Machine Learning Predicts Liver Cancer Risk from Routine Clinical Data: a Large Population-Based Multicentric Study
crossref(2024)
摘要
Background and aims: Hepatocellular carcinoma (HCC) is a highly fatal tumor, for which early detection and risk stratification is crucial, yet remains challenging. We aimed to develop an interpretable machine-learning framework for HCC risk stratification based on routinely collected clinical data. Methods: We leverage data obtained from over 900,000 individuals and 983 cases of HCC across two large-scale population-based cohorts: the UK Biobank study and the "All Of Us Research Program". For all of these patients, clinical data from timepoints years before diagnosis of HCC was available. We integrate data modalities including demographics, electronic health records, lifestyle, routine blood tests, genomics and metabolomics to offer a unique, multi-modal perspective on HCC risk. Results: Our random-forest-based model significantly outperforms all publicly available state-of-the-art risk-scores, with an AUROC of 0.88 both for internal and external test sets. We demonstrate robustness of our model across ethnic subgroups, a major advance over previous models with variable performance by ethnicity. Further, we perform extensive feature-importance analysis, showcasing our approach as an interpretable framework. We provide all model weights and an open-source web calculator to facilitate further validation of our model. Conclusion: Our study presents a robust and interpretable machine-learning framework for HCC risk stratification, which offers the potential to improve early detection and could ultimately reduce disease burden through targeted interventions. ### Competing Interest Statement JNK declares consulting services for Bioptimus, France; Owkin, France; DoMore Diagnostics, Norway; Panakeia, UK; AstraZeneca, UK; Mindpeak, Germany; and MultiplexDx, Slovakia. Furthermore, he holds shares in StratifAI GmbH, Germany, Synagen GmbH, Germany, and has received a research grant by GSK, and has received honoraria by AstraZeneca, Bayer, Daiichi Sankyo, Eisai, Janssen, Merck, MSD, BMS, Roche, Pfizer and Fresenius. TB has served on advisory boards for AdvanzPharma/Intercept Pharmaceuticals, SOBI, Novartis, and Gilead, and has received speaker fees from Falk Foundation, CSL Behring, Norgine, Intercept, Abbvie, Gilead, Merck, and Gore. OSMEN holds shares in StratifAI GmbH, Germany. Apichat Kaewdech re-ceived research grants or support from Roche, Roche Diagnostics, and Abbott Laboratories, and honoraria from Roche, Roche Diagnostics, Abbott Laboratories, and Esai. ### Clinical Protocols [https://github.com/schneiderlabac/hcc\_u\_soon][1] ### Funding Statement JC is supported by the Mildred-Scheel-Postdoktorandenprogramm of the German Cancer Aid (grant #70115730). JNK is supported by the German Federal Ministry of Health (DEEP LIVER, ZMVI1-2520DAT111), the Max-Eder-Programme of the German Cancer Aid (grant #70113864), the German Federal Ministry of Education and Research (PEARL, 01KD2104C; CAMINO, 01EO2101; SWAG, 01KD2215A; TRANSFORM LIVER, 031L0312A; TANGERINE, 01KT2302 through ERA-NET Transcan), the German Academic Exchange Service (SECAI, 57616814), the German Federal Joint Committee (Transplant.KI, 01VSF21048) the European Union's Horizon Europe and innovation programme (ODELIA, 101057091; GENIAL, 101096312) and the National Institute for Health and Care Research (NIHR, NIHR213331) Leeds Biomedical Research Centre. The views expressed are those of the author(s) and not necessarily those of the NHS, the NIHR or the Department of Health and Social Care. DT is supported by the German Federal Ministry of Education and Research (SWAG, 01KD2215A; TRANSFORM LIVER), the European Union's Horizon