Debiased Prototypical Learning Improves Generalized Category Discovery
IEEE International Conference on Multimedia and Expo(2024)
摘要
Generalized Category Discovery (GCD) aims to discover novel and seen categories using knowledge from labeled samples. Existing methods like SimGCD showed a bias towards predicting seen categories. We identify that the primary source of misclassification in these methods is the incorrect categorization of unseen categories as seen categories. To mitigate this bias, we introduce a novel approach that leverages labels generated via k-means++ for each training example to compute dynamic prototypes for each mini-batch, providing more balanced supervision. Additionally, we incorporate prototypical contrastive learning loss that performs contrastive learning on these prototypes, reducing the bias towards seen categories and facilitating the learning of unbiased representations. Extensive experimental results on various GCD benchmarks reveal that our approach not only reduces the seen category bias in representation learning but also sets a new state-of-the-art performance across all tested benchmarks.
更多查看译文
关键词
Generalized Category Discovery,Semi-Supervised Learning,Contrastive Learning,Clustering
AI 理解论文
溯源树
样例
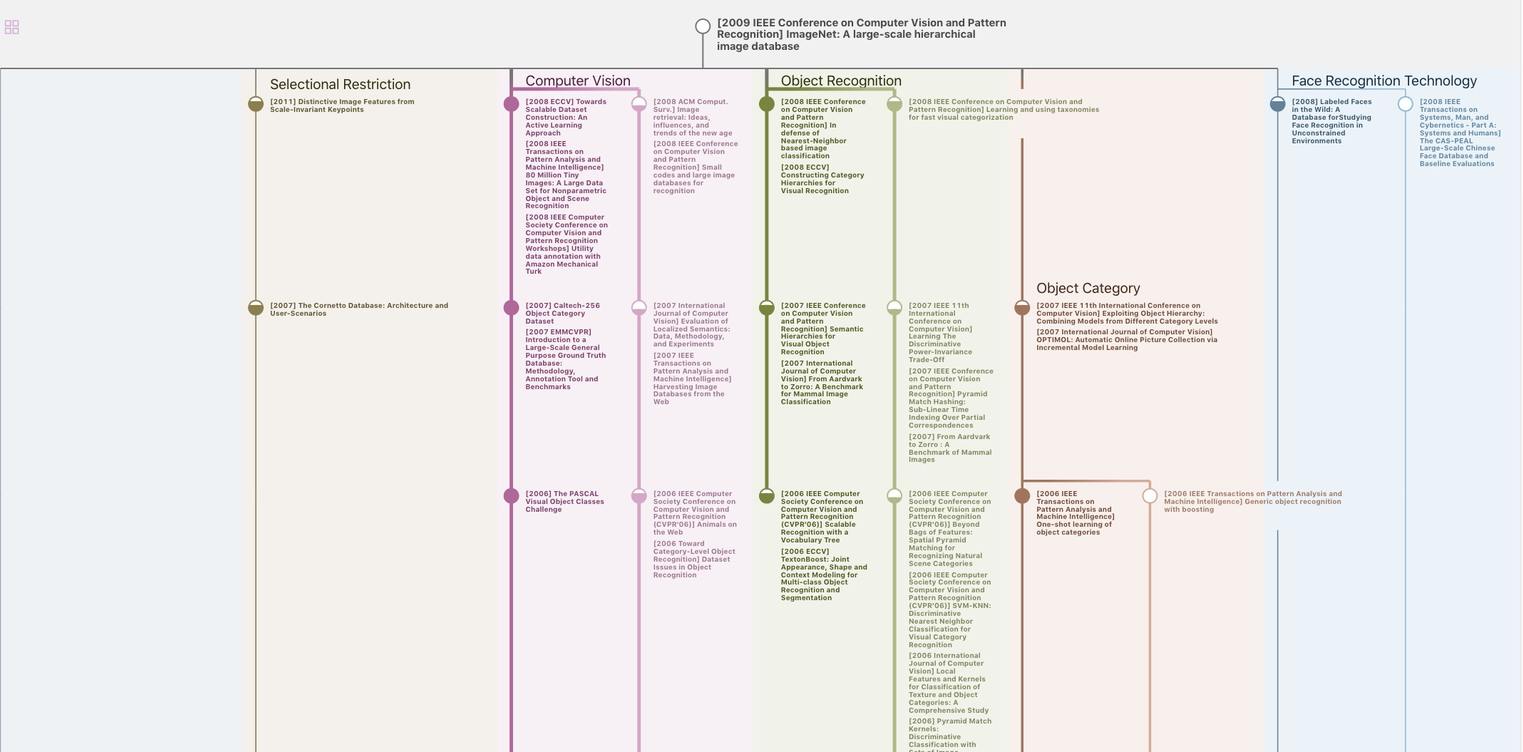
生成溯源树,研究论文发展脉络
数据免责声明
页面数据均来自互联网公开来源、合作出版商和通过AI技术自动分析结果,我们不对页面数据的有效性、准确性、正确性、可靠性、完整性和及时性做出任何承诺和保证。若有疑问,可以通过电子邮件方式联系我们:report@aminer.cn