Occam's Razor for Self Supervised Learning: What is Sufficient to Learn Good Representations?
CoRR(2024)
摘要
Deep Learning is often depicted as a trio of data-architecture-loss. Yet,
recent Self Supervised Learning (SSL) solutions have introduced numerous
additional design choices, e.g., a projector network, positive views, or
teacher-student networks. These additions pose two challenges. First, they
limit the impact of theoretical studies that often fail to incorporate all
those intertwined designs. Second, they slow-down the deployment of SSL methods
to new domains as numerous hyper-parameters need to be carefully tuned. In this
study, we bring forward the surprising observation that–at least for
pretraining datasets of up to a few hundred thousands samples–the additional
designs introduced by SSL do not contribute to the quality of the learned
representations. That finding not only provides legitimacy to existing
theoretical studies, but also simplifies the practitioner's path to SSL
deployment in numerous small and medium scale settings. Our finding answers a
long-lasting question: the often-experienced sensitivity to training settings
and hyper-parameters encountered in SSL come from their design, rather than the
absence of supervised guidance.
更多查看译文
AI 理解论文
溯源树
样例
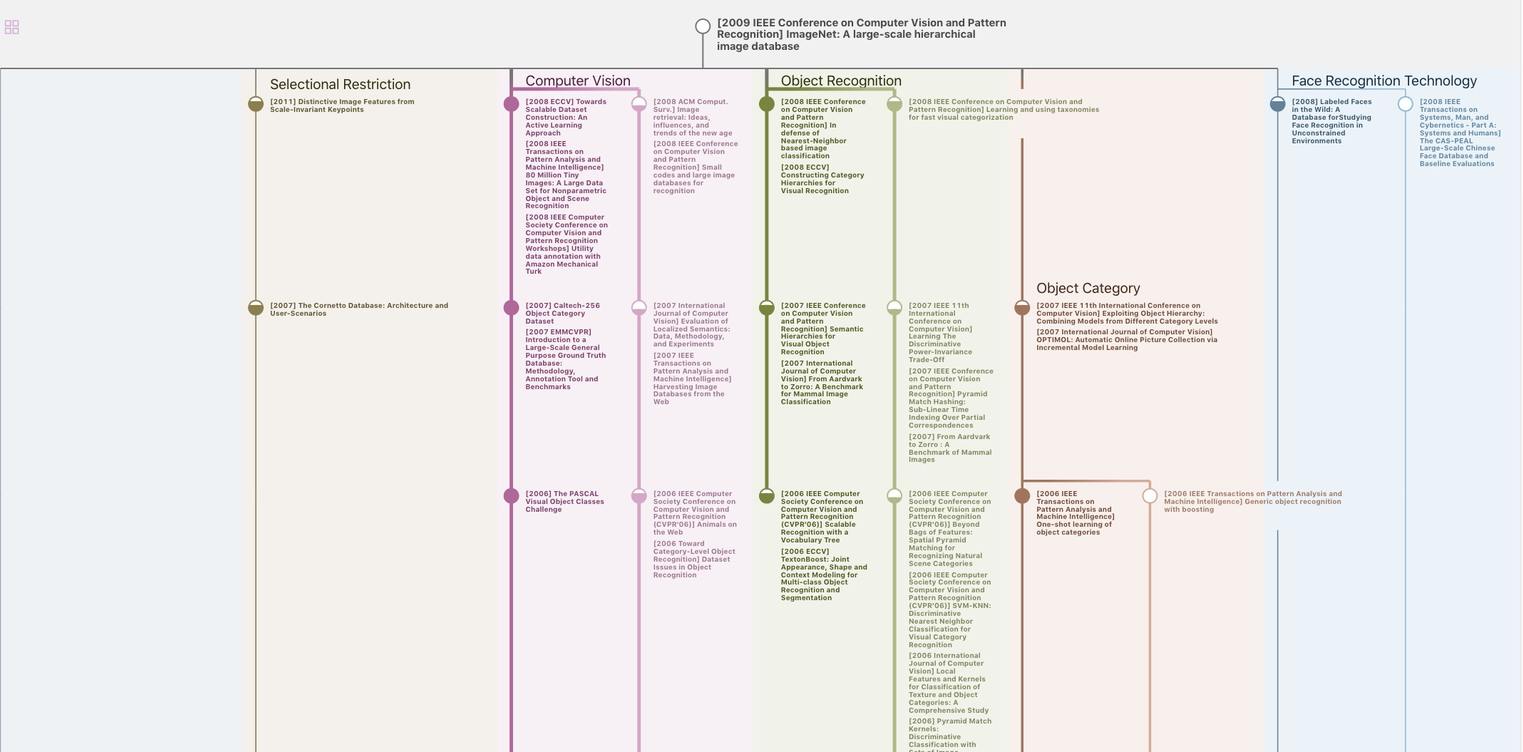
生成溯源树,研究论文发展脉络
数据免责声明
页面数据均来自互联网公开来源、合作出版商和通过AI技术自动分析结果,我们不对页面数据的有效性、准确性、正确性、可靠性、完整性和及时性做出任何承诺和保证。若有疑问,可以通过电子邮件方式联系我们:report@aminer.cn