Towards a Federated Intrusion Detection System based on Neuromorphic Computing
2024 9th International Conference on Smart and Sustainable Technologies (SpliTech)(2024)
摘要
Intrusion Detection Systems (IDSs) have emerged as essential tools for detecting cyber attacks and safeguarding sensitive data. Over time, there has been a shift towards designing IDSs that leverage Federated Learning (FL) methods, enabling them to detect attacks across distributed environments while upholding privacy-preserving manner. Concurrently, selecting the appropriate algorithm for Host execution, ensuring data privacy, low power consumption, and swift execution, has become a promising challenge. Recently, there has been a growing interest in Spike Neural Networks (SNNs) due to their ability to directly generate spikes and closely emulate human brain functions. SNN-based models are optimized to achieve energy efficiency by representing computations through asynchronously generated spikes. To tackle these challenges, we propose a theoretical approach for implementing a Federated Intrusion Detection System (IDS) that gathers data from different geographical locations, based on Neuromorphic Computing principles.
更多查看译文
关键词
Neuromorphic Computing,Artificial Intelligence,Federated Learning,Intrusion Detection System
AI 理解论文
溯源树
样例
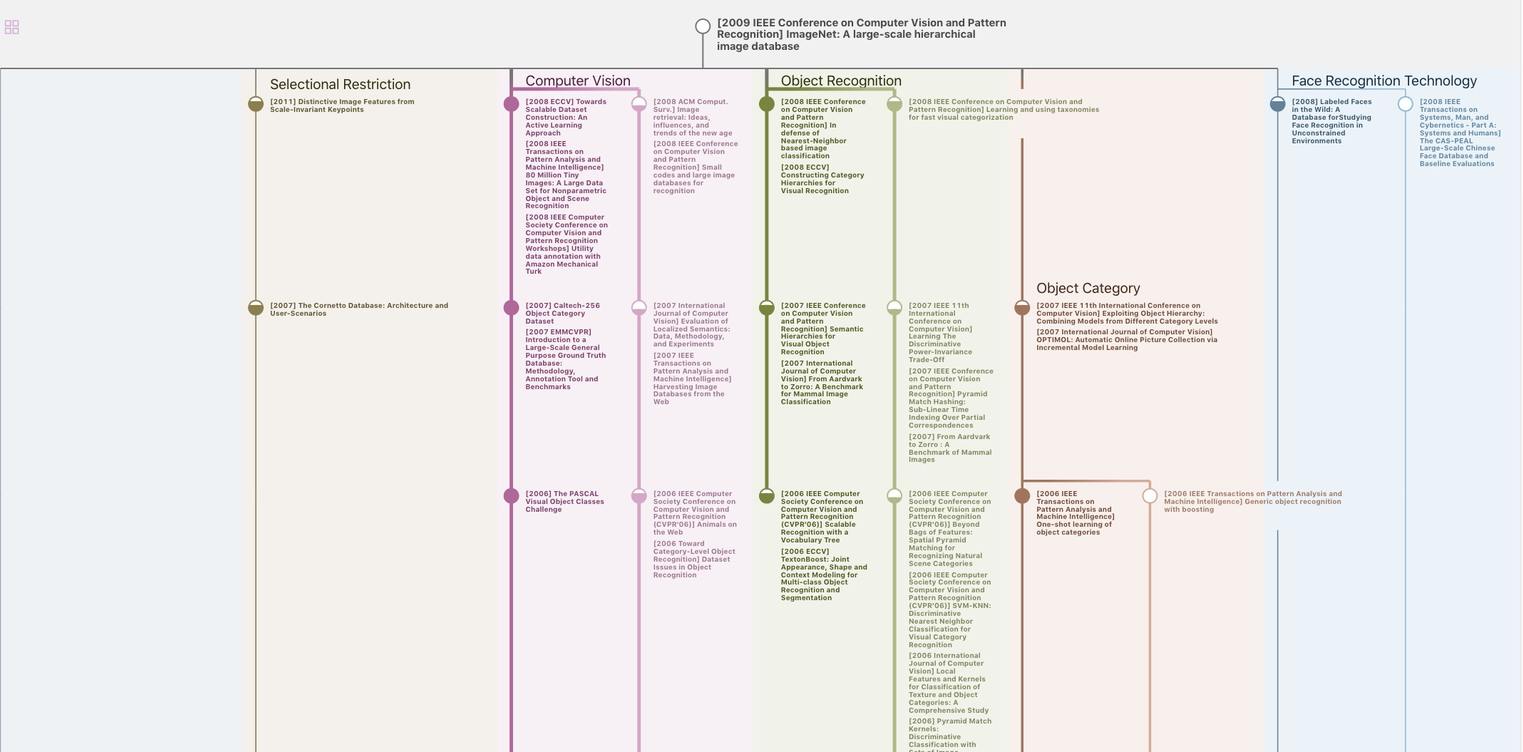
生成溯源树,研究论文发展脉络
数据免责声明
页面数据均来自互联网公开来源、合作出版商和通过AI技术自动分析结果,我们不对页面数据的有效性、准确性、正确性、可靠性、完整性和及时性做出任何承诺和保证。若有疑问,可以通过电子邮件方式联系我们:report@aminer.cn