Recognition Method of Cabbage Heads at Harvest Stage under Complex Background Based on Improved YOLOv8n
AGRICULTURE-BASEL(2024)
摘要
To address the problems of low recognition accuracy and slow processing speed when identifying harvest-stage cabbage heads in complex environments, this study proposes a lightweight harvesting period cabbage head recognition algorithm that improves upon YOLOv8n. We propose a YOLOv8n-Cabbage model, integrating an enhanced backbone network, the DyHead (Dynamic Head) module insertion, loss function optimization, and model light-weighting. To assess the proposed method, a comparison with extant mainstream object detection models is conducted. The experimental results indicate that the improved cabbage head recognition model proposed in this study can adapt cabbage head recognition under different lighting conditions and complex backgrounds. With a compact size of 4.8 MB, this model achieves 91% precision, 87.2% recall, and a mAP@50 of 94.5%—the model volume has been reduced while the evaluation metrics have all been improved over the baseline model. The results demonstrate that this model can be applied to the real-time recognition of harvest-stage cabbage heads under complex field environments.
更多查看译文
关键词
cabbage,recognition and localization,object detection,deep learning,automatic harvesting
AI 理解论文
溯源树
样例
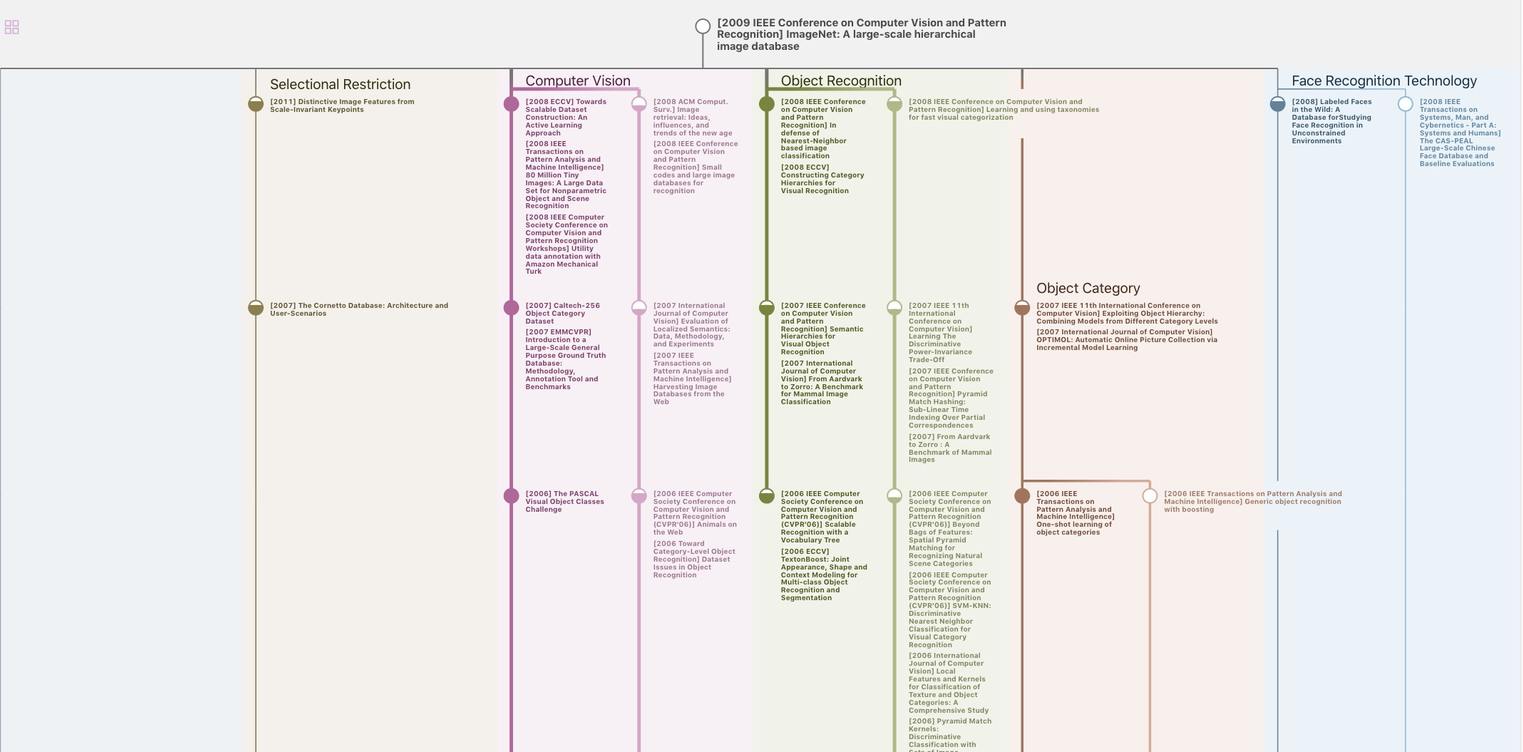
生成溯源树,研究论文发展脉络
数据免责声明
页面数据均来自互联网公开来源、合作出版商和通过AI技术自动分析结果,我们不对页面数据的有效性、准确性、正确性、可靠性、完整性和及时性做出任何承诺和保证。若有疑问,可以通过电子邮件方式联系我们:report@aminer.cn