What If We Recaption Billions of Web Images with LLaMA-3?
CoRR(2024)
摘要
Web-crawled image-text pairs are inherently noisy. Prior studies demonstrate
that semantically aligning and enriching textual descriptions of these pairs
can significantly enhance model training across various vision-language tasks,
particularly text-to-image generation. However, large-scale investigations in
this area remain predominantly closed-source. Our paper aims to bridge this
community effort, leveraging the powerful and open-sourced LLaMA-3, a
GPT-4 level LLM. Our recaptioning pipeline is simple: first, we fine-tune a
LLaMA-3-8B powered LLaVA-1.5 and then employ it to recaption 1.3 billion images
from the DataComp-1B dataset. Our empirical results confirm that this enhanced
dataset, Recap-DataComp-1B, offers substantial benefits in training advanced
vision-language models. For discriminative models like CLIP, we observe
enhanced zero-shot performance in cross-modal retrieval tasks. For generative
models like text-to-image Diffusion Transformers, the generated images exhibit
a significant improvement in alignment with users' text instructions,
especially in following complex queries. Our project page is
https://www.haqtu.me/Recap-Datacomp-1B/
更多查看译文
AI 理解论文
溯源树
样例
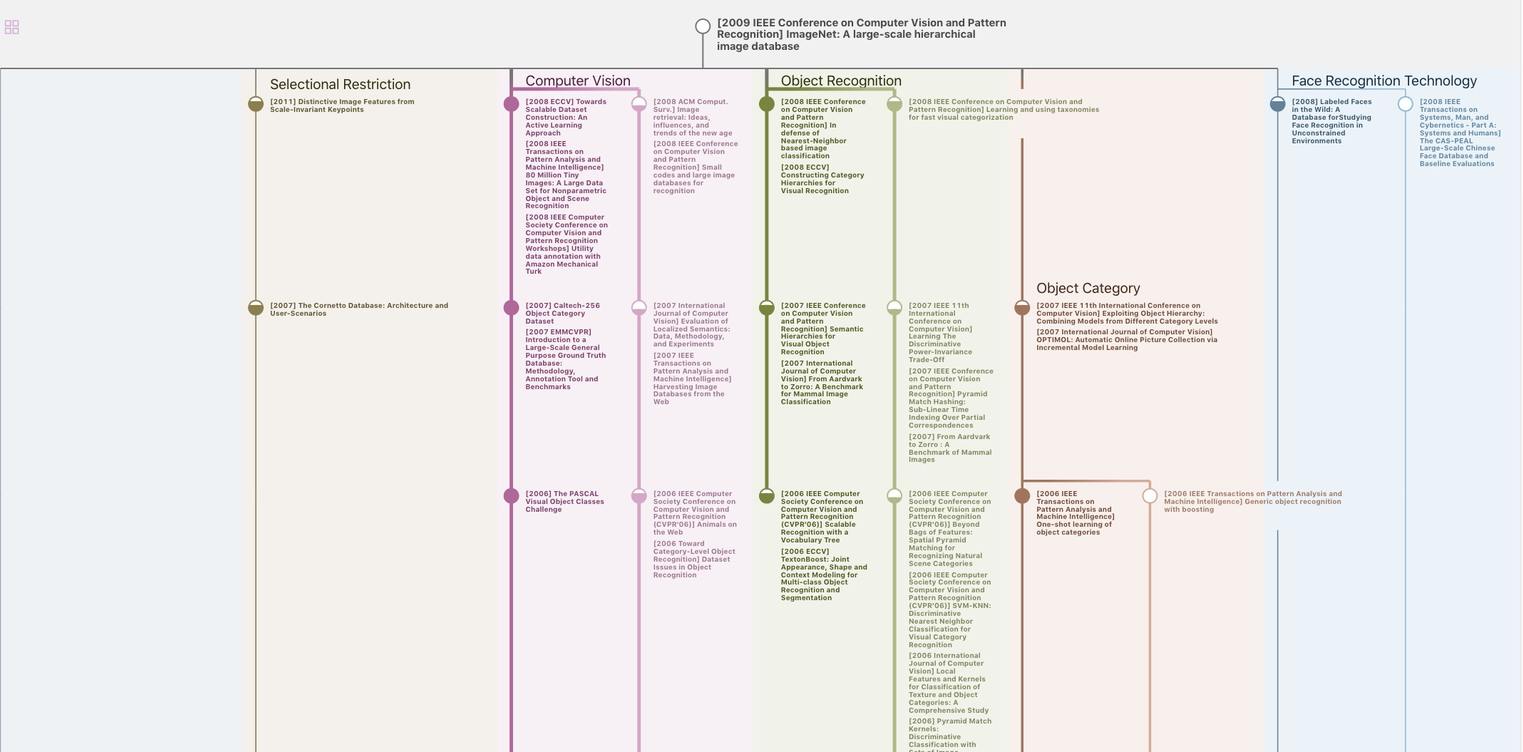
生成溯源树,研究论文发展脉络
数据免责声明
页面数据均来自互联网公开来源、合作出版商和通过AI技术自动分析结果,我们不对页面数据的有效性、准确性、正确性、可靠性、完整性和及时性做出任何承诺和保证。若有疑问,可以通过电子邮件方式联系我们:report@aminer.cn