SAM-NeRF: NeRF-Based 3D Instance Segmentation with Segment Anything Model.
ARTIFICIAL NEURAL NETWORKS AND MACHINE LEARNING-ICANN 2024, PT II(2024)
摘要
Existing NeRF-based instance segmentation methods lift 2D annotated or predicted semantic and instance masks into 3D through radiance field, but still insufficient in segmenting on the unlabeled semantic classes. The Segment Anything Model (SAM) introduces a foundation model for image segmentation, and achieves impressive zero-shot performance. In this paper, we take advantage of SAM and propose SAM-NeRF for 3D instance segmentation agnostic to semantic labels. Unlike existing SAM-based 3D instance segmentation methods which rely on user provided prompts, SAM-NeRF only uses 2D posed images and SAM generated segmentation results to render novel view images with 3D consistent instance masks. To account for the inconsistencies on the instance identifiers across views, we represent the 3D instances with a neural instance field, and propose a mask matching method to lift SAM segmentation results to 3D scene consistently without any semantic supervision. As SAM segments the scene under various granularity, causing divergence cross views, we further propose a merging algorithm to improve the 3D segmentation performance with unified granularity between different views. Without any semantic guidance, our method outperforms the start-of-the-art 2D and 3D supervised panoptic segmentation methods on unlabeled classes in all evaluated datasets.
更多查看译文
关键词
3D instance segmentation,NeRF,Segment Anything Model,Zero-shot segmentation
AI 理解论文
溯源树
样例
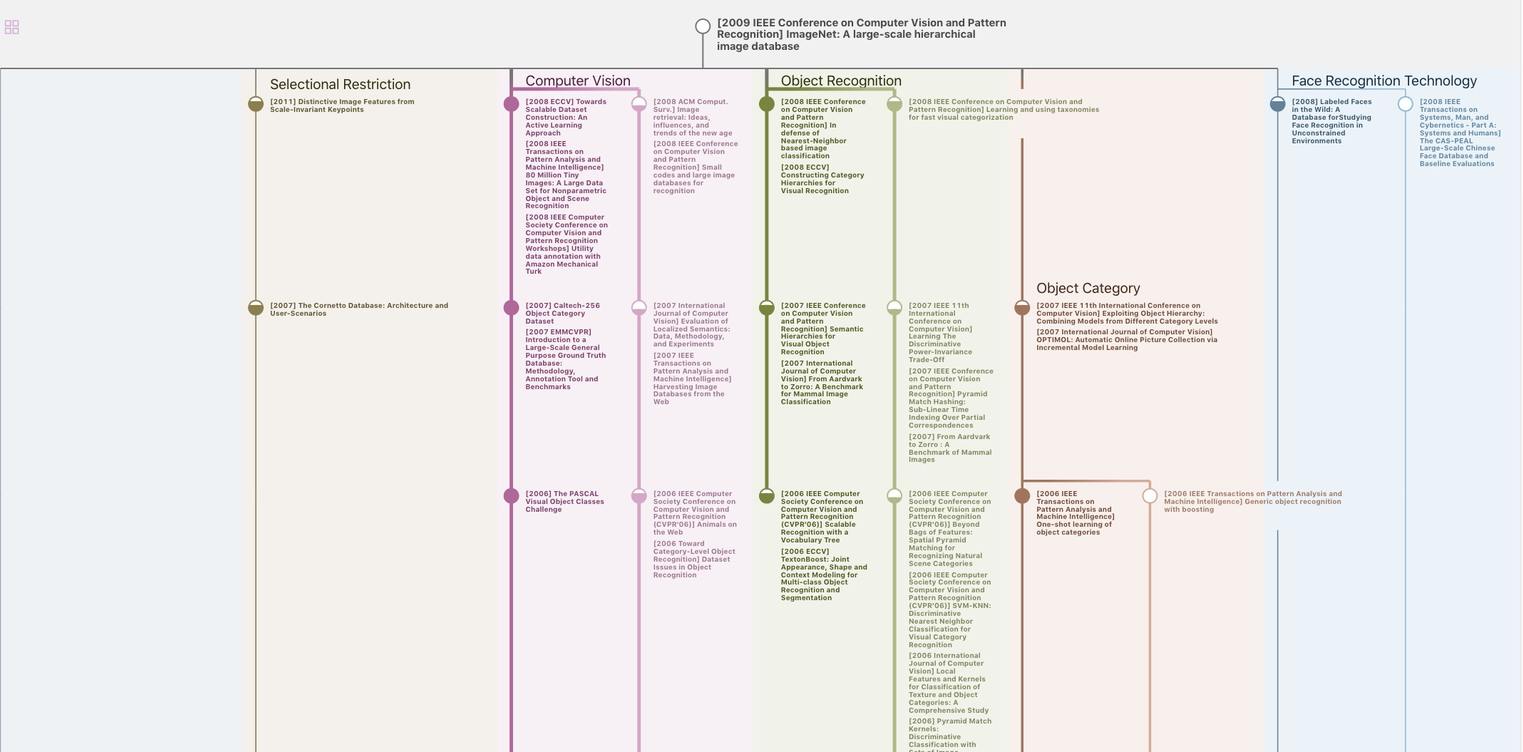
生成溯源树,研究论文发展脉络
数据免责声明
页面数据均来自互联网公开来源、合作出版商和通过AI技术自动分析结果,我们不对页面数据的有效性、准确性、正确性、可靠性、完整性和及时性做出任何承诺和保证。若有疑问,可以通过电子邮件方式联系我们:report@aminer.cn