Are Large Language Models Reliable Argument Quality Annotators?
ROBUST ARGUMENTATION MACHINES, RATIO 2024(2024)
摘要
Evaluating the quality of arguments is a crucial aspect of any systemleveraging argument mining. However, it is a challenge to obtain reliable andconsistent annotations regarding argument quality, as this usually requiresdomain-specific expertise of the annotators. Even among experts, the assessmentof argument quality is often inconsistent due to the inherent subjectivity ofthis task. In this paper, we study the potential of using state-of-the-artlarge language models (LLMs) as proxies for argument quality annotators. Toassess the capability of LLMs in this regard, we analyze the agreement betweenmodel, human expert, and human novice annotators based on an establishedtaxonomy of argument quality dimensions. Our findings highlight that LLMs canproduce consistent annotations, with a moderately high agreement with humanexperts across most of the quality dimensions. Moreover, we show that usingLLMs as additional annotators can significantly improve the agreement betweenannotators. These results suggest that LLMs can serve as a valuable tool forautomated argument quality assessment, thus streamlining and accelerating theevaluation of large argument datasets.
更多查看译文
关键词
Argumentation quality,Automated argument quality assessment,Large language models,Argument mining
AI 理解论文
溯源树
样例
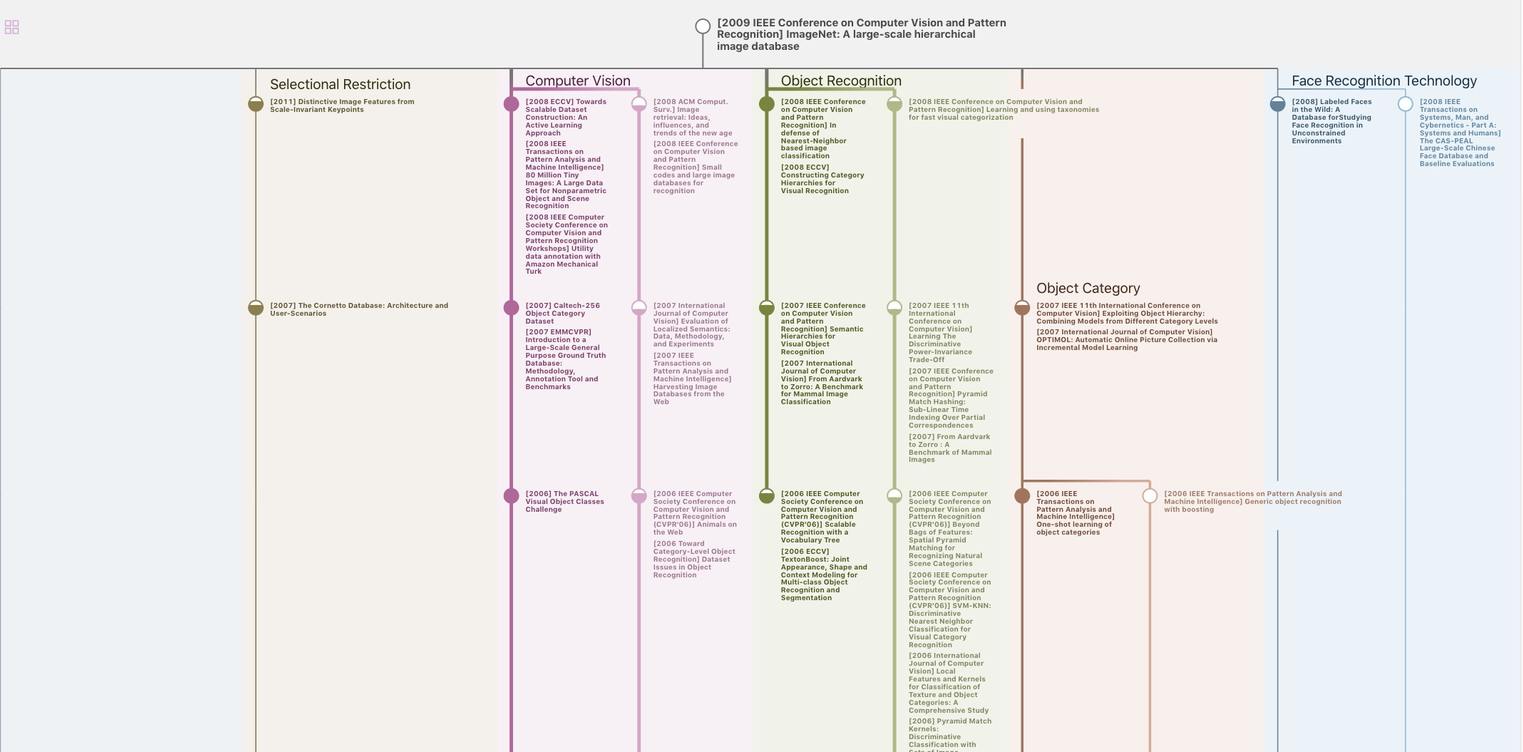
生成溯源树,研究论文发展脉络
数据免责声明
页面数据均来自互联网公开来源、合作出版商和通过AI技术自动分析结果,我们不对页面数据的有效性、准确性、正确性、可靠性、完整性和及时性做出任何承诺和保证。若有疑问,可以通过电子邮件方式联系我们:report@aminer.cn