Towards Lifelong Dialogue Agents Via Relation-aware Memory Construction and Timeline-augmented Response Generation
arxiv(2024)
摘要
To achieve lifelong human-agent interaction, dialogue agents need to constantly memorize perceived information and properly retrieve it for response generation (RG). While prior work focuses on getting rid of outdated memories to improve retrieval quality, we argue that such memories provide rich, important contextual cues for RG (e.g., changes in user behaviors) in long-term conversations. We present Theanine, a framework for LLM-based lifelong dialogue agents. Theanine discards memory removal and manages large-scale memories by linking them based on their temporal and cause-effect relation. Enabled by this linking structure, Theanine augments RG with memory timelines - series of memories representing the evolution or causality of relevant past events. Along with Theanine, we introduce TeaFarm, a counterfactual-driven evaluation scheme, addressing the limitation of G-Eval and human efforts in measuring memory-augmented dialogue agents. A supplementary video for Theanine and data for TeaFarm are at https://huggingface.co/spaces/ResearcherScholar/Theanine.
更多查看译文
AI 理解论文
溯源树
样例
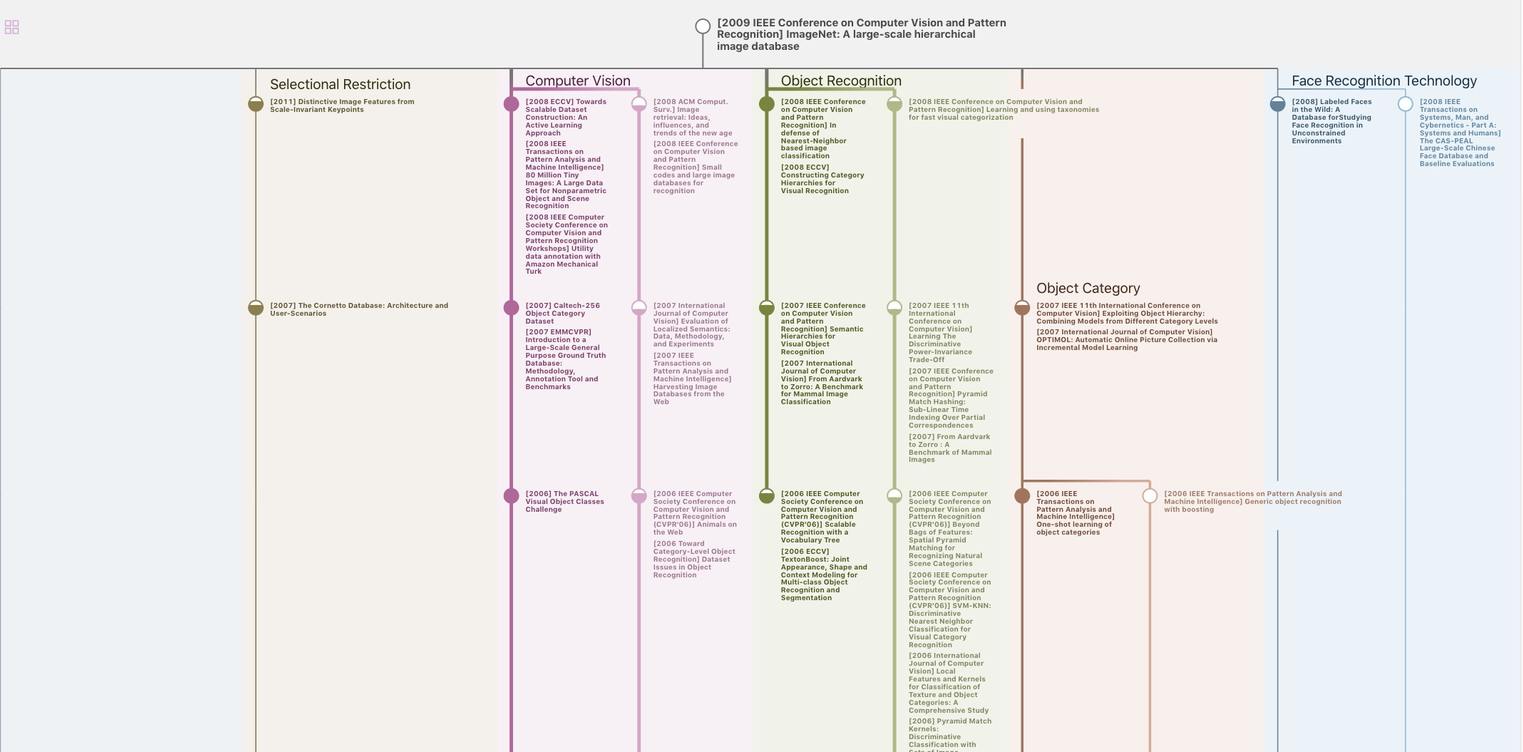
生成溯源树,研究论文发展脉络
数据免责声明
页面数据均来自互联网公开来源、合作出版商和通过AI技术自动分析结果,我们不对页面数据的有效性、准确性、正确性、可靠性、完整性和及时性做出任何承诺和保证。若有疑问,可以通过电子邮件方式联系我们:report@aminer.cn