Nonideality-aware Training Makes Memristive Networks More Robust to Adversarial Attacks
CoRR(2024)
摘要
Neural networks are now deployed in a wide number of areas from object classification to natural language systems. Implementations using analog devices like memristors promise better power efficiency, potentially bringing these applications to a greater number of environments. However, such systems suffer from more frequent device faults and overall, their exposure to adversarial attacks has not been studied extensively. In this work, we investigate how nonideality-aware training - a common technique to deal with physical nonidealities - affects adversarial robustness. We find that adversarial robustness is significantly improved, even with limited knowledge of what nonidealities will be encountered during test time.
更多查看译文
AI 理解论文
溯源树
样例
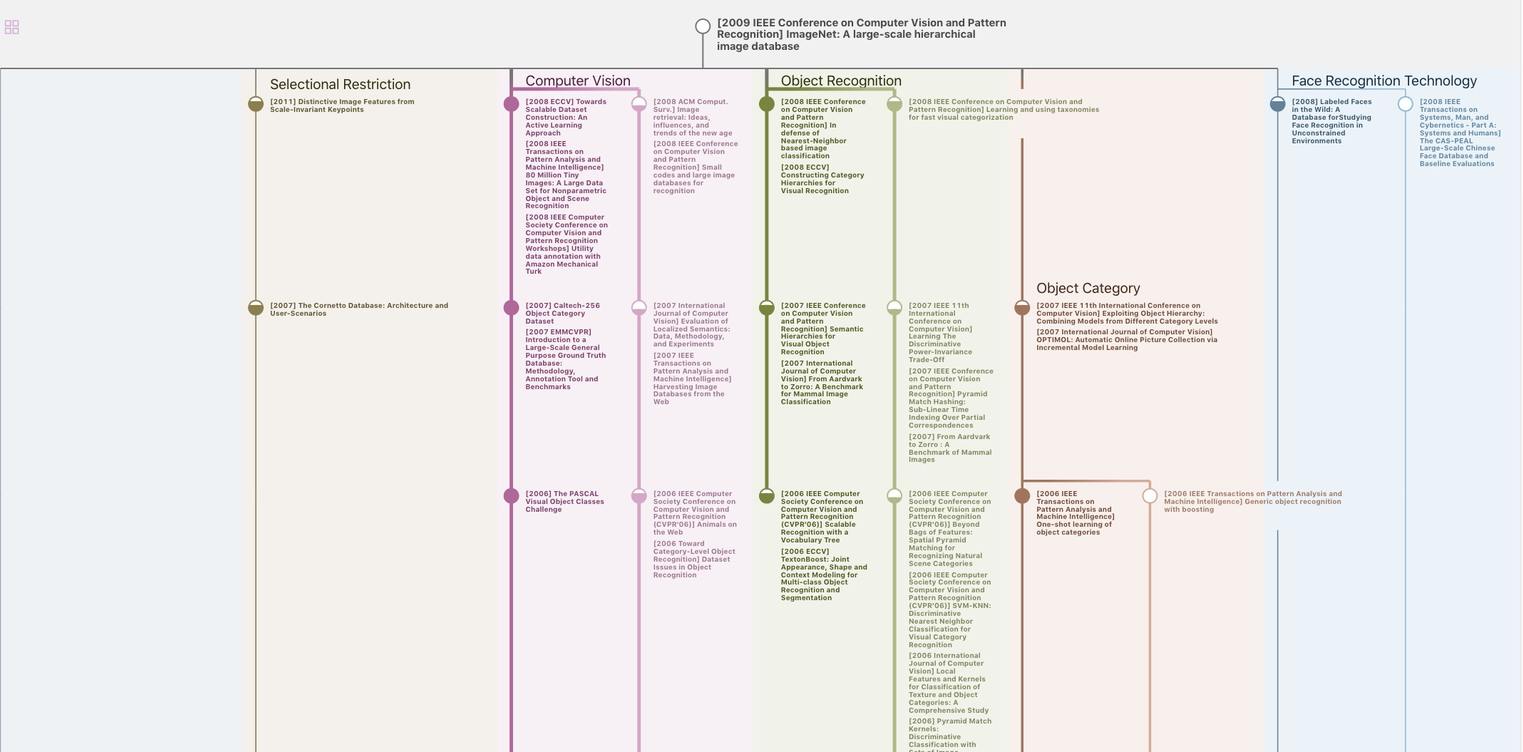
生成溯源树,研究论文发展脉络
数据免责声明
页面数据均来自互联网公开来源、合作出版商和通过AI技术自动分析结果,我们不对页面数据的有效性、准确性、正确性、可靠性、完整性和及时性做出任何承诺和保证。若有疑问,可以通过电子邮件方式联系我们:report@aminer.cn