Inverting the Leverage Score Gradient: an Efficient Approximate Newton Method
CoRR(2024)
摘要
Leverage scores have become essential in statistics and machine learning, aiding regression analysis, randomized matrix computations, and various other tasks. This paper delves into the inverse problem, aiming to recover the intrinsic model parameters given the leverage scores gradient. This endeavor not only enriches the theoretical understanding of models trained with leverage score techniques but also has substantial implications for data privacy and adversarial security. We specifically scrutinize the inversion of the leverage score gradient, denoted as g(x). An innovative iterative algorithm is introduced for the approximate resolution of the regularized least squares problem stated as min_x ∈ℝ^d 0.5 g(x) - c_2^2 + 0.5diag(w)Ax_2^2. Our algorithm employs subsampled leverage score distributions to compute an approximate Hessian in each iteration, under standard assumptions, considerably mitigating the time complexity. Given that a total of T = log( x_0 - x^* _2/ ϵ) iterations are required, the cost per iteration is optimized to the order of O( (nnz(A) + d^ω ) ·poly(log(n/δ)), where nnz(A) denotes the number of non-zero entries of A.
更多查看译文
AI 理解论文
溯源树
样例
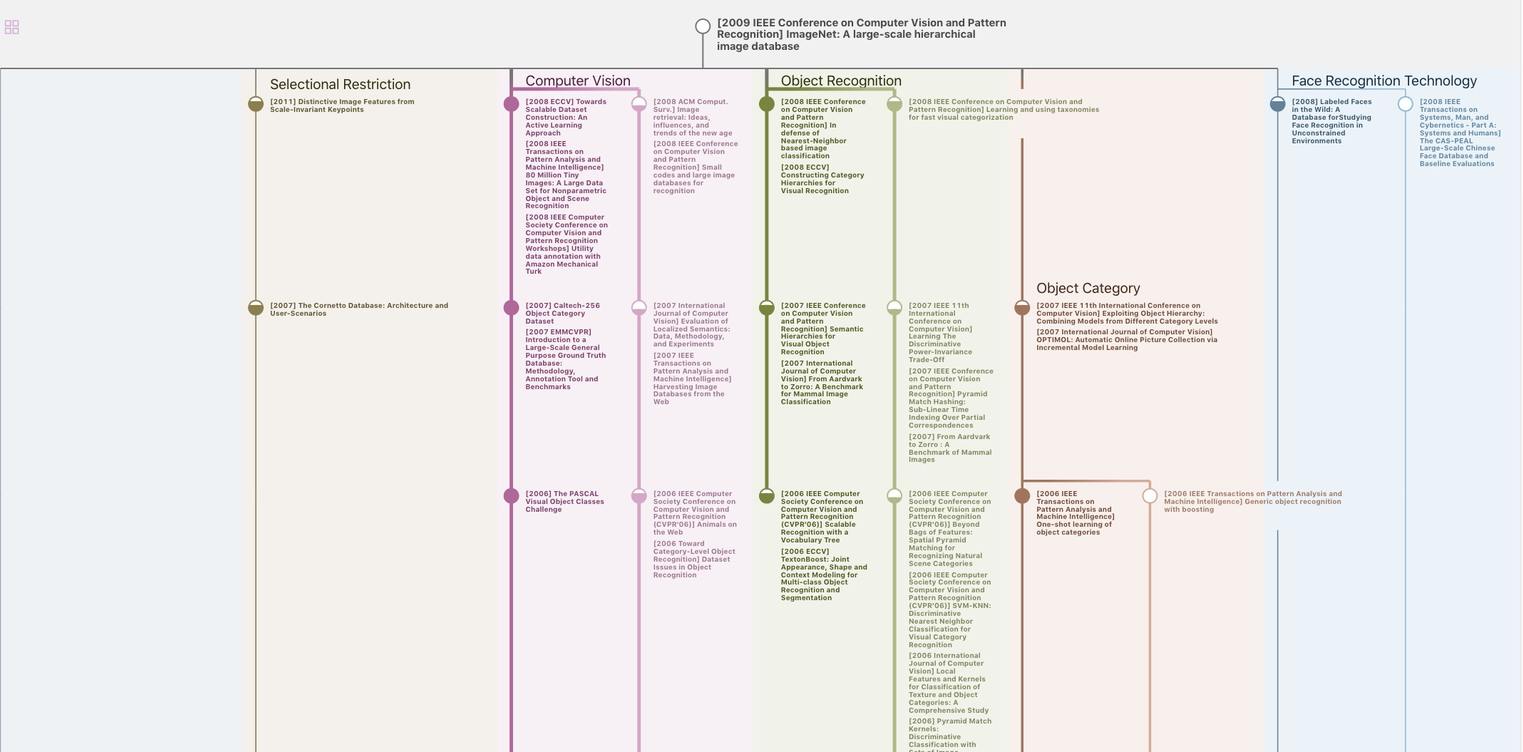
生成溯源树,研究论文发展脉络
数据免责声明
页面数据均来自互联网公开来源、合作出版商和通过AI技术自动分析结果,我们不对页面数据的有效性、准确性、正确性、可靠性、完整性和及时性做出任何承诺和保证。若有疑问,可以通过电子邮件方式联系我们:report@aminer.cn