Advancing the Understanding of Fixed Point Iterations in Deep Neural Networks: A Detailed Analytical Study
arxiv(2024)
摘要
Recent empirical studies have identified fixed point iteration phenomena in deep neural networks, where the hidden state tends to stabilize after several layers, showing minimal change in subsequent layers. This observation has spurred the development of practical methodologies, such as accelerating inference by bypassing certain layers once the hidden state stabilizes, selectively fine-tuning layers to modify the iteration process, and implementing loops of specific layers to maintain fixed point iterations. Despite these advancements, the understanding of fixed point iterations remains superficial, particularly in high-dimensional spaces, due to the inadequacy of current analytical tools. In this study, we conduct a detailed analysis of fixed point iterations in a vector-valued function modeled by neural networks. We establish a sufficient condition for the existence of multiple fixed points of looped neural networks based on varying input regions. Additionally, we expand our examination to include a robust version of fixed point iterations. To demonstrate the effectiveness and insights provided by our approach, we provide case studies that looped neural networks may exist 2^d number of robust fixed points under exponentiation or polynomial activation functions, where d is the feature dimension. Furthermore, our preliminary empirical results support our theoretical findings. Our methodology enriches the toolkit available for analyzing fixed point iterations of deep neural networks and may enhance our comprehension of neural network mechanisms.
更多查看译文
AI 理解论文
溯源树
样例
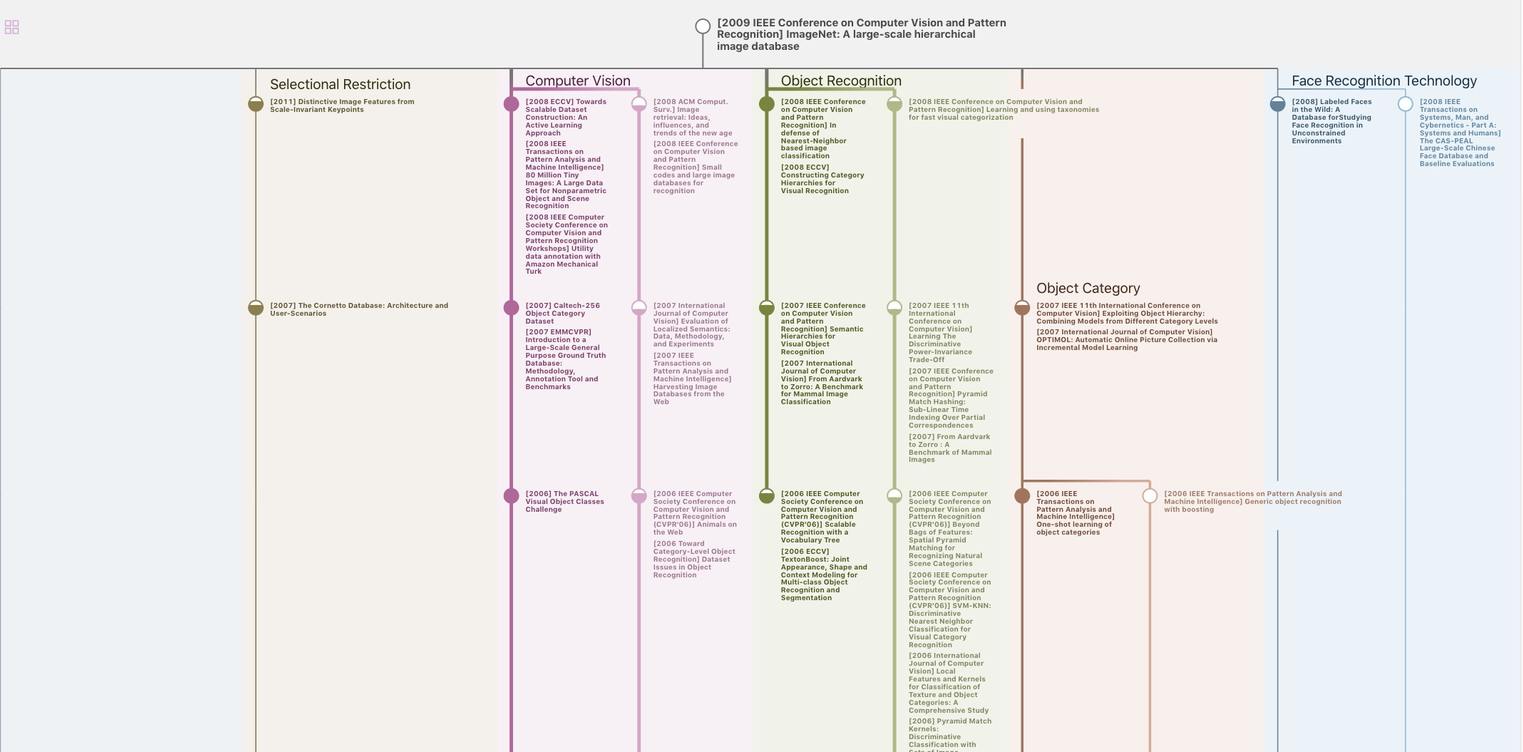
生成溯源树,研究论文发展脉络
数据免责声明
页面数据均来自互联网公开来源、合作出版商和通过AI技术自动分析结果,我们不对页面数据的有效性、准确性、正确性、可靠性、完整性和及时性做出任何承诺和保证。若有疑问,可以通过电子邮件方式联系我们:report@aminer.cn