系我们:report@aminer.cn