JointFlow: Optimizing Service Deployment for Large-Scale Model Workflows in JointCloud
IEEE International Conference on Web Services(2024)
摘要
LLM-based workflows utilize Large Language Models (LLMs) for dynamic user requests, combining task planning and multiple machine learning (ML) models. Existing ML workflow platforms assume static structure deployment, neglecting dynamic orchestration for comprehensive workflows that fulfill users’ diverse requirements. Model selection in dynamic workflows involves various models and parallelism configurations, each with unique accuracy and efficiency trade-offs.To address these limitations, we introduce JointFlow, a solution offering LLM-based workflows as a service by dynamically constructing workflows across heterogeneous JointCloud infrastructures. It models and optimizes dynamic workflows, focusing on accuracy and efficiency trade-offs in model selection and parallelism configurations. A super-DAG represents the dynamic sub-task workflows from LLMs, profiling configurations across infrastructures. JointFlow also seeks the optimal workflow placement strategy. Experiments show it reduces serving costs while achieving throughput objectives compared to state-of-the-art methods.
更多查看译文
关键词
Large Language Models based workflow,model parallelism,resource provisioning,dynamic workflow,JointCloud
AI 理解论文
溯源树
样例
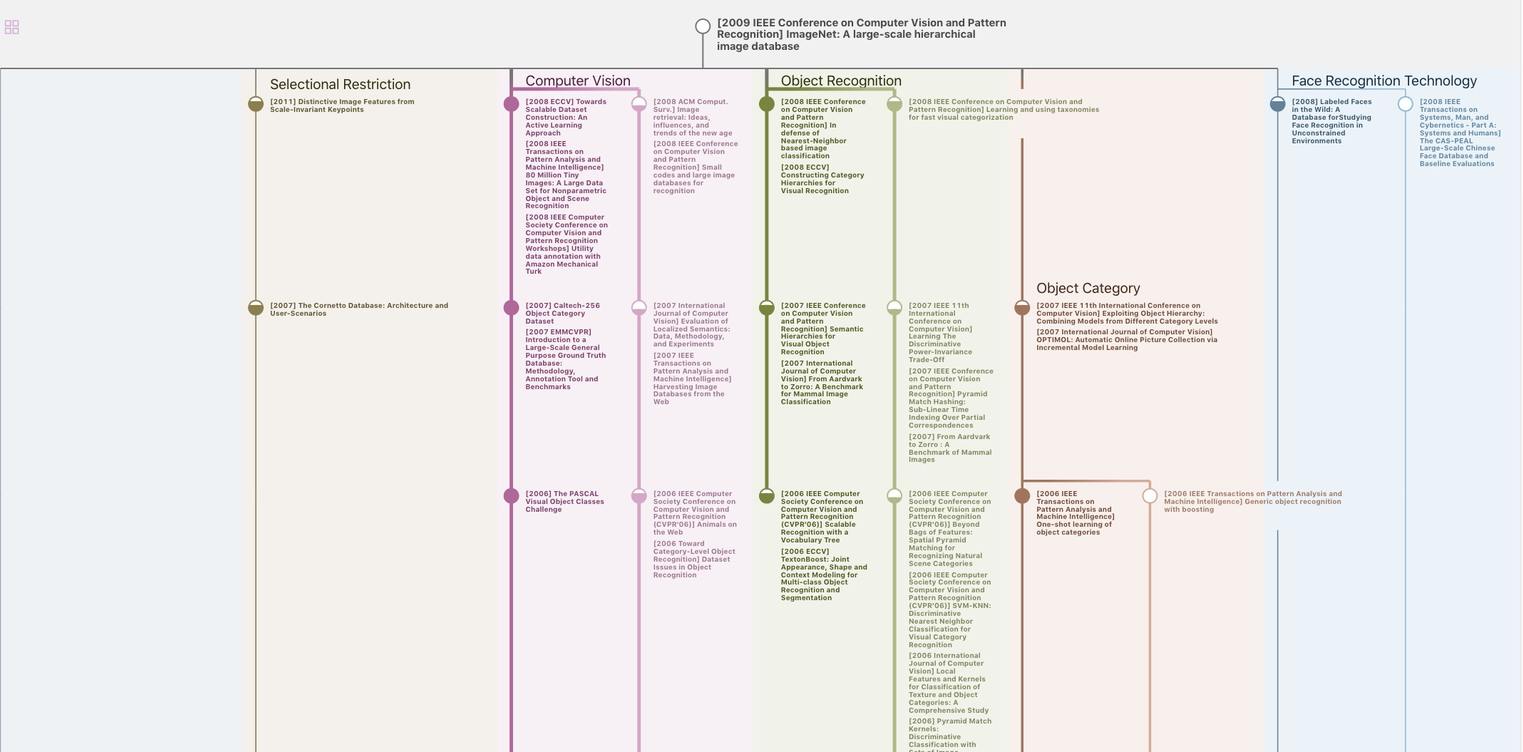
生成溯源树,研究论文发展脉络
数据免责声明
页面数据均来自互联网公开来源、合作出版商和通过AI技术自动分析结果,我们不对页面数据的有效性、准确性、正确性、可靠性、完整性和及时性做出任何承诺和保证。若有疑问,可以通过电子邮件方式联系我们:report@aminer.cn