AI Scheme for High-Accuracy and Contactless Assessment of Parkinson’s Disease Grades
BIOMEDICAL SIGNAL PROCESSING AND CONTROL(2025)
摘要
This paper addresses the significant challenges inaccurately and consistently assessing Parkinson's disease (PD) severity, particularly in elderly patients, where traditional diagnostic methods struggle with quantification and standardization. To overcome these limitations, we propose a novel, AI-based scheme for PD grade assessment that enhances accuracy and standardization while avoiding the complexities associated with wearable sensors. We collected a dataset of 110 videos from volunteers across different PD severity grades. Using MediaPipe, a contactless pose extraction model, we extracted 19 distinct kinematic features from joint movements to generate real-time kinematic data. A rigorous data screening phase was employed to eliminate irrelevant or noisy features, reducing the dimensionality of the dataset. We then evaluated PD grades using five different machine learning algorithms. Among these, the K-Nearest Neighbors (KNN) classifier achieved the highest performance, with an overall accuracy of 96.63% and 100% accuracy in classifying Grade 4 and Grade 5 patients. These results demonstrate the effectiveness of our AI-based scheme in providing accurate, contactless, and standardized PD severity assessments. Notably, the elimination of wearable sensors not only improves diagnostic accuracy but also enhances the feasibility of remote diagnosis, benefiting both physicians and patients. Our study underscores that the proposed AI solution represents a significant advancement in PD diagnosis by offering a reliable, sensor-free, and standardized assessment method, which is highly valuable for clinical use and remote management of PD.
更多查看译文
关键词
Parkinson's disease(PD),Kinematic features,Machine learning,Grade assessment
AI 理解论文
溯源树
样例
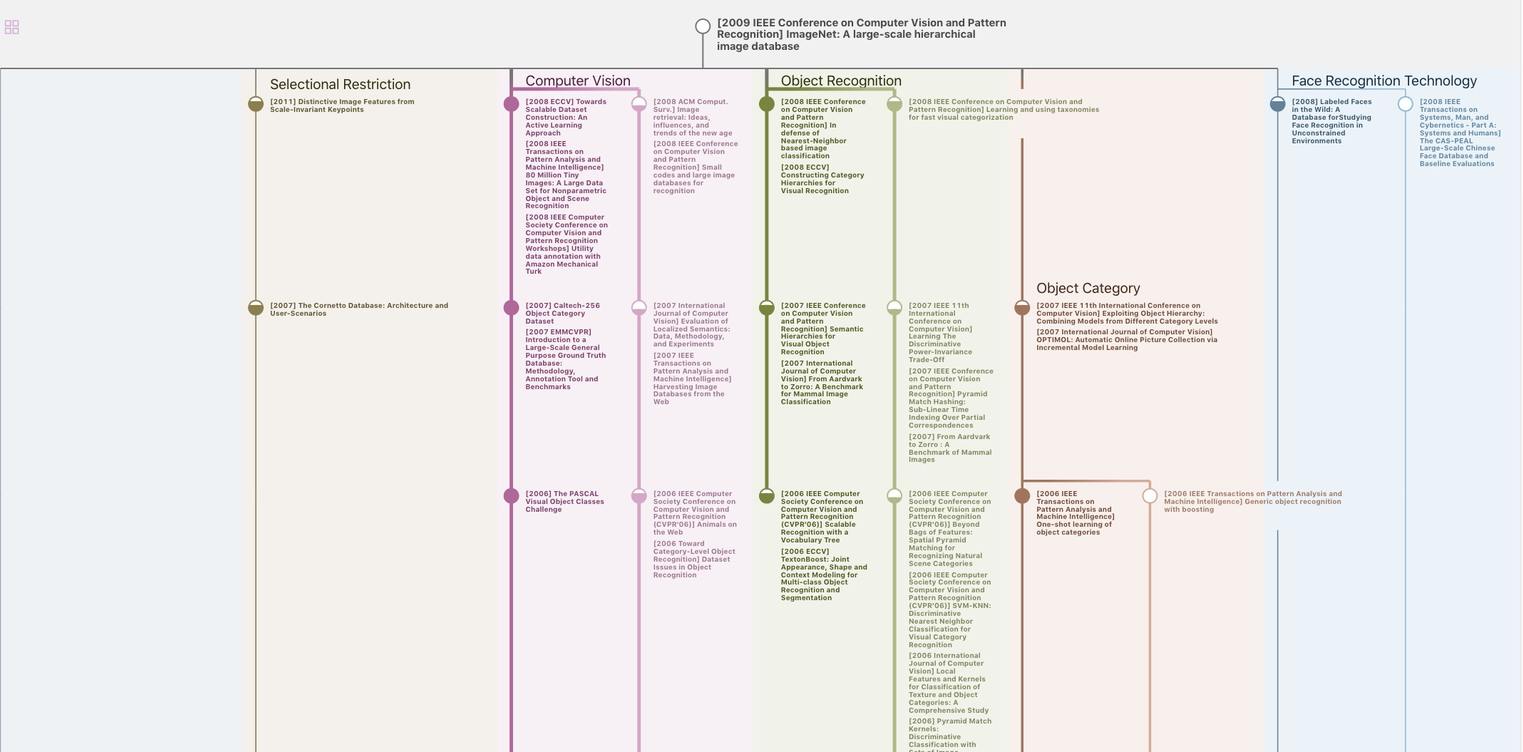
生成溯源树,研究论文发展脉络
数据免责声明
页面数据均来自互联网公开来源、合作出版商和通过AI技术自动分析结果,我们不对页面数据的有效性、准确性、正确性、可靠性、完整性和及时性做出任何承诺和保证。若有疑问,可以通过电子邮件方式联系我们:report@aminer.cn