Cost-Efficient Computation Offloading in VEC Using Deep Reinforcement Learning Techniques
20TH INTERNATIONAL WIRELESS COMMUNICATIONS & MOBILE COMPUTING CONFERENCE, IWCMC 2024(2024)
摘要
The rapid progression in autonomous driving and vehicle networking technologies has catalyzed the emergence of advanced vehicle applications, aiming to augment traffic safety and the driving experience. This advancement, however, is challenged by the limited computational and storage capacities inherent in on-board vehicle systems. To address this, Vehicle Edge Computing (VEC) emerges as a pivotal solution, enhancing vehicular computational capabilities. In this context, we introduce a novel VEC task offloading model utilizing Deep Reinforcement Learning (DRL). This model leverages the otherwise idle computational resources available in vehicles to facilitate efficient edge computing offloading within heterogeneous networks. A key innovation in our approach is the integration of Reinforcement Learning (RL) with Deep Learning (DL), significantly improving the convergence efficiency of the system. We also introduce an enhanced Q-learning algorithm tailored to jointly address the task offloading and processing challenges in VEC. This algorithm is adept at making optimal offloading decisions, aiming to minimize the overall system cost, encompassing both latency and energy consumption. Through rigorous simulation, our results demonstrate that this improved Q-learning approach substantially reduces total system costs while concurrently improving the quality of service in VEC environments. Our study not only offers a robust framework for computation offloading in vehicular networks but also paves the way for future research in AI-driven vehicular technology optimizations.
更多查看译文
关键词
Vehicle Edge Computing,Autonomous Driving,Heterogeneous Networks,Computation Offloading,Q-learning Algorithm,System Cost Minimization,Quality of Service,Vehicular Networking,AI in Transportation,Edge Computing Efficiency
AI 理解论文
溯源树
样例
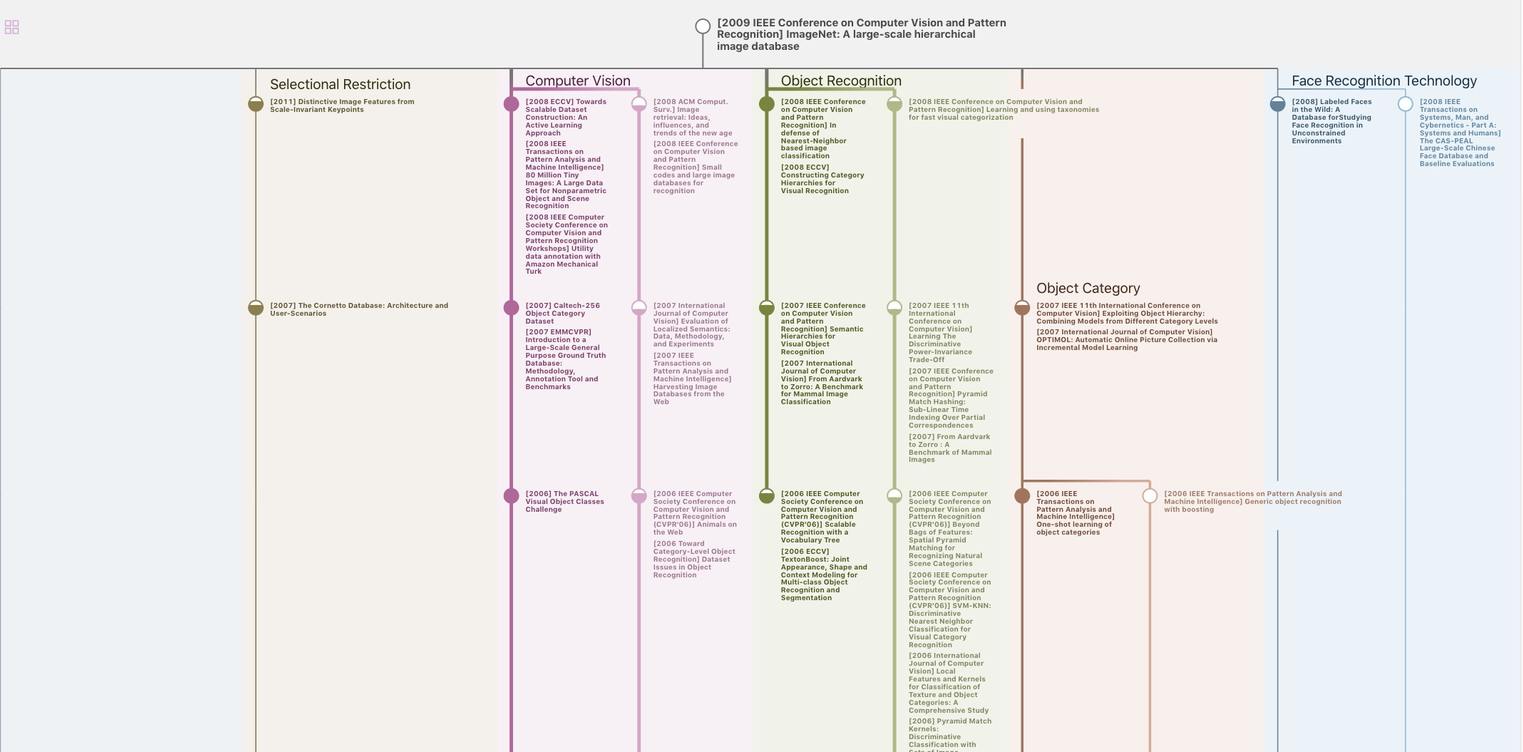
生成溯源树,研究论文发展脉络
数据免责声明
页面数据均来自互联网公开来源、合作出版商和通过AI技术自动分析结果,我们不对页面数据的有效性、准确性、正确性、可靠性、完整性和及时性做出任何承诺和保证。若有疑问,可以通过电子邮件方式联系我们:report@aminer.cn