Choices Are More Important Than Efforts: LLM Enables Efficient Multi-Agent Exploration
CoRR(2024)
摘要
With expansive state-action spaces, efficient multi-agent exploration remains a longstanding challenge in reinforcement learning. Although pursuing novelty, diversity, or uncertainty attracts increasing attention, redundant efforts brought by exploration without proper guidance choices poses a practical issue for the community. This paper introduces a systematic approach, termed LEMAE, choosing to channel informative task-relevant guidance from a knowledgeable Large Language Model (LLM) for Efficient Multi-Agent Exploration. Specifically, we ground linguistic knowledge from LLM into symbolic key states, that are critical for task fulfillment, in a discriminative manner at low LLM inference costs. To unleash the power of key states, we design Subspace-based Hindsight Intrinsic Reward (SHIR) to guide agents toward key states by increasing reward density. Additionally, we build the Key State Memory Tree (KSMT) to track transitions between key states in a specific task for organized exploration. Benefiting from diminishing redundant explorations, LEMAE outperforms existing SOTA approaches on the challenging benchmarks (e.g., SMAC and MPE) by a large margin, achieving a 10x acceleration in certain scenarios.
更多查看译文
AI 理解论文
溯源树
样例
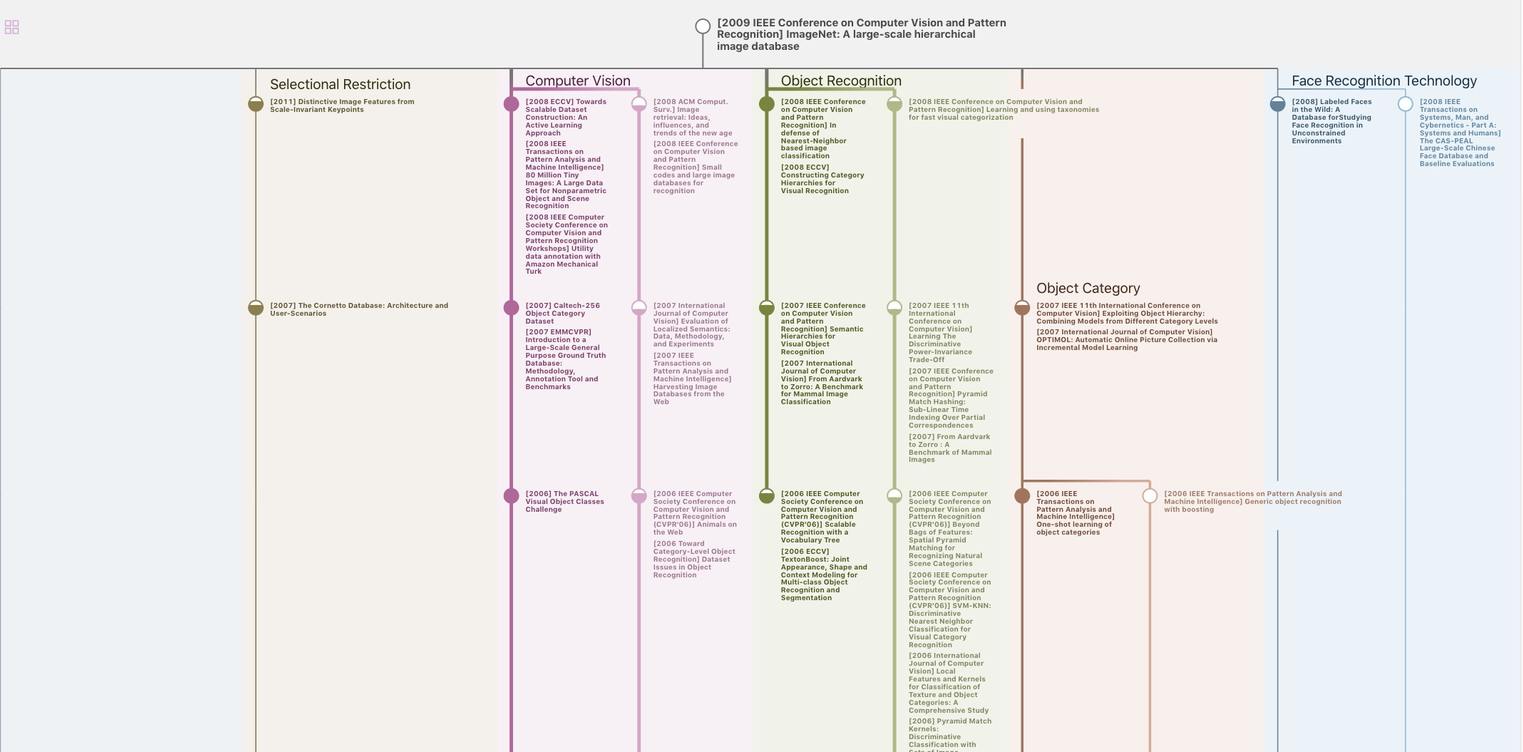
生成溯源树,研究论文发展脉络
数据免责声明
页面数据均来自互联网公开来源、合作出版商和通过AI技术自动分析结果,我们不对页面数据的有效性、准确性、正确性、可靠性、完整性和及时性做出任何承诺和保证。若有疑问,可以通过电子邮件方式联系我们:report@aminer.cn