Tracing Training Progress: Dynamic Influence Based Selection for Active Learning
ACM International Conference on Multimedia(2024)
摘要
Active learning (AL) aims to select highly informative data points from an unlabeled dataset for annotation, mitigating the need for extensive human labeling effort. However, classical AL methods heavily rely on human expertise to design the sampling strategy, inducing limited scalability and generalizability. Many efforts have sought to address this limitation by directly connecting sample selection with model performance improvement, typically through influence function. Nevertheless, these approaches often ignore the dynamic nature of model behavior during training optimization, despite empirical evidence highlights the importance of dynamic influence to track the sample contribution. This oversight can lead to suboptimal selection, hindering the generalizability of model. In this study, we explore the dynamic influence based data selection strategy by tracing the impact of unlabeled instances on model performance throughout the training process. Our theoretical analyses suggest that selecting samples with higher projected gradients along the accumulated optimization direction at each checkpoint leads to improved performance. Furthermore, to capture a wider range of training dynamics without incurring excessive computational or memory costs, we introduce an additional dynamic loss term designed to encapsulate more generalized training progress information. These insights are integrated into a universal and task-agnostic AL framework termed Dynamic Influence Scoring for Active Learning (DISAL). Comprehensive experiments across various tasks have demonstrated that DISAL significantly surpasses existing state-of-the-art AL methods, demonstrating its ability to facilitate more efficient and effective learning in different domains.
更多查看译文
AI 理解论文
溯源树
样例
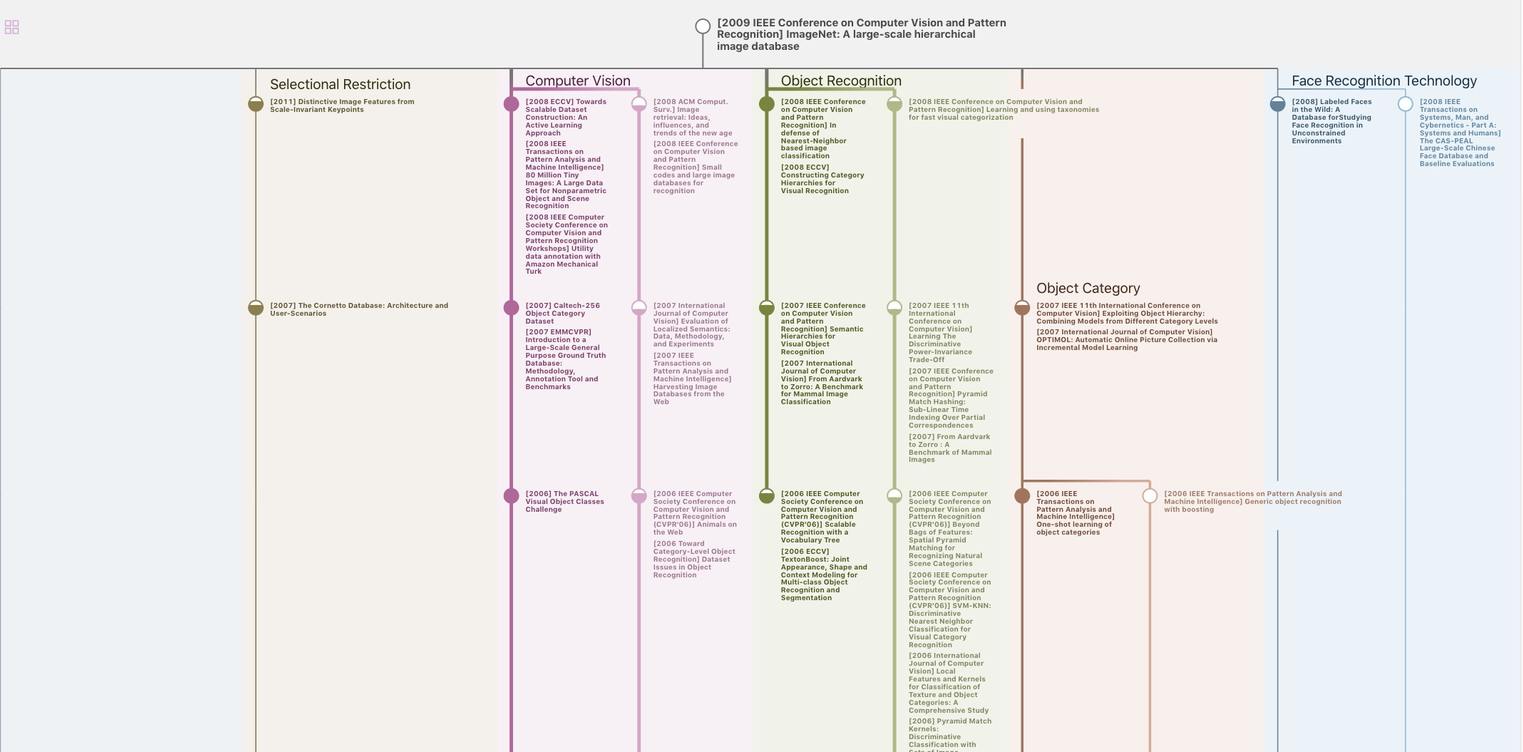
生成溯源树,研究论文发展脉络
数据免责声明
页面数据均来自互联网公开来源、合作出版商和通过AI技术自动分析结果,我们不对页面数据的有效性、准确性、正确性、可靠性、完整性和及时性做出任何承诺和保证。若有疑问,可以通过电子邮件方式联系我们:report@aminer.cn