Nnscaler: Constraint-Guided Parallelization Plan Generation for Deep Learning Training.
PROCEEDINGS OF THE 18TH USENIX SYMPOSIUM ON OPERATING SYSTEMS DESIGN AND IMPLEMENTATION, OSDI 2024(2024)
摘要
With the growing model size of deep neural networks (DNN), deep learning training is increasingly relying on handcrafted search spaces to find efficient parallelization execution plans. However, our study shows that existing search spaces exclude plans that significantly impact the training performance of well-known DNN models (e.g., AlphaFold2) under important settings, such as when handling large embedding tables in large language models. To address this problem, we propose nnScaler, a framework that generates efficient parallelization plans for deep learning training. Instead of relying on the existing search space, nnScaler advocates a more general approach that empowers domain experts to construct their own search space through three primitives, op-trans, op-assign, and op-order, which capture model transformation and the temporal-spatial scheduling of the transformed model of any parallelization plans. To avoid space explosion, nnScaler allows the application of constraints to those primitives during space construction. With the proposed primitives and constraints, nnScaler can compose existing search spaces as well as new ones. Experiments show that nnScaler can find new parallelization plans in new search spaces that achieve up to 3.5x speedup compared to solutions such as DeepSpeed, Megatron-LM, and Alpa for popular DNN models like Swin-Transformer and AlphaFold2.
更多查看译文
AI 理解论文
溯源树
样例
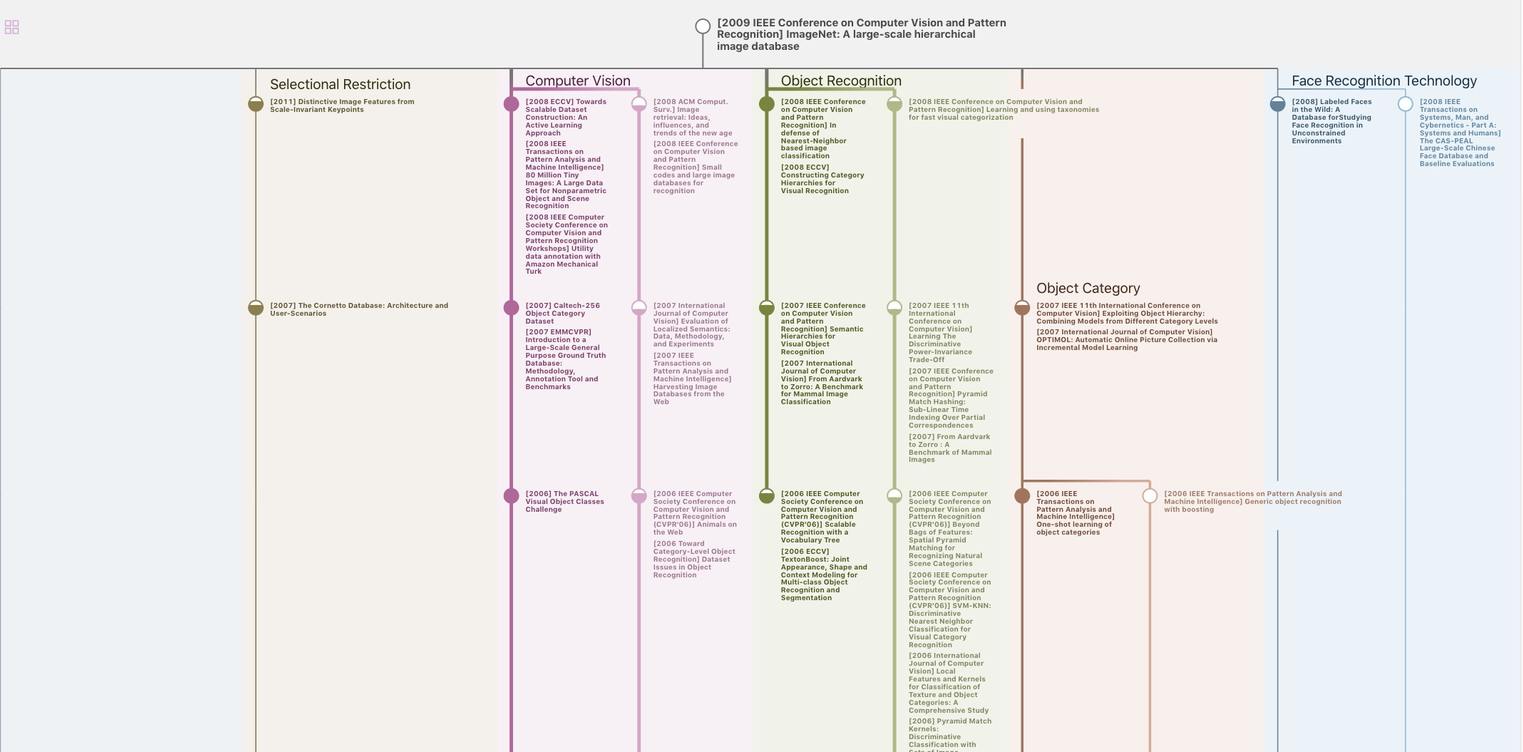
生成溯源树,研究论文发展脉络
数据免责声明
页面数据均来自互联网公开来源、合作出版商和通过AI技术自动分析结果,我们不对页面数据的有效性、准确性、正确性、可靠性、完整性和及时性做出任何承诺和保证。若有疑问,可以通过电子邮件方式联系我们:report@aminer.cn