Single-cell Gene Regulatory Network Analysis for Mixed Cell Populations.
QUANTITATIVE BIOLOGY(2024)
摘要
Gene regulatory network (GRN) refers to the complex network formed by regulatory interactions between genes in living cells. In this paper, we consider inferring GRNs in single cells based on single-cell RNA sequencing (scRNA-seq) data. In scRNA-seq, single cells are often profiled from mixed populations, and their cell identities are unknown. A common practice for single-cell GRN analysis is to first cluster the cells and infer GRNs for every cluster separately. However, this two-step procedure ignores uncertainty in the clustering step and thus could lead to inaccurate estimation of the networks. Here, we consider the mixture Poisson log-normal model (MPLN) for network inference of count data from mixed populations. The precision matrices of the MPLN are the GRNs of different cell types. To avoid the intractable optimization of the MPLN's log-likelihood, we develop an algorithm called variational mixture Poisson log-normal (VMPLN) to jointly estimate the GRNs of different cell types based on the variational inference method. We compare VMPLN with state-of-the-art single-cell regulatory network inference methods. Comprehensive simulation shows that VMPLN achieves better performance, especially in scenarios where different cell types have a high mixing degree. Benchmarking on real scRNA-seq data also demonstrates that VMPLN can provide more accurate network estimation in most cases. Finally, we apply VMPLN to a large scRNA-seq dataset from patients infected with severe acute respiratory syndrome coronavirus 2 (SARS-CoV-2) and find that VMPLN identifies critical differences in regulatory networks in immune cells between patients with moderate and severe symptoms. The source codes are available on the GitHub website (github.com/XiDsLab/SCVMPLN).
更多查看译文
关键词
gene regulatory network,graphical model,precision matrix,variational inference,single-cell RNA sequencing
AI 理解论文
溯源树
样例
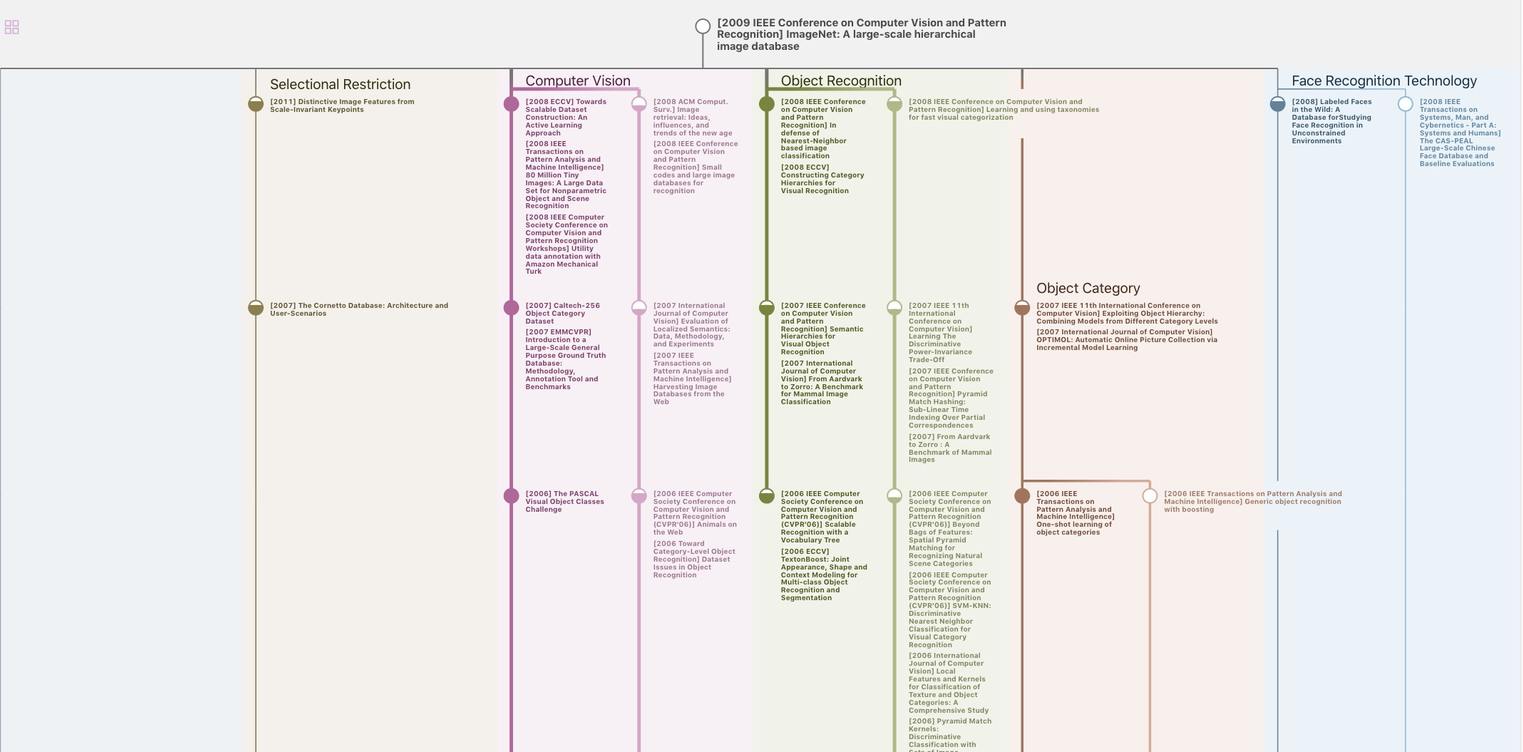
生成溯源树,研究论文发展脉络
数据免责声明
页面数据均来自互联网公开来源、合作出版商和通过AI技术自动分析结果,我们不对页面数据的有效性、准确性、正确性、可靠性、完整性和及时性做出任何承诺和保证。若有疑问,可以通过电子邮件方式联系我们:report@aminer.cn