Globally Informed Graph Contrastive Learning for Recommendation
ADVANCED INTELLIGENT COMPUTING TECHNOLOGY AND APPLICATIONS, PT XIII, ICIC 2024(2024)
摘要
In recent years, the fusion of graph convolutional networks (GCNs) with contrastive learning (CL) has emerged as a promising approach in recommender systems, owing to its adeptness in extracting self-supervised signals from the original data, thus addressing the challenge of data sparsity. While this methodology has been proven to be effective, it predominantly focuses on capturing discrepancies in user preferences through the construction of contrastive views, often neglecting the underlying commonalities in user preferences. To address this limitation, we introduce a novel framework, Globally Informed Graph Contrastive Learning (GIGCL), which aims to incorporate global features into the construction of contrastive views, facilitating the capture of shared features among nodes and refining the nodes' embedding outcomes. Central to our framework are four key modules, with the global features extractor module and the global informed fusion module serving as pivotal components. Following the initial embedding phase, local embeddings are fed into the global features extractor module to derive global features. Subsequently, in the global informed fusion module, the global features are seamlessly integrated with the local embeddings, generating a pair of contrastive views. Experimental results on two real-world datasets demonstrate the superiority of our proposed GIGCL over state-of-the-art baselines, and underscore the efficacy of GIGCL in reconciling divergent and convergent user preferences, thereby enhancing recommendation performance.
更多查看译文
关键词
Graph Convolutional Networks,Contrastive Learning,Collaborative Filtering,Recommender System
AI 理解论文
溯源树
样例
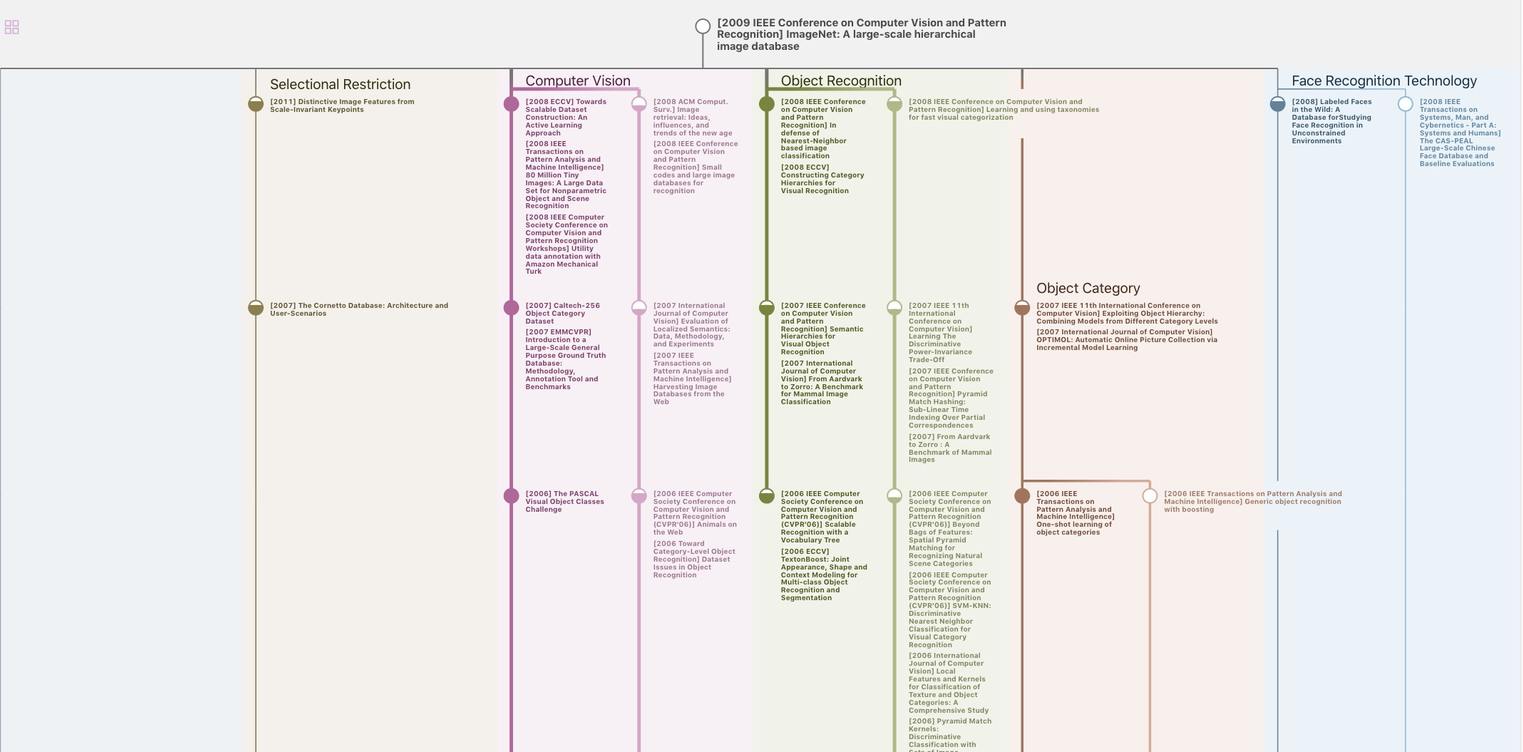
生成溯源树,研究论文发展脉络
数据免责声明
页面数据均来自互联网公开来源、合作出版商和通过AI技术自动分析结果,我们不对页面数据的有效性、准确性、正确性、可靠性、完整性和及时性做出任何承诺和保证。若有疑问,可以通过电子邮件方式联系我们:report@aminer.cn