Spatial-channel Transformer Network Based on Mask-Rcnn for Efficient Mushroom Instance Segmentation
INTERNATIONAL JOURNAL OF AGRICULTURAL AND BIOLOGICAL ENGINEERING(2024)
摘要
Edible mushrooms are rich in nutrients; however, harvesting mainly relies on manual labor. Coarse localization of each mushroom is necessary to enable a robotic arm to accurately pick edible mushrooms. Previous studies used detection algorithms that did not consider mushroom pixel-level information. When these algorithms are combined with a depth map, the information is lost. Moreover, in instance segmentation algorithms, convolutional neural network (CNN)-based methods are lightweight, and the extracted features are not correlated. To guarantee real-time location detection and improve the accuracy of mushroom segmentation, this study proposed a new spatial-channel transformer network model based on Mask-CNN (SCTMask-RCNN). The fusion of Mask-RCNN with the self-attention mechanism extracts the global correlation outcomes of image features from the channel and spatial dimensions. Subsequently, Mask-RCNN was used to maintain a lightweight structure and extract local features using a spatial pooling pyramidal structure to achieve multiscale local feature fusion and improve detection accuracy. The results showed that the SCT-Mask-RCNN method achieved a segmentation accuracy of 0.750 on segm_Precision_mAP and detection accuracy of 0.638 on Bbox_Precision_mAP. Compared to existing methods, the proposed method improved the accuracy of the evaluation metrics Bbox_Precision_mAP and segm_Precision_mAP by over 2% and 5%, respectively.
更多查看译文
关键词
edible mushrooms,picking,instance segmentation,deep learning,algorithm
AI 理解论文
溯源树
样例
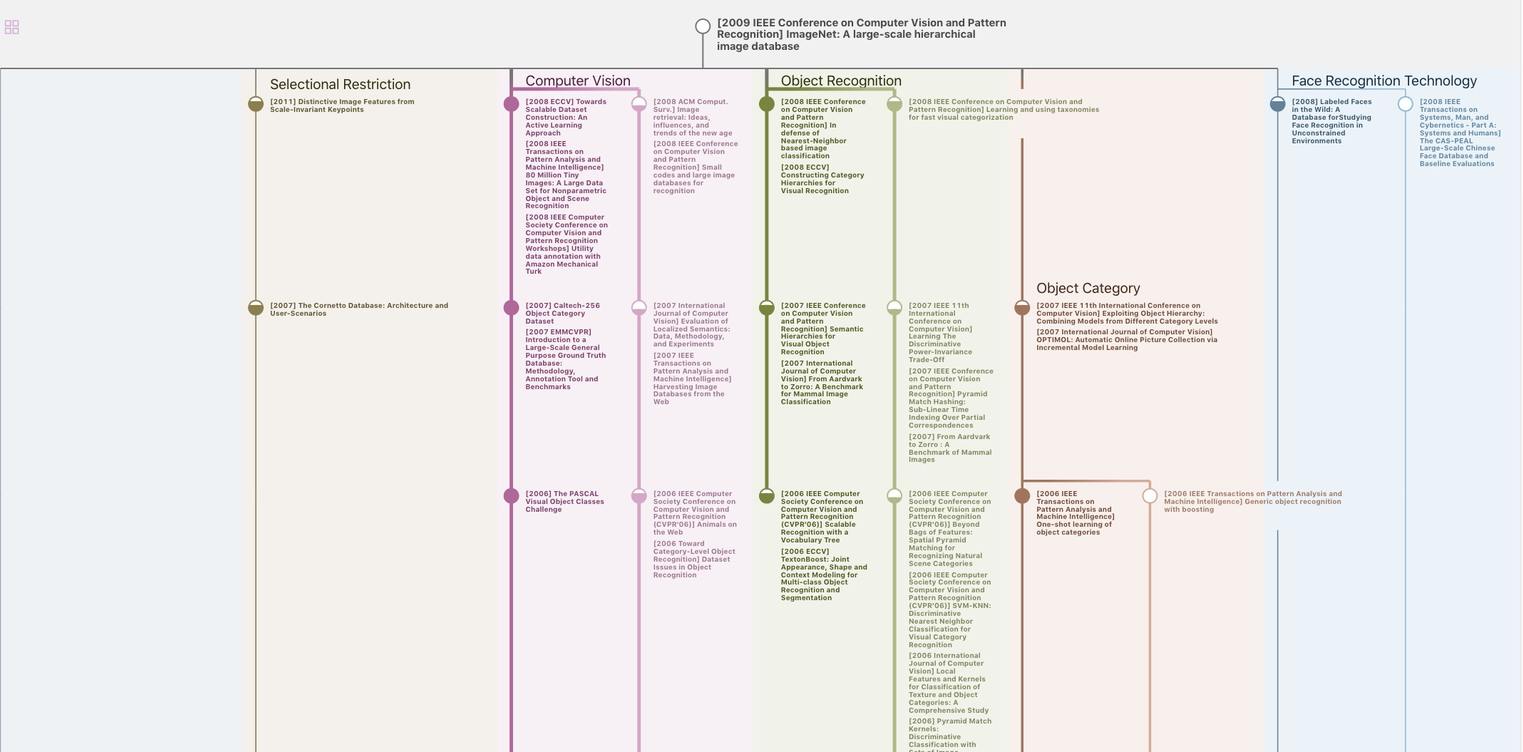
生成溯源树,研究论文发展脉络
数据免责声明
页面数据均来自互联网公开来源、合作出版商和通过AI技术自动分析结果,我们不对页面数据的有效性、准确性、正确性、可靠性、完整性和及时性做出任何承诺和保证。若有疑问,可以通过电子邮件方式联系我们:report@aminer.cn