A Patient-Specific Auto-Planning Method for MRI-guided Adaptive Radiotherapy in Prostate Cancer
RADIOTHERAPY AND ONCOLOGY(2024)
摘要
Background and purpose: Fast and automated generation of treatment plans is desirable for magnetic resonance imaging (MRI)-guided adaptive radiotherapy (MRIgART). This study proposed a novel patient-specific autoplanning method and validated its feasibility in improving the existing online planning workflow. Materials and methods: Data from 40 patients with prostate cancer were collected retrospectively. A patient-specific auto-planning method was proposed to generate adaptive treatment plans. First, a population dose-prediction model (M-0) was trained using data from previous patients. Second, a patient-specific model (M-ps) was created for each new patient by fine-tuning M-0 with the patient's data. Finally, an auto plan was optimized using the parameters derived from the predicted dose distribution by M-ps. The auto plans were compared with manual plans in terms of plan quality, efficiency, dosimetric verification, and clinical evaluation. Results: The auto plans improved target coverage, reduced irradiation to the rectum, and provided comparable protection to other organs-at-risk. Target coverage for the planning target volume (+0.61 %, P = 0.023) and clinical target volume 4000 (+1.60 %, P < 0.001) increased. V-2900cGy (-1.06 %, P = 0.004) and V-1810cGy (-2.49 %, P < 0.001) to the rectal wall and V-1810cGy (-2.82 %, P = 0.012) to the rectum were significantly reduced. The auto plans required less planning time (-3.92 min, P = 0.001), monitor units (-46.48, P = 0.003), and delivery time (-0.26 min, P = 0.004), and their gamma pass rates (3 %/2 mm) were higher (+0.47 %, P = 0.014). Conclusion: The proposed patient-specific auto-planning method demonstrated a robust level of automation and was able to generate high-quality treatment plans in less time for MRIgART in prostate cancer.
更多查看译文
关键词
MRI-guided adaptive radiotherapy,Auto-planning,Patient-specific,Dose prediction,Deep learning
AI 理解论文
溯源树
样例
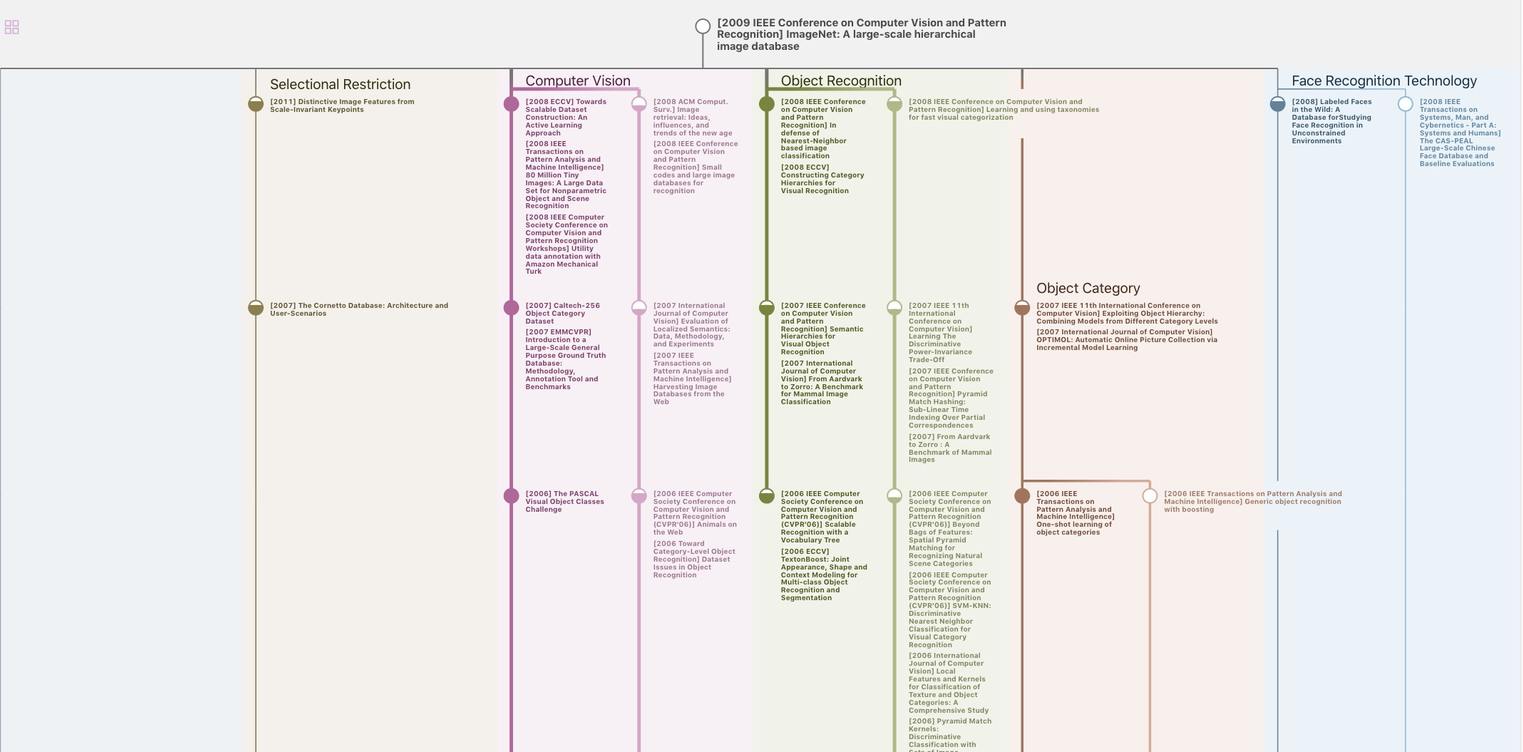
生成溯源树,研究论文发展脉络
数据免责声明
页面数据均来自互联网公开来源、合作出版商和通过AI技术自动分析结果,我们不对页面数据的有效性、准确性、正确性、可靠性、完整性和及时性做出任何承诺和保证。若有疑问,可以通过电子邮件方式联系我们:report@aminer.cn