Decoding Liver Cancer Prognosis: from Multi-omics Subtypes, Prognostic Models to Single Cell Validation
biorxiv(2024)
摘要
Purpose: Hepatocellular carcinoma (HCC) is a highly aggressive tumor characterized by significant heterogeneity and invasiveness, leading to a lack of precise individualized treatment strategies and poor patient outcomes. This necessitates the urgent development of accurate patient stratification methods and targeted therapies based on distinct tumor characteristics. Experimental Design: By integrating gene expression data from The Cancer Genome Atlas (TCGA), International Cancer Genome Consortium (ICGC), and Gene Expression Omnibus (GEO), we identified subtypes through a multi-omics consensus clustering approach amalgamated from 10 clustering techniques. Subsequently, we developed a prognostic model, employing machine learning algorithms, based on subtype classification features. Finally, by analyzing single cell sequencing data, we investigated the mechanisms driving prognostic variations among distinct subtypes. Results: First, we developed a novel consensus clustering method that categorizes liver cancer patients into two subtypes, CS1 and CS2. Second, we constructed a prognostic prediction model, which demonstrated superior predictive accuracy compared to several models published in the past five years. Finally, we observed differences between CS1 and CS2 in various metabolic pathways, biological processes, and signaling pathways, such as fatty acid metabolism, hypoxia levels, and the PI3K-AKT signaling pathway. ### Competing Interest Statement The authors have declared no competing interest.
更多查看译文
AI 理解论文
溯源树
样例
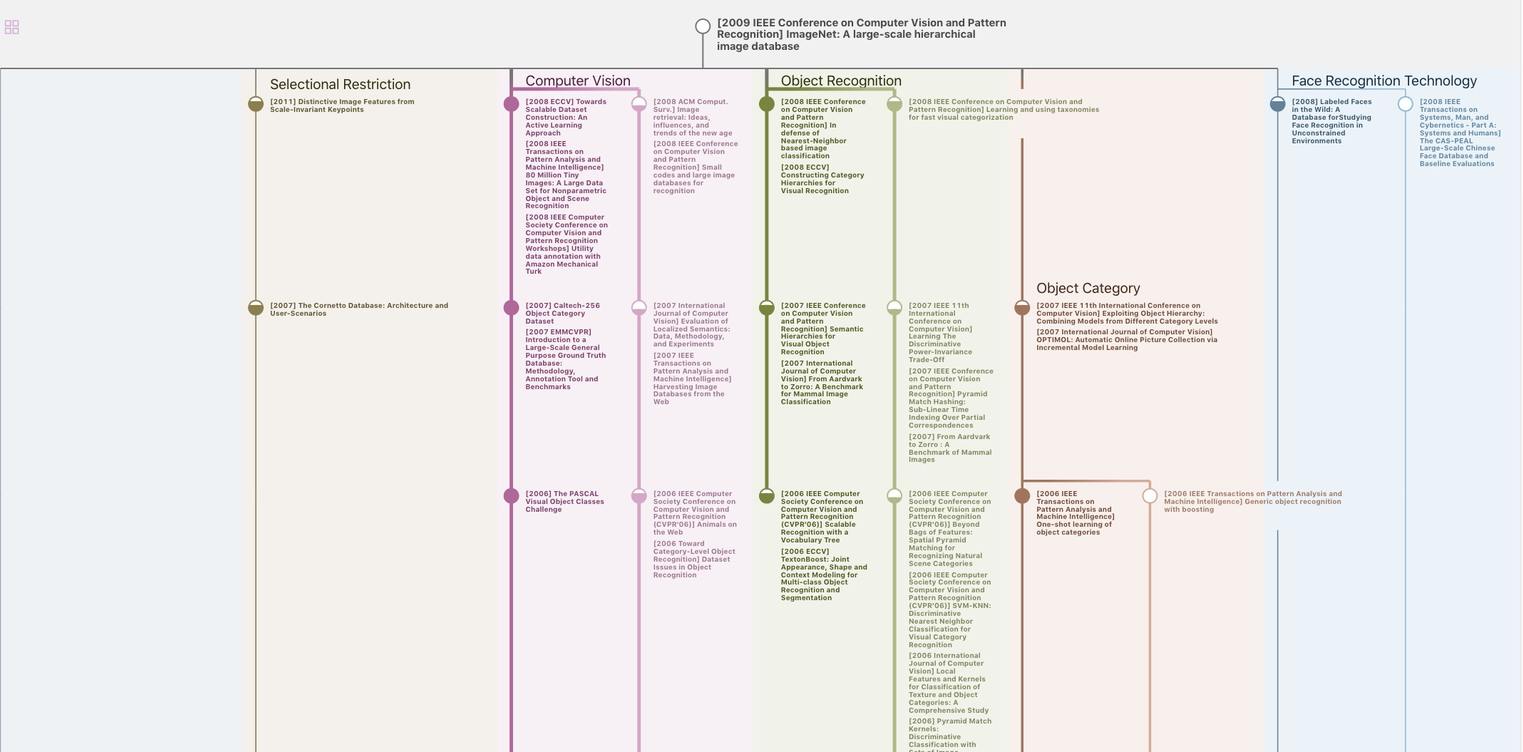
生成溯源树,研究论文发展脉络
数据免责声明
页面数据均来自互联网公开来源、合作出版商和通过AI技术自动分析结果,我们不对页面数据的有效性、准确性、正确性、可靠性、完整性和及时性做出任何承诺和保证。若有疑问,可以通过电子邮件方式联系我们:report@aminer.cn