Positional Diffusion: Graph-based Diffusion Models for Set Ordering
Pattern Recognit Lett(2024)
摘要
Positional reasoning is the process of ordering an unsorted set of parts into a consistent structure. To address this problem, we present Positional Diffusion, a plug-and-play graph formulation with Diffusion Probabilistic Models. Using a diffusion process, we add Gaussian noise to the set elements' position and map them to a random position in a continuous space. Positional Diffusion learns to reverse the noising process and recover the original positions through an Attention-based Graph Neural Network. To evaluate our method, we conduct extensive experiments on three different tasks and seven datasets, comparing our approach against the stateof-the-art methods for visual puzzle-solving, sentence ordering, and room arrangement, demonstrating that our method outperforms long-lasting research on puzzle solving with up to +17% compared to the secondbest deep learning method, and performs on par against the state-of-the-art methods on sentence ordering and room rearrangement. Our work highlights the suitability of diffusion models for ordering problems and proposes a novel formulation and method for solving various ordering tasks. We release our code at https://github.com/IIT-PAVIS/Positional_Diffusion.
更多查看译文
关键词
Graph neural networks,Diffusion probabilistic models,Positional reasoning
AI 理解论文
溯源树
样例
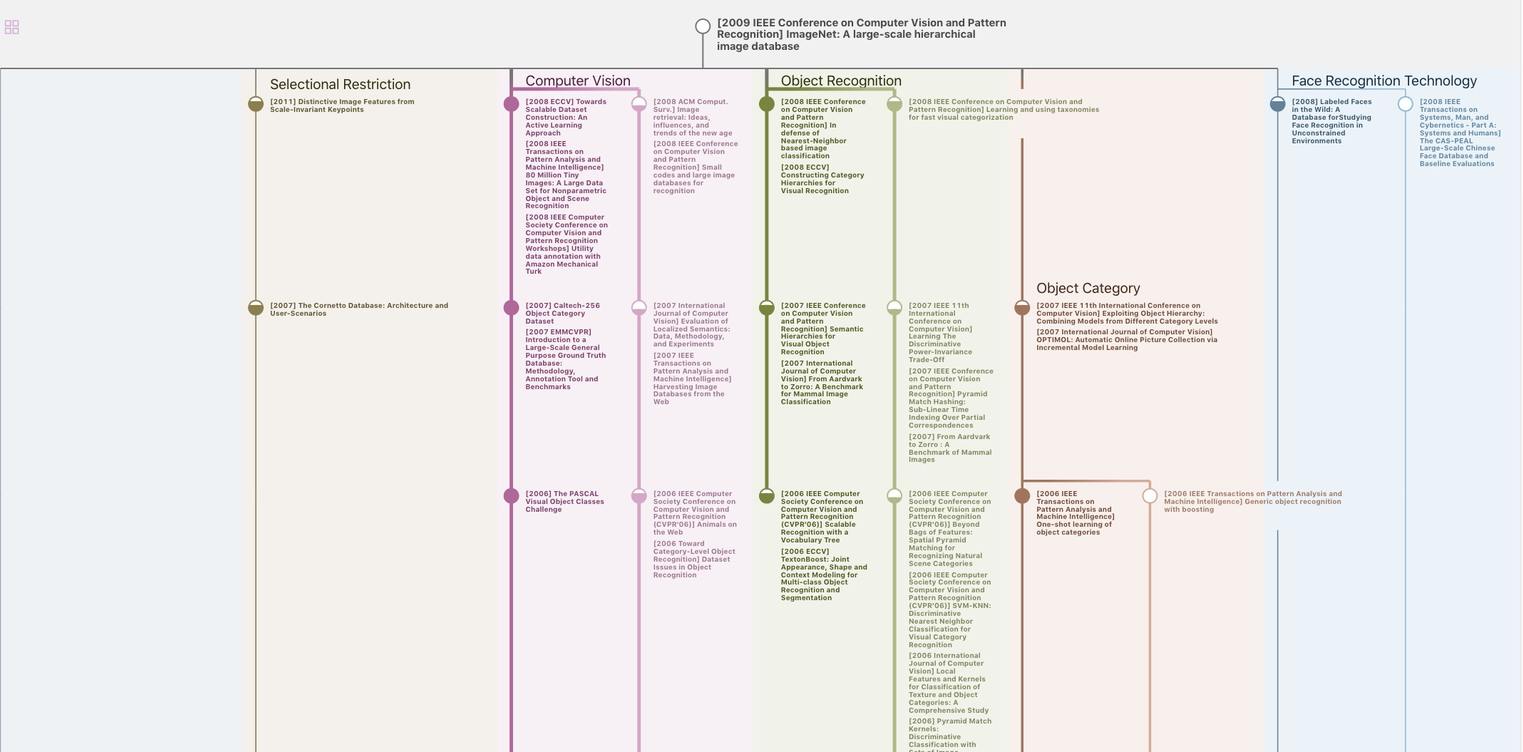
生成溯源树,研究论文发展脉络
数据免责声明
页面数据均来自互联网公开来源、合作出版商和通过AI技术自动分析结果,我们不对页面数据的有效性、准确性、正确性、可靠性、完整性和及时性做出任何承诺和保证。若有疑问,可以通过电子邮件方式联系我们:report@aminer.cn