Retrieval Meets Reasoning: Dynamic In-Context Editing for Long-Text Understanding
CoRR(2024)
摘要
Current Large Language Models (LLMs) face inherent limitations due to their
pre-defined context lengths, which impede their capacity for multi-hop
reasoning within extensive textual contexts. While existing techniques like
Retrieval-Augmented Generation (RAG) have attempted to bridge this gap by
sourcing external information, they fall short when direct answers are not
readily available. We introduce a novel approach that re-imagines information
retrieval through dynamic in-context editing, inspired by recent breakthroughs
in knowledge editing. By treating lengthy contexts as malleable external
knowledge, our method interactively gathers and integrates relevant
information, thereby enabling LLMs to perform sophisticated reasoning steps.
Experimental results demonstrate that our method effectively empowers
context-limited LLMs, such as Llama2, to engage in multi-hop reasoning with
improved performance, which outperforms state-of-the-art context window
extrapolation methods and even compares favorably to more advanced commercial
long-context models. Our interactive method not only enhances reasoning
capabilities but also mitigates the associated training and computational
costs, making it a pragmatic solution for enhancing LLMs' reasoning within
expansive contexts.
更多查看译文
AI 理解论文
溯源树
样例
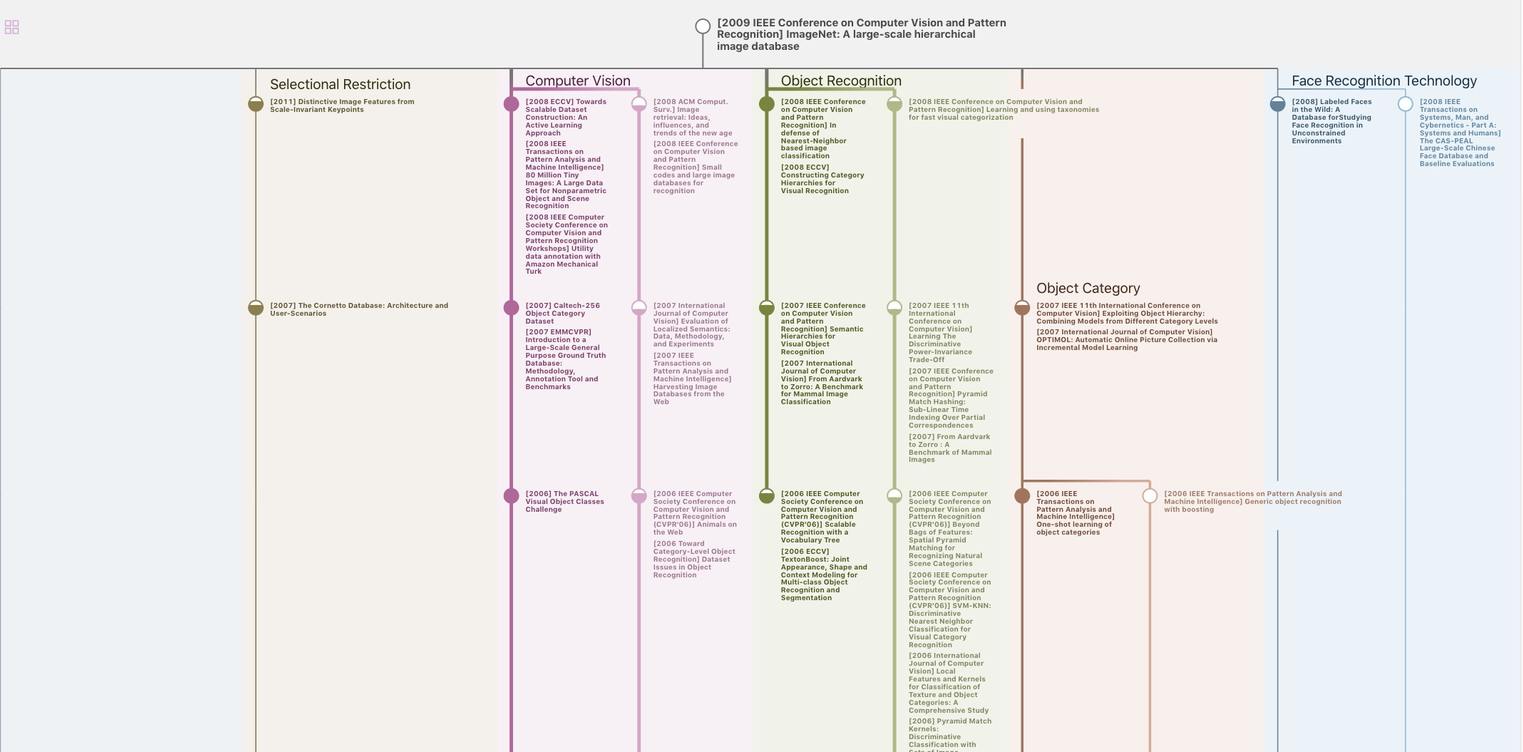
生成溯源树,研究论文发展脉络
数据免责声明
页面数据均来自互联网公开来源、合作出版商和通过AI技术自动分析结果,我们不对页面数据的有效性、准确性、正确性、可靠性、完整性和及时性做出任何承诺和保证。若有疑问,可以通过电子邮件方式联系我们:report@aminer.cn