SimulBench: Evaluating Language Models with Creative Simulation Tasks
CoRR(2024)
摘要
We introduce SimulBench, a benchmark designed to evaluate large language models (LLMs) across a diverse collection of creative simulation scenarios, such as acting as a Linux terminal or playing text games with users. While these simulation tasks serve as effective measures of an LLM's general intelligence, they are seldom incorporated into existing benchmarks. A major challenge is to develop an evaluation framework for testing different LLMs fairly while preserving the multi-round interactive nature of simulation tasks between users and AI. To tackle this issue, we suggest using a fixed LLM as a user agent to engage with an LLM to collect dialogues first under different tasks. Then, challenging dialogue scripts are extracted for evaluating different target LLMs. To facilitate automatic assessment on \DataName{}, GPT-4 is employed as the evaluator, tasked with reviewing the quality of the final response generated by the target LLMs given multi-turn dialogue scripts. Our comprehensive experiments indicate that these simulation tasks continue to pose a significant challenge with their unique natures and show the gap between proprietary models and the most advanced open LLMs. For example, GPT-4-turbo outperforms LLaMA-3-70b-Chat on 18.55\% more cases.
更多查看译文
AI 理解论文
溯源树
样例
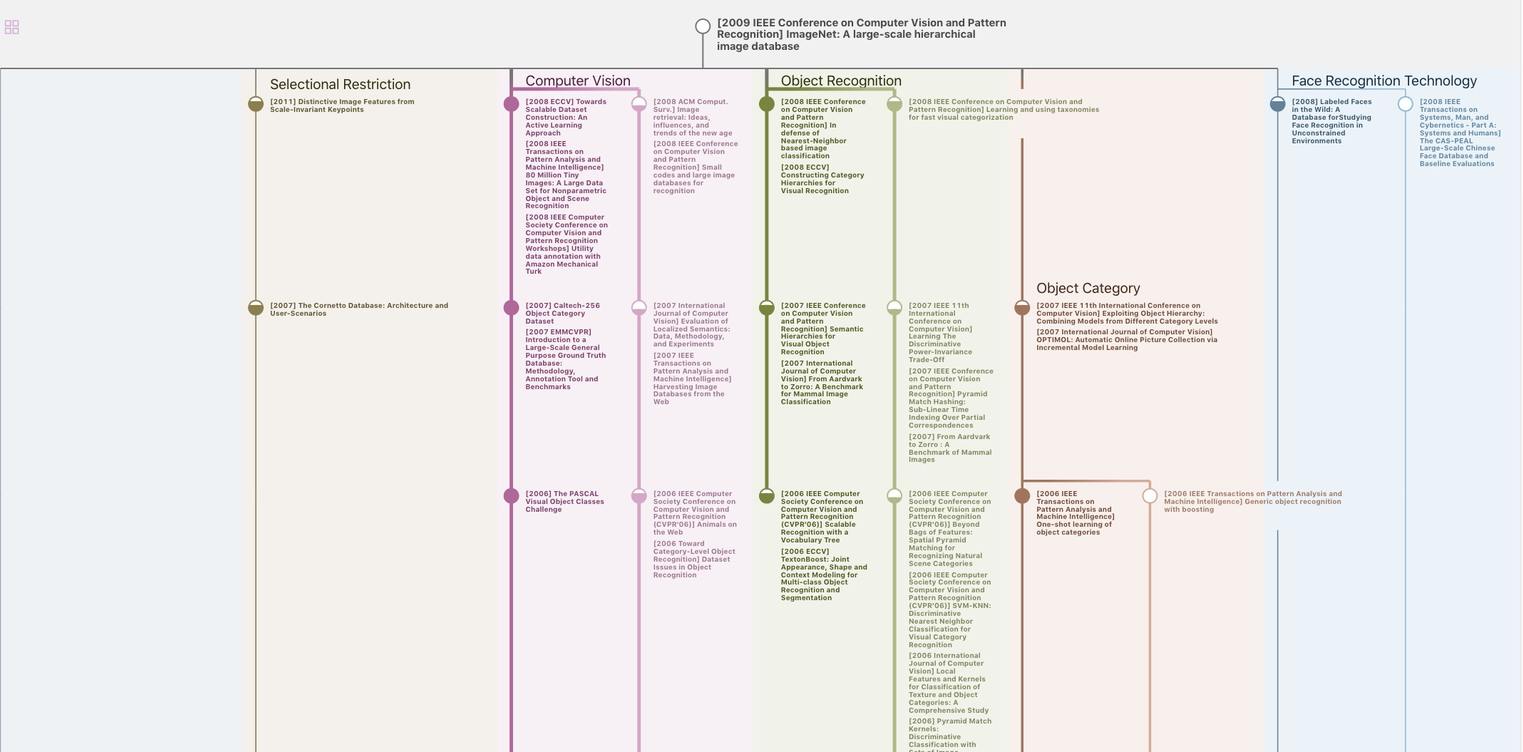
生成溯源树,研究论文发展脉络
数据免责声明
页面数据均来自互联网公开来源、合作出版商和通过AI技术自动分析结果,我们不对页面数据的有效性、准确性、正确性、可靠性、完整性和及时性做出任何承诺和保证。若有疑问,可以通过电子邮件方式联系我们:report@aminer.cn