A Deep-Learning-boosted Surrogate Model of a Metal Foam Based Protonic Ceramic Electrolysis Cell Stack for Uncertainty Quantification
ENERGY CONVERSION AND MANAGEMENT(2024)
摘要
Protonic ceramic electrolysis cells (PCECs) are widely considered as an emerging technology that shows capability for enabling environmentally friendly large-scale production of hydrogen. PCEC stacks' performance is considerably lower compared to smaller, lab-scale button cells. This performance gap is largely attributed to the highly uneven distribution of reactants throughout the larger cell or stack, which presents a significant challenge to the development of PCEC stack. The issue of non-uniform reactant distribution in PCEC stacks can be effectively addressed by incorporating metal foam structures to replace the conventional rib design of the interconnect components. A 3D multiphysical model is developed to train a deep-learning-boosted surrogate model for PCEC with metal foam, which is subsequently used for parameter screening and sensitivity analysis. A collection of 12 inputs that encompass operating/structural/microstructural/material parameters are chosen, in which the operating parameters are identified as influential parameters. Three distribution uniformity indices maintain strong consistence to variations in the inputs, implying the positive role of metal foam. Faradaic efficiency (FE) is primarily influenced by the operating voltage with a total Sobol index of 0.6. The current density and FE are determined as the most stable performance indicators, exhibiting standard deviations of 0.15 and 0.81 respectively. According to a safety threshold of 10 K cm(-1) for temperature gradient, the probability of the stack operating below this threshold is 88.61 %. Valuable insights into the design and performance of PCEC stacks that incorporate metal foam structures are provided. The workflow developed in this work can be further leveraged to explore and optimize PCECs.
更多查看译文
关键词
Metal foam,Hydrogen production,Uncertainty quantification,Polynomial Chaos Expansion, Deep neural network
AI 理解论文
溯源树
样例
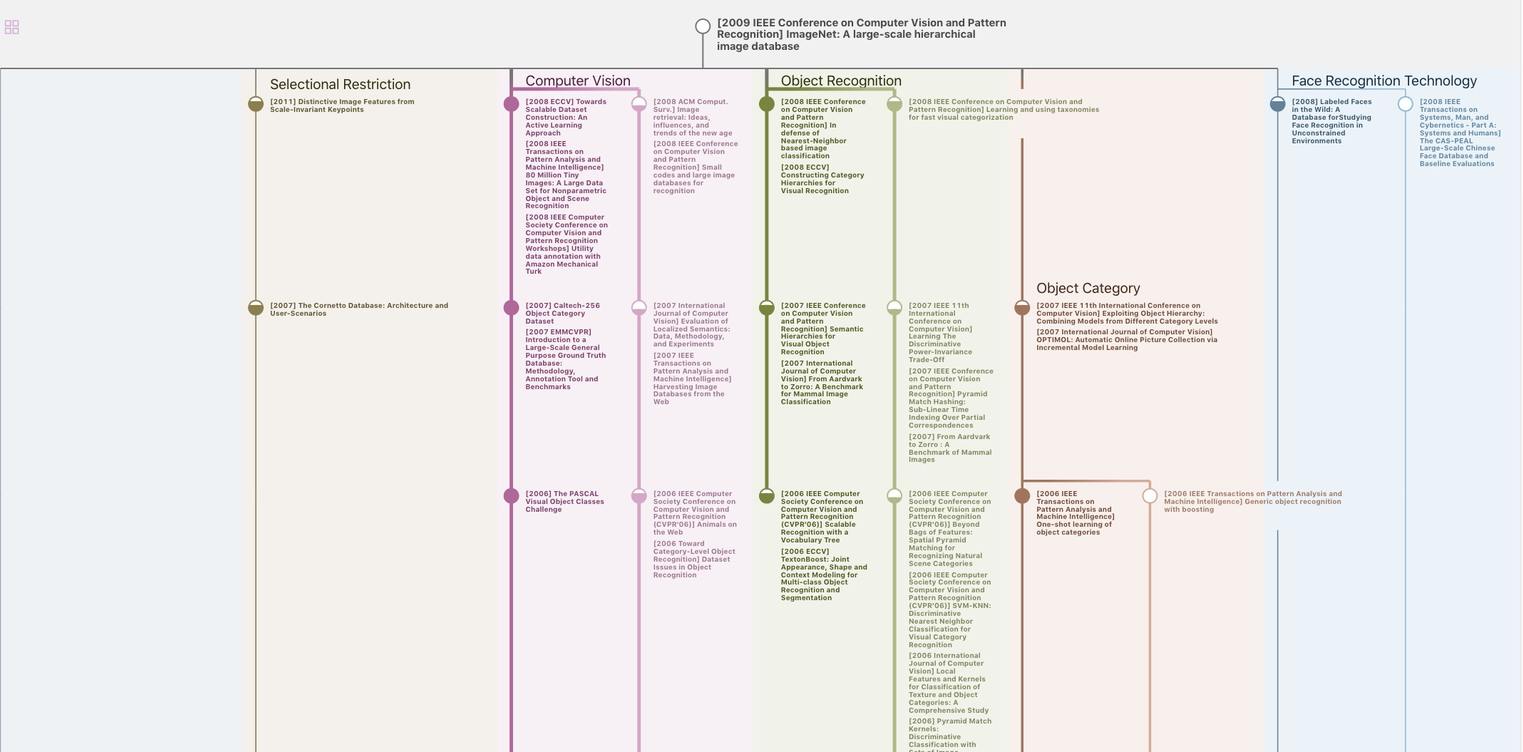
生成溯源树,研究论文发展脉络
数据免责声明
页面数据均来自互联网公开来源、合作出版商和通过AI技术自动分析结果,我们不对页面数据的有效性、准确性、正确性、可靠性、完整性和及时性做出任何承诺和保证。若有疑问,可以通过电子邮件方式联系我们:report@aminer.cn