RelKD 2024: the Second International Workshop on Resource-Efficient Learning for Knowledge Discovery
KDD 2024(2024)
摘要
Modern machine learning techniques, particularly deep learning, have showcased remarkable efficacy across numerous knowledge discovery and data mining applications. However, the advancement of many of these methods is frequently impeded by resource constraint challenges in many scenarios, such as limited labeled data (data-level), small model size requirements in real-world computing platforms (model-level), and efficient mapping of the computations to heterogeneous target hardware (system-level). Addressing all these factors is crucial for effectively and efficiently deploying developed models across a broad spectrum of real-world systems, including large-scale social network analysis, recommendation systems, and real-time anomaly detection. Therefore, there is a critical need to develop efficient learning techniques to address the challenges posed by resource limitations, whether from data, model/algorithm, or system/hardware perspectives. The proposed international workshop on "Resource-Efficient Learning for Knowledge Discovery (RelKD 2024)" will provide a great venue for academic researchers and industrial practitioners to share challenges, solutions, and future opportunities of resource-efficient learning.
更多查看译文
关键词
Resource-Efficient Learning,Knowledge Discovery,Machine Learning,Data Mining,Constraint Challenges,Workshop
AI 理解论文
溯源树
样例
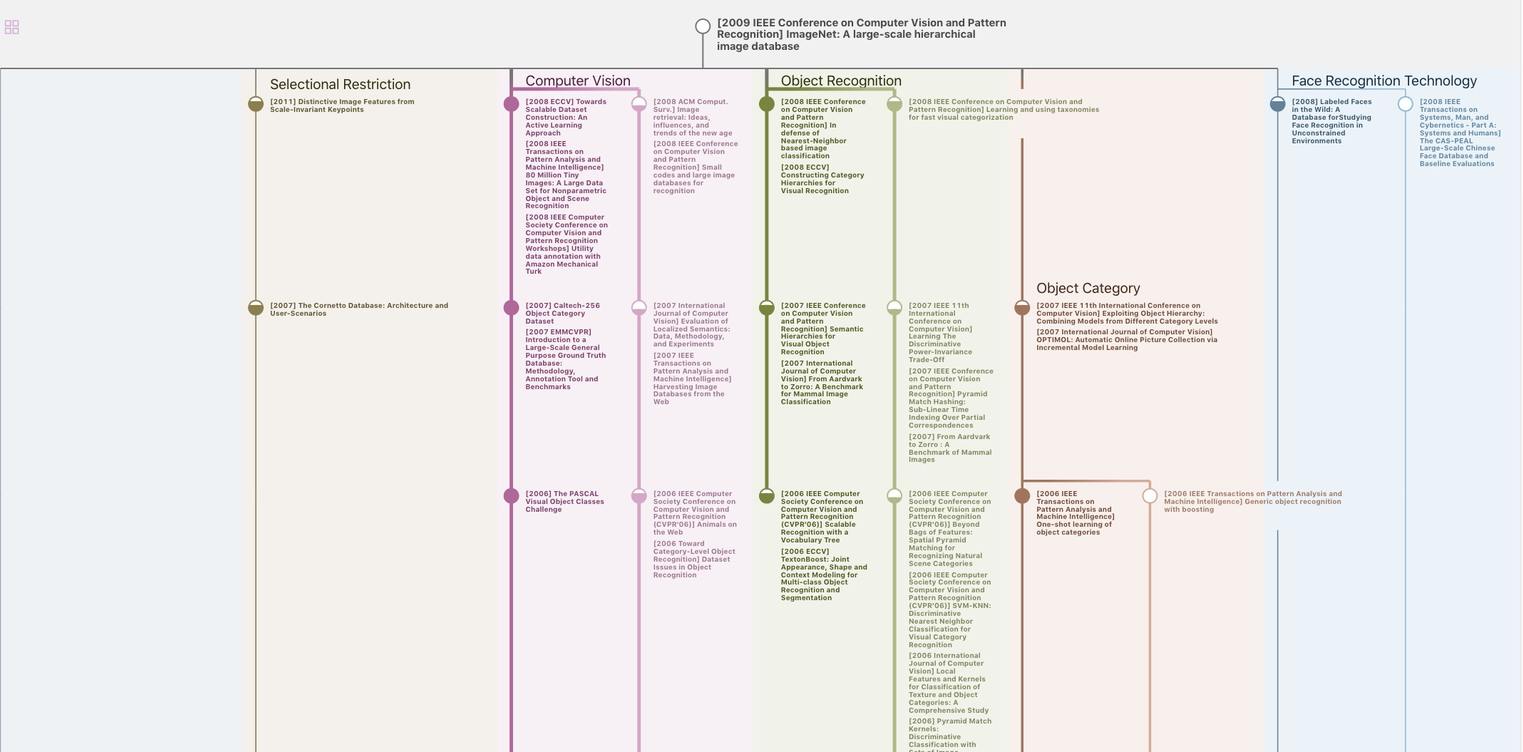
生成溯源树,研究论文发展脉络
数据免责声明
页面数据均来自互联网公开来源、合作出版商和通过AI技术自动分析结果,我们不对页面数据的有效性、准确性、正确性、可靠性、完整性和及时性做出任何承诺和保证。若有疑问,可以通过电子邮件方式联系我们:report@aminer.cn