Diagnosis and Biomarker Screening of Endometrial Cancer Enabled by a Versatile Exosome Metabolic Fingerprint Platform.
ANALYTICAL CHEMISTRY(2024)
摘要
Exosomes have emerged as a revolutionary tool for liquid biopsy (LB), as they carry specific cargo from cells. Profiling the metabolites of exosomes is crucial for cancer diagnosis and biomarker discovery. Herein, we propose a versatile platform for exosomal metabolite assay of endometrial cancer (EC). The platform is based on a nanostructured composite material comprising gold nanoparticle-coated magnetic COF with aptamer modification (Fe3O4@COF@Au-Apt). The unique design and novel synthesis strategy of Fe3O4@COF@Au-Apt provide the material with a large specific surface area, enabling the efficient and specific isolation of exosomes. The exosomes captured Fe3O4@COF@Au-Apt can be directly used as the laser desorption/ionization mass spectrometry (LDI-MS) matrix for rapid exosomal metabolic patterns. By integrating these functionalities into a single platform, the analytical process is simplified, eliminating the need for additional elution steps and minimizing potential sample loss, resulting in large-scale exosomal metabolic fingerprints. Combining with machine learning algorithms on the metabolic patterns, accurate discrimination between endometrial patients (EGs) and benign controls (CGs) was achieved, and the area under the receiver operating characteristic curve of the blind test cohort was 0.924. Confusion matrix analysis of important metabolic fingerprint features further demonstrates the high accuracy of the proposed approach toward EC diagnosis, with an overall accuracy of 94.1%. Moreover, four metabolites, namely, hydroxychalcone, l-acetylcarnitine, elaidic acid, and glutathione, have been identified as potential biomarkers of EC. These results highlight the great value of the integrated exosome metabolic fingerprint platform in facilitating low-cost and high-throughput characterization of exosomal metabolites for cancer diagnosis and biomarker discovery.
更多查看译文
AI 理解论文
溯源树
样例
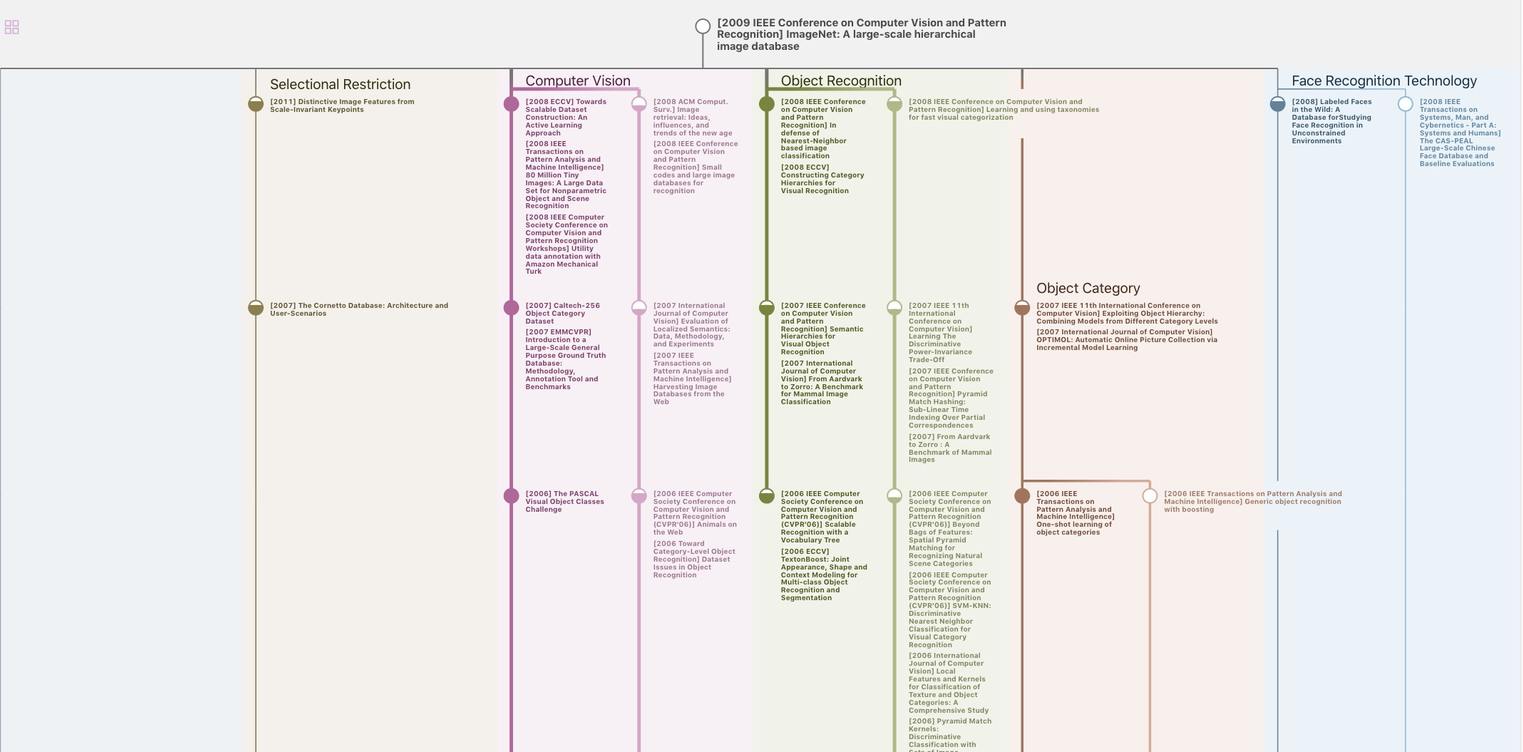
生成溯源树,研究论文发展脉络
数据免责声明
页面数据均来自互联网公开来源、合作出版商和通过AI技术自动分析结果,我们不对页面数据的有效性、准确性、正确性、可靠性、完整性和及时性做出任何承诺和保证。若有疑问,可以通过电子邮件方式联系我们:report@aminer.cn