A Criterion for Selecting the Appropriate One from the Trained Models for Model-Based Offline Policy Evaluation
CAAI TRANSACTIONS ON INTELLIGENCE TECHNOLOGY(2024)
摘要
Offline policy evaluation, evaluating and selecting complex policies for decision-making by only using offline datasets is important in reinforcement learning. At present, the model-based offline policy evaluation (MBOPE) is widely welcomed because of its easy to implement and good performance. MBOPE directly approximates the unknown value of a given policy using the Monte Carlo method given the estimated transition and reward functions of the environment. Usually, multiple models are trained, and then one of them is selected to be used. However, a challenge remains in selecting an appropriate model from those trained for further use. The authors first analyse the upper bound of the difference between the approximated value and the unknown true value. Theoretical results show that this difference is related to the trajectories generated by the given policy on the learnt model and the prediction error of the transition and reward functions at these generated data points. Based on the theoretical results, a new criterion is proposed to tell which trained model is better suited for evaluating the given policy. At last, the effectiveness of the proposed criterion is demonstrated on both benchmark and synthetic offline datasets.
更多查看译文
关键词
artificial inteligence,deep neural networks,machine learning
AI 理解论文
溯源树
样例
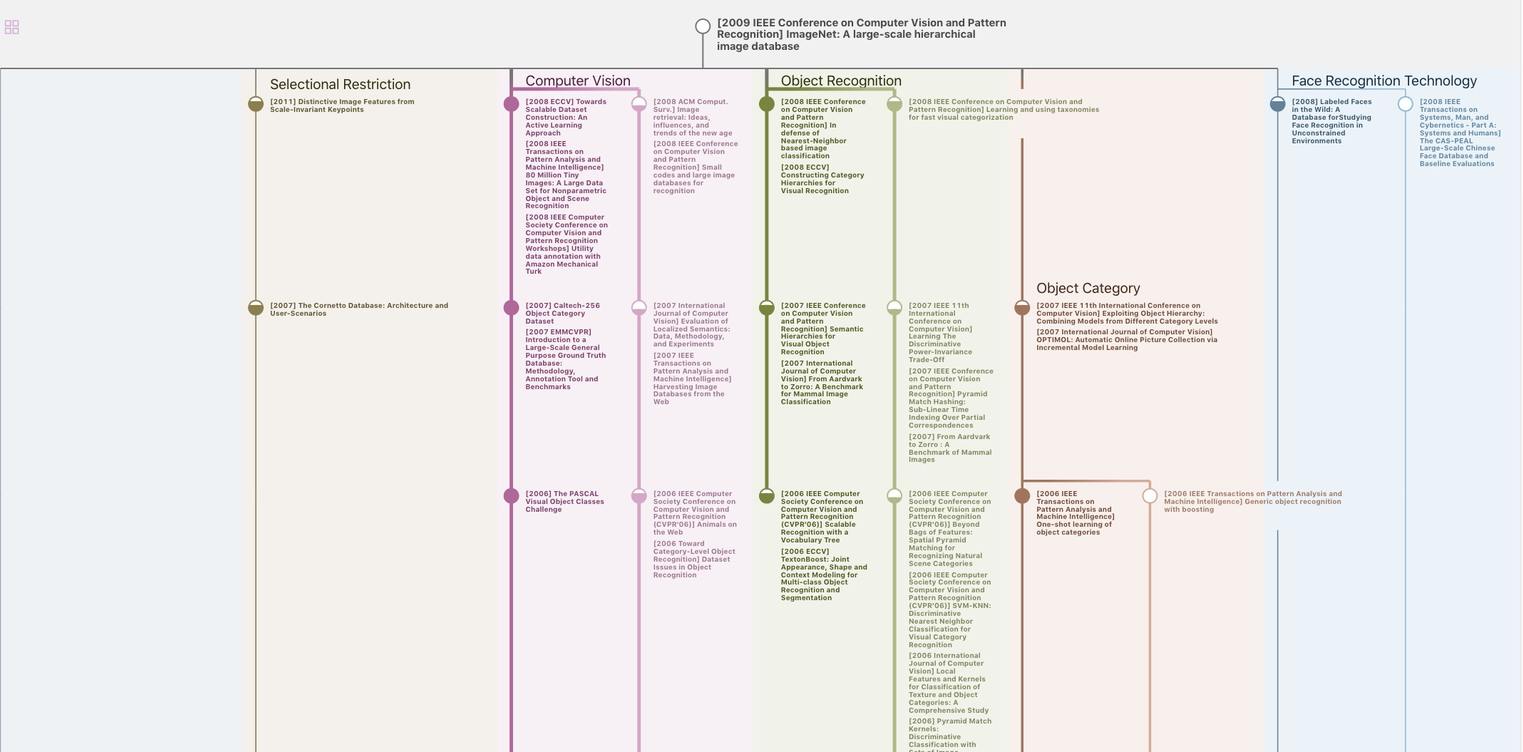
生成溯源树,研究论文发展脉络
数据免责声明
页面数据均来自互联网公开来源、合作出版商和通过AI技术自动分析结果,我们不对页面数据的有效性、准确性、正确性、可靠性、完整性和及时性做出任何承诺和保证。若有疑问,可以通过电子邮件方式联系我们:report@aminer.cn