Long-time asymptotics of noisy SVGD outside the population limit
CoRR(2024)
摘要
Stein Variational Gradient Descent (SVGD) is a widely used sampling algorithm
that has been successfully applied in several areas of Machine Learning. SVGD
operates by iteratively moving a set of interacting particles (which represent
the samples) to approximate the target distribution. Despite recent studies on
the complexity of SVGD and its variants, their long-time asymptotic behavior
(i.e., after numerous iterations ) is still not understood in the finite number
of particles regime. We study the long-time asymptotic behavior of a noisy
variant of SVGD. First, we establish that the limit set of noisy SVGD for large
is well-defined. We then characterize this limit set, showing that it
approaches the target distribution as increases. In particular, noisy SVGD
provably avoids the variance collapse observed for SVGD. Our approach involves
demonstrating that the trajectories of noisy SVGD closely resemble those
described by a McKean-Vlasov process.
更多查看译文
AI 理解论文
溯源树
样例
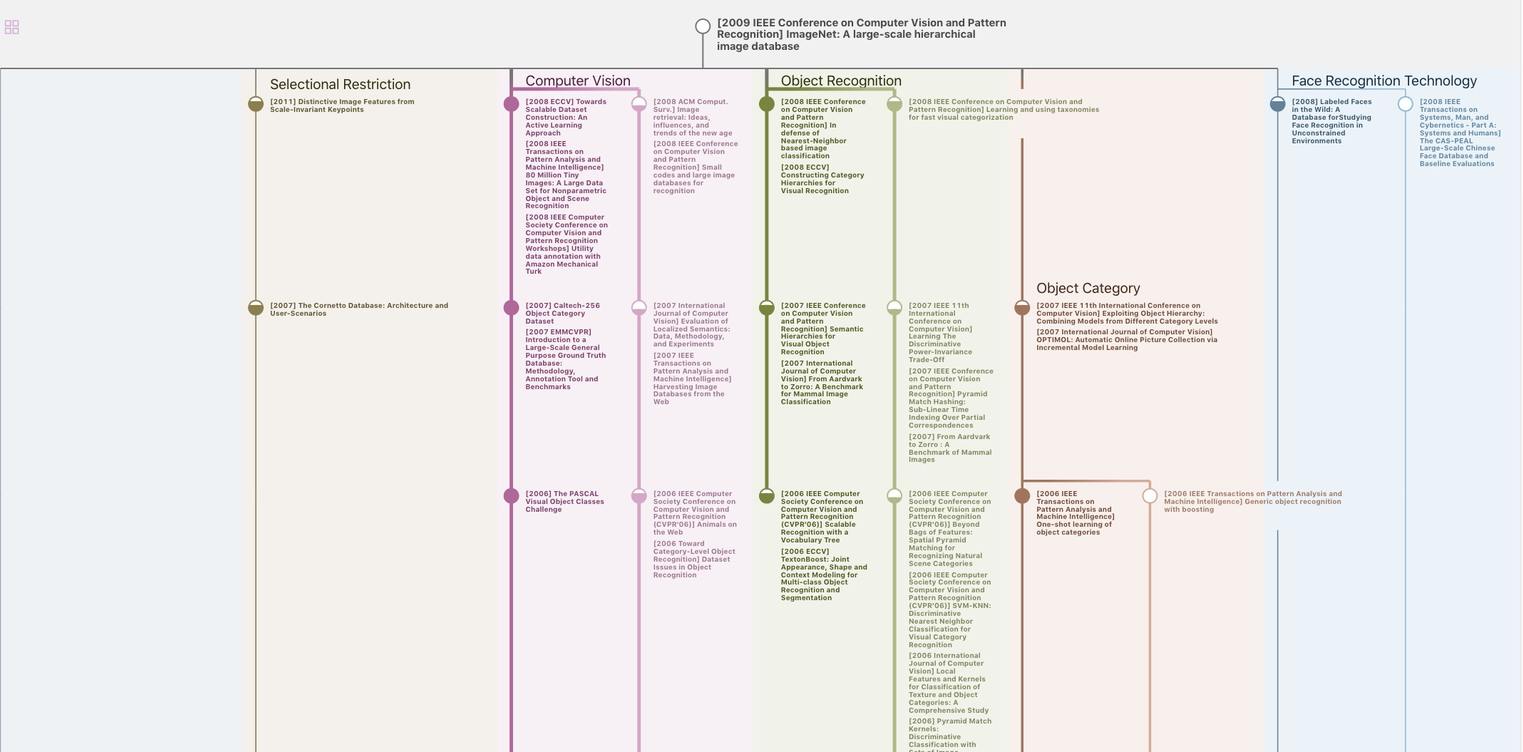
生成溯源树,研究论文发展脉络
数据免责声明
页面数据均来自互联网公开来源、合作出版商和通过AI技术自动分析结果,我们不对页面数据的有效性、准确性、正确性、可靠性、完整性和及时性做出任何承诺和保证。若有疑问,可以通过电子邮件方式联系我们:report@aminer.cn