Multi-Dimensional Pruning: Joint Channel, Layer and Block Pruning with Latency Constraint
CoRR(2024)
摘要
As we push the boundaries of performance in various vision tasks, the models
grow in size correspondingly. To keep up with this growth, we need very
aggressive pruning techniques for efficient inference and deployment on edge
devices. Existing pruning approaches are limited to channel pruning and
struggle with aggressive parameter reductions. In this paper, we propose a
novel multi-dimensional pruning framework that jointly optimizes pruning across
channels, layers, and blocks while adhering to latency constraints. We develop
a latency modeling technique that accurately captures model-wide latency
variations during pruning, which is crucial for achieving an optimal
latency-accuracy trade-offs at high pruning ratio. We reformulate pruning as a
Mixed-Integer Nonlinear Program (MINLP) to efficiently determine the optimal
pruned structure with only a single pass. Our extensive results demonstrate
substantial improvements over previous methods, particularly at large pruning
ratios. In classification, our method significantly outperforms prior art HALP
with a Top-1 accuracy of 70.0(v.s. 68.6) and an FPS of 5262 im/s(v.s. 4101
im/s). In 3D object detection, we establish a new state-of-the-art by pruning
StreamPETR at a 45
(0.451 vs. 0.449) than the dense baseline.
更多查看译文
AI 理解论文
溯源树
样例
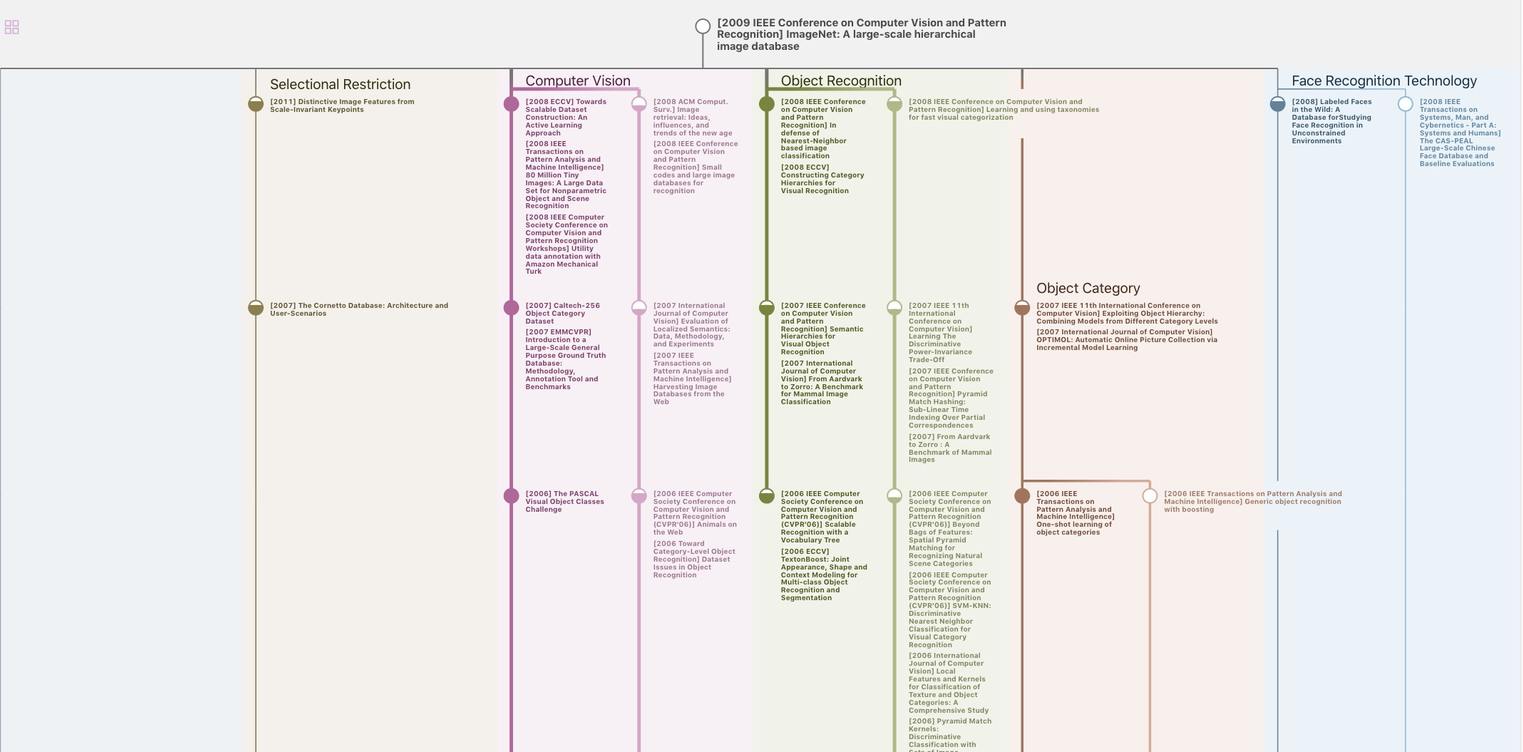
生成溯源树,研究论文发展脉络
数据免责声明
页面数据均来自互联网公开来源、合作出版商和通过AI技术自动分析结果,我们不对页面数据的有效性、准确性、正确性、可靠性、完整性和及时性做出任何承诺和保证。若有疑问,可以通过电子邮件方式联系我们:report@aminer.cn