Improving Security and Privacy in Advanced Federated Learning Environments for Cyber-Physical Systems
2024 IEEE INTERNATIONAL CONFERENCE ON COMMUNICATIONS WORKSHOPS, ICC WORKSHOPS 2024(2024)
摘要
Ensuring protection against a wide range of complex and advanced threats is crucial in the continually changing field of cyber-physical systems. This article examines the essential security and privacy elements in the context of novel service delivery models, explicitly focusing on federated learning as a fundamental paradigm. Our study aims to investigate the implementation of a synchronous reinforcement learning (RL) agent in a federated learning setting. Our purpose is to create resilient allocation strategies for cyber-physical systems. By engaging in synchronized training, RL agents establish efficient communication channels, enabling them to acquire a versatile policy that can effectively respond to many forms of assault. Our technique utilizes synchronous reinforcement learning in a federated learning context to create robust and adaptive systems prioritizing data protection in continuous data exchange settings. This study signifies a notable advancement in strengthening cyber-physical systems against developing threats, in line with the conference's focus on security and privacy for innovative service delivery models.
更多查看译文
关键词
Reinforcement Learning(RL),Federated Learning(FL),Cyber Physical Systems(CPS)
AI 理解论文
溯源树
样例
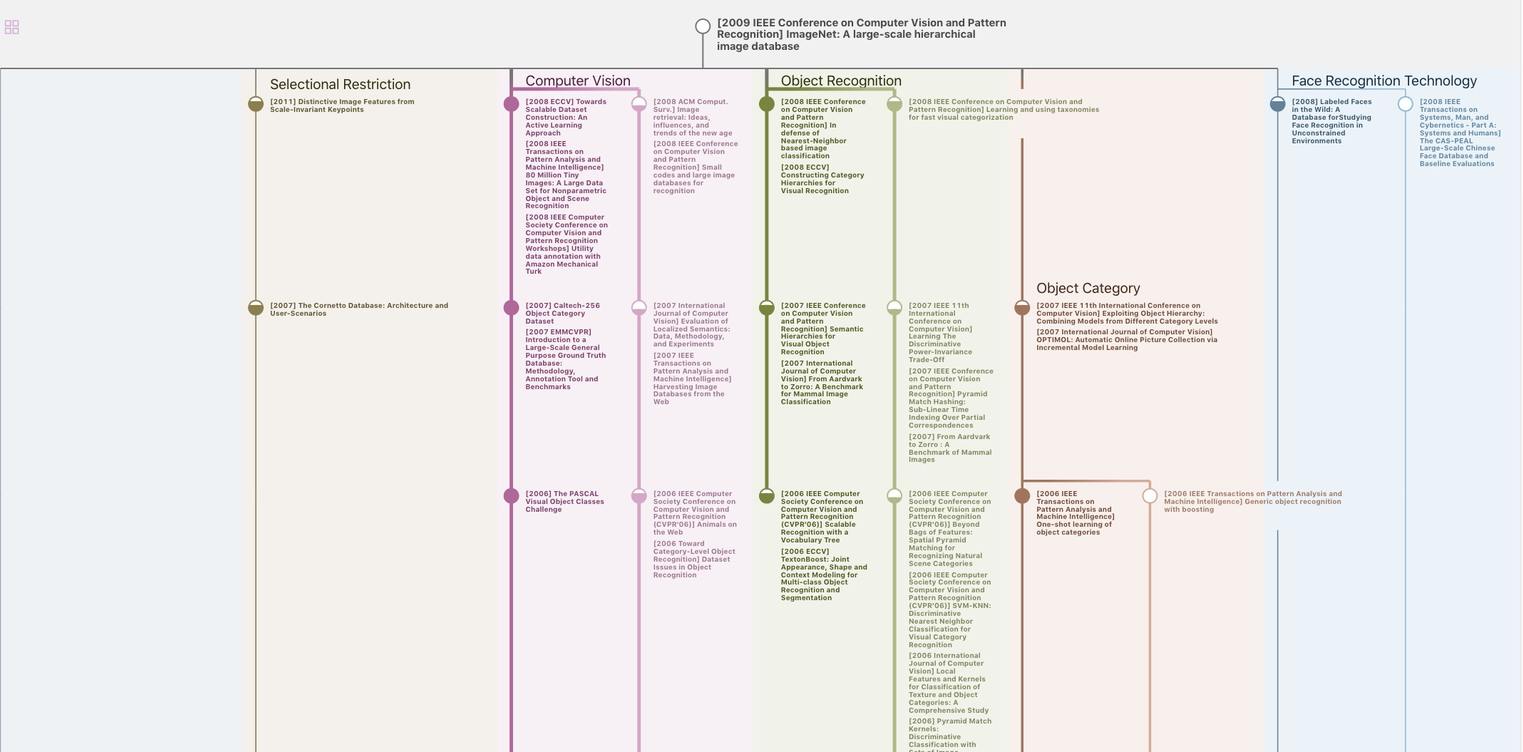
生成溯源树,研究论文发展脉络
数据免责声明
页面数据均来自互联网公开来源、合作出版商和通过AI技术自动分析结果,我们不对页面数据的有效性、准确性、正确性、可靠性、完整性和及时性做出任何承诺和保证。若有疑问,可以通过电子邮件方式联系我们:report@aminer.cn