DIEKAE: Difference Injection for Efficient Knowledge Augmentation and Editing of Large Language Models
CoRR(2024)
摘要
Pretrained Language Models (PLMs) store extensive knowledge within their
weights, enabling them to recall vast amount of information. However, relying
on this parametric knowledge brings some limitations such as outdated
information or gaps in the training data. This work addresses these problems by
distinguish between two separate solutions: knowledge editing and knowledge
augmentation. We introduce Difference Injection for Efficient Knowledge
Augmentation and Editing (DIEKÆ), a new method that decouples knowledge
processing from the PLM (LLaMA2-7B, in particular) by adopting a series of
encoders. These encoders handle external knowledge and inject it into the PLM
layers, significantly reducing computational costs and improving performance of
the PLM. We propose a novel training technique for these encoders that does not
require back-propagation through the PLM, thus greatly reducing the memory and
time required to train them. Our findings demonstrate how our method is faster
and more efficient compared to multiple baselines in knowledge augmentation and
editing during both training and inference. We have released our code and data
at https://github.com/alessioGalatolo/DIEKAE.
更多查看译文
AI 理解论文
溯源树
样例
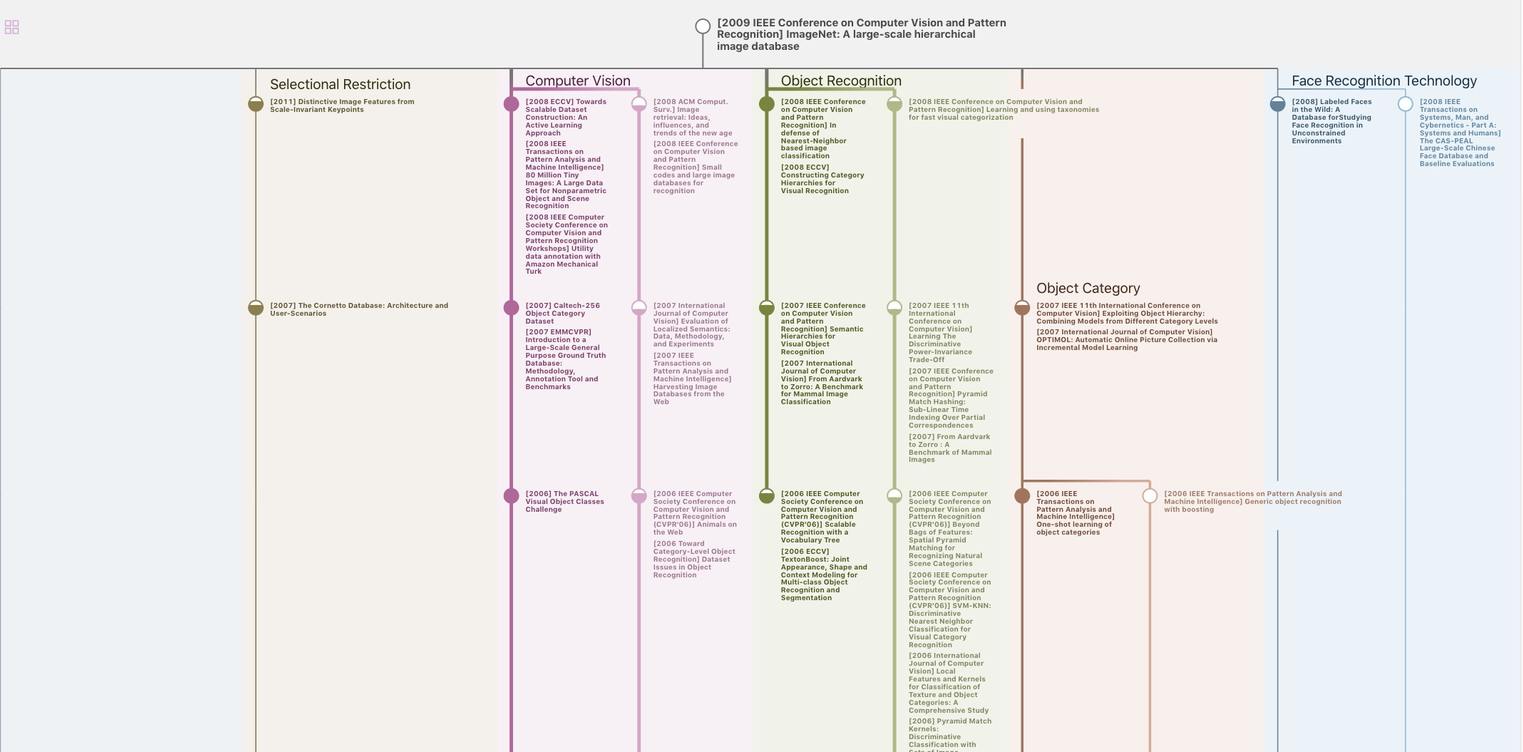
生成溯源树,研究论文发展脉络
数据免责声明
页面数据均来自互联网公开来源、合作出版商和通过AI技术自动分析结果,我们不对页面数据的有效性、准确性、正确性、可靠性、完整性和及时性做出任何承诺和保证。若有疑问,可以通过电子邮件方式联系我们:report@aminer.cn