Europe and innovation programme (ODELIA, 101057091). TL was funded by the German Cancer Aid (Deutsche Krebshilfe-DECADE 70115166), the Federal Ministry of Education and Research (BMBF - TRANSFORM LIVER 031L0312B) and the Federal Ministry of Health (BMG - DEEP LIVER 2520DAT111). TB is supported by the German Research Foundation (SFB1382 Project ID 403224013/B07). C.V.S is supported by a grant from the Interdisciplinary Centre for Clinical Research within the faculty of Medicine at the RWTH Aachen University (PTD 1-13/IA 532313), the Junior Principal Investigator Fellowship program of RWTH Aachen Excellence strategy and the NRW Rueckkehr Programme of the Ministry of Culture and Science of the German State of North Rhine-Westphalia. K.M.S is supported by the Federal Ministry of Education and Research (BMBF) and the Ministry of Culture and Science of the German State of North Rhine-Westphalia under the Excellence strategy of the federal government and the Laender as well as the NRW Rueckkehr Programme of the Ministry of Culture and Science of the German State of North Rhine-Westphalia. C.V.S and K.M.S are supported by the CRC 1382 project A11 and B09 funded by Deutsche Forschungsgesellschaft (DFG, German Research Foundation) - Project-ID 403224013 - FB 1382". D.Y.Z. is supported by the National Heart, Lung, and Blood Institute of the National Institute of Health under award number F30HL172382. ### Author Declarations I confirm all relevant ethical guidelines have been followed, and any necessary IRB and/or ethics committee approvals have been obtained. Yes The details of the IRB/oversight body that provided approval or exemption for the research described are given below: UK Biobank data, including NMR metabolomics, are publicly available to bona fide researchers upon application at http://www.ukbiobank.ac.uk/using-the-resource/. Detailed information on predictors and endpoints used in this study is presented in Supplementary Tables 1-25. This study used data from the All of Us Research Pro-gram's Controlled Tier Dataset v7, available to authorized users on the Researcher Workbench. I confirm that all necessary patient/participant consent has been obtained and the appropriate institutional forms have been archived, and that any patient/participant/sample identifiers included were not known to anyone (e.g., hospital staff, patients or participants themselves) outside the research group so cannot be used to identify individuals. Yes I understand that all clinical trials and any other prospective interventional studies must be registered with an ICMJE-approved registry, such as ClinicalTrials.gov. I confirm that any such study reported in the manuscript has been registered and the trial registration ID is provided (note: if posting a prospective study registered retrospectively, please provide a statement in the trial ID field explaining why the study was not registered in advance). Yes I have followed all appropriate research reporting guidelines, such as any relevant EQUATOR Network research reporting checklist(s) and other pertinent material, if applicable. Yes UK Biobank data, including NMR metabolomics, are publicly available to bona fide researchers upon application at http://www.ukbiobank.ac.uk/using-the-resource/. Detailed information on predictors and endpoints used in this study is presented in Supplementary Tables 1-25. This study used data from the All of Us Research Program's Controlled Tier Dataset v7, available to authorized users on the Researcher Workbench. [1]: https://github.com/schneiderlabac/hcc_u_soon
更多查看译文
AI 理解论文
溯源树
样例
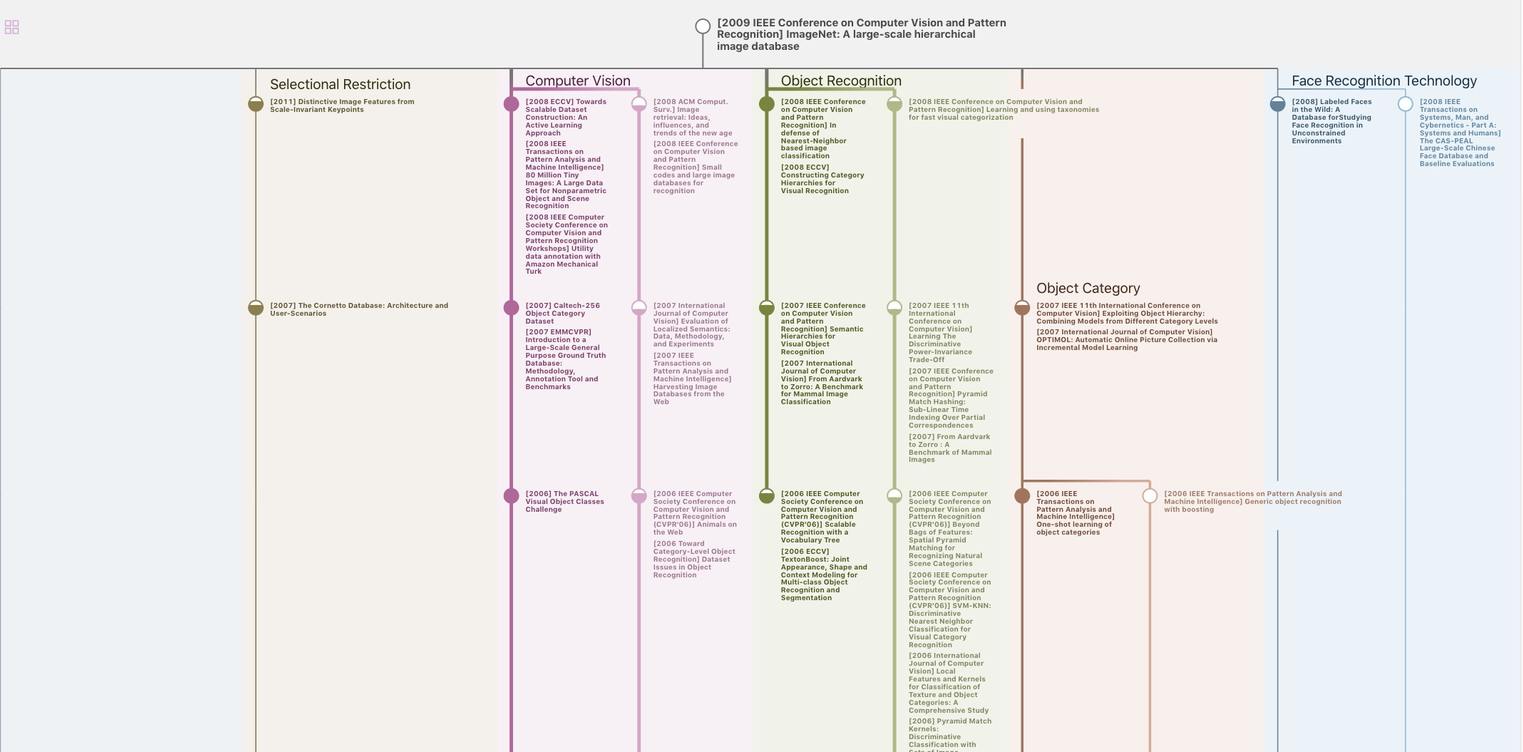
生成溯源树,研究论文发展脉络
数据免责声明
页面数据均来自互联网公开来源、合作出版商和通过AI技术自动分析结果,我们不对页面数据的有效性、准确性、正确性、可靠性、完整性和及时性做出任何承诺和保证。若有疑问,可以通过电子邮件方式联系我们:report@aminer.cn