ScaLES: Scalable Latent Exploration Score for Pre-Trained Generative Networks
CoRR(2024)
摘要
We develop Scalable Latent Exploration Score (ScaLES) to mitigate
over-exploration in Latent Space Optimization (LSO), a popular method for
solving black-box discrete optimization problems. LSO utilizes continuous
optimization within the latent space of a Variational Autoencoder (VAE) and is
known to be susceptible to over-exploration, which manifests in unrealistic
solutions that reduce its practicality. ScaLES is an exact and theoretically
motivated method leveraging the trained decoder's approximation of the data
distribution. ScaLES can be calculated with any existing decoder, e.g. from a
VAE, without additional training, architectural changes, or access to the
training data. Our evaluation across five LSO benchmark tasks and three VAE
architectures demonstrates that ScaLES enhances the quality of the solutions
while maintaining high objective values, leading to improvements over existing
solutions. We believe that new avenues to LSO will be opened by ScaLES ability
to identify out of distribution areas, differentiability, and computational
tractability. Open source code for ScaLES is available at
https://github.com/OmerRonen/scales.
更多查看译文
AI 理解论文
溯源树
样例
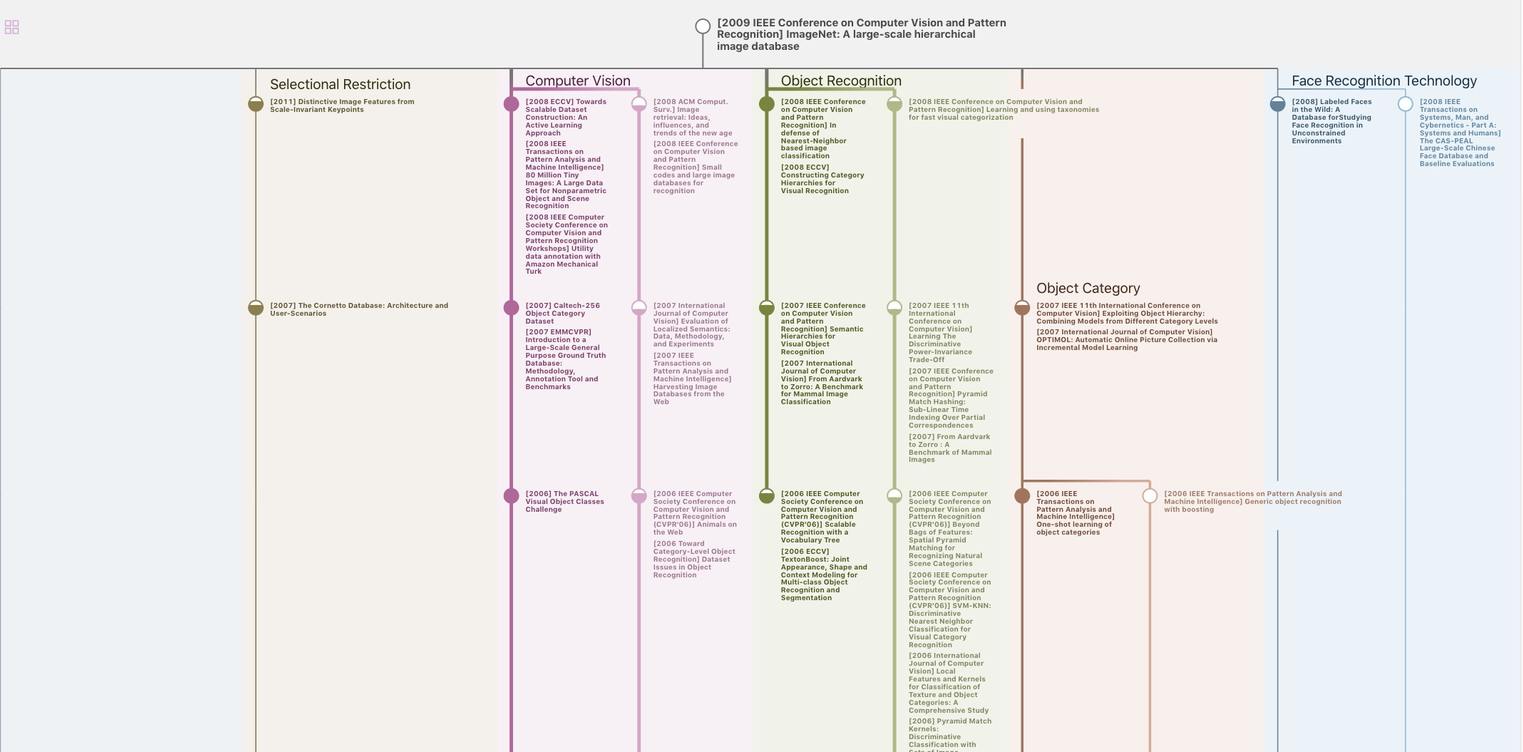
生成溯源树,研究论文发展脉络
数据免责声明
页面数据均来自互联网公开来源、合作出版商和通过AI技术自动分析结果,我们不对页面数据的有效性、准确性、正确性、可靠性、完整性和及时性做出任何承诺和保证。若有疑问,可以通过电子邮件方式联系我们:report@aminer.cn