An Improved Infrared Simulation Method Based on Generative Adversarial Networks
INFRARED PHYSICS & TECHNOLOGY(2024)
摘要
Infrared simulation technology has great application value in the fields of UAV target simulation and infrared signal processing. However, traditional infrared simulation technology has the disadvantages of poor scene adaptability, low fidelity of infrared simulation images and long training time. This paper proposes an infrared image simulation algorithm based on improved Generative Adversarial Networks(GAN) to generate multi-scene infrared images, which could provide a reliable expansion of infrared database. First, we construct a GAN model by introducing a multi-resolution hierarchical structure and attention mechanism, which can effectively enhance the model’s adaptability to different scenes during the simulation of generating infrared images. Secondly, the SSIM loss term and L1 loss term are introduced during the training process to form a composite loss function, which prompts the proposed GAN to generate high-fidelity infrared simulation images. Finally, while maintaining the quality of simulated infrared images, a lightweight model is designed through model compression to significantly reduce the number of model parameters.The traditional discriminator based on the UNet-self attention is replaced by a discriminator based on attention mechanisms and adaptive max-pooling structure. The proposed GAN is trained and tested on three public datasets. Experimental results demonstrate that the proposed method improves structural similarity by 11% compared to Pix2Pix and achieves a Peak Signal-to-Noise Ratio (PSNR) of 25.8, which is 3.9 higher than InfraGAN. At the same time, compared with InfraGAN, the number of model parameters is reduced by 68% , which makes the method efficiently obtain simulated infrared images corresponding to given visible images.
更多查看译文
关键词
Infrared simulation technology,Deep learning,Lightweight model,Generative adversarial networks
AI 理解论文
溯源树
样例
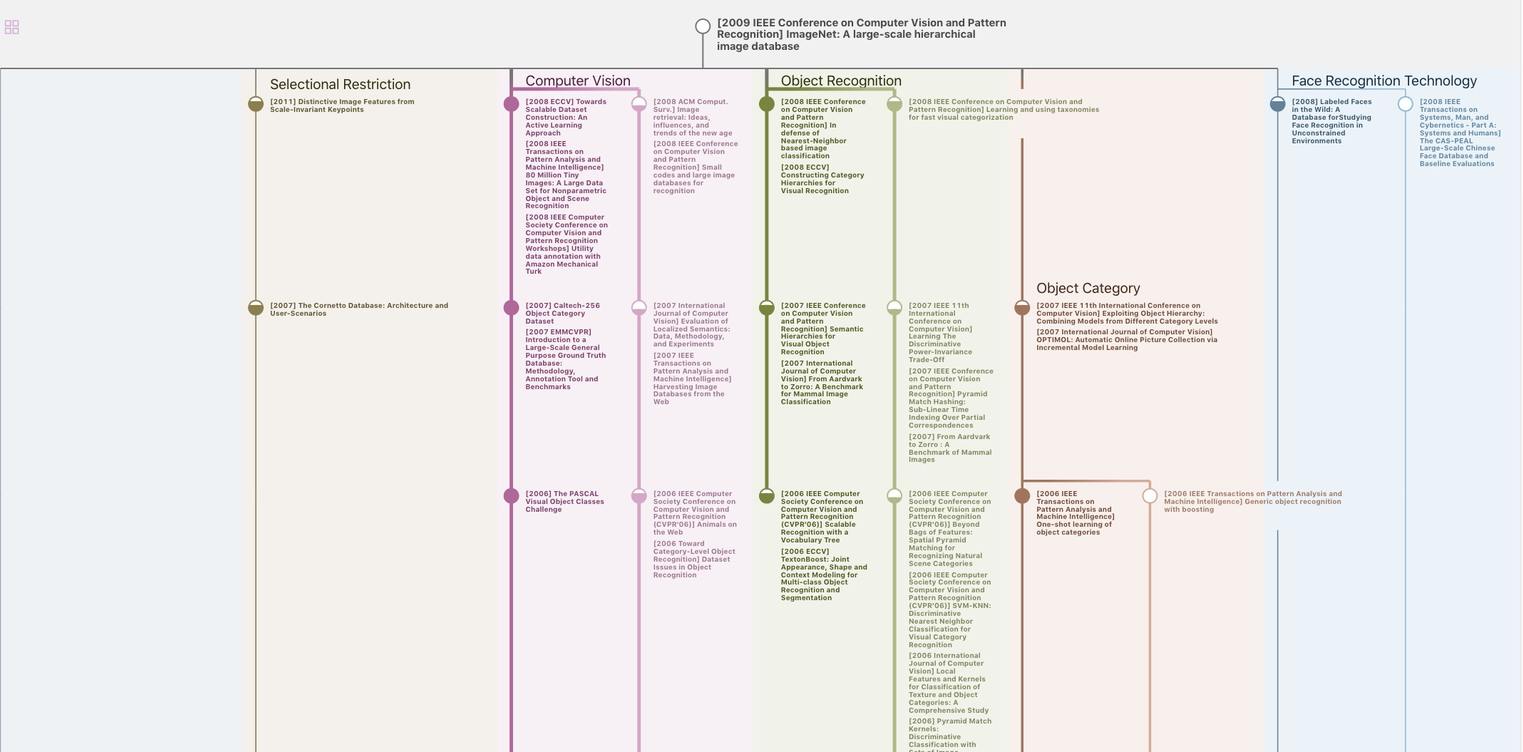
生成溯源树,研究论文发展脉络
数据免责声明
页面数据均来自互联网公开来源、合作出版商和通过AI技术自动分析结果,我们不对页面数据的有效性、准确性、正确性、可靠性、完整性和及时性做出任何承诺和保证。若有疑问,可以通过电子邮件方式联系我们:report@aminer.cn