Bringing Dynamic Sparsity to the Forefront for Low-Power Audio Edge Computing: Brain-inspired Approach for Sparsifying Network Updates
IEEE Solid-State Circuits Magazine(2024)
摘要
Dynamic sparsity is intrinsic to biological computing and is key to its extreme power efficiency. Edge computing systems can improve their energy efficiency and reduce response latency by exploiting this neuromorphic principle. The neuromorphic approach for the extraction of acoustic features replaces conventional ADC and DSP with biological cochlea-inspired filters and event generators implemented in mixed-signal circuits. The resulting sparse feature events drive inference in dynamic-sparsity-aware neural network accelerators to reduce computational load and memory access. The demonstration of edge keyword spotting shows the dynamic savings in power. Exploiting dynamic sparsity at all levels will be the next step toward the design of intelligent devices for the edge.
更多查看译文
关键词
Edge Computing,Neural Network,Energy Efficiency,Neuron Model,Computational Memory,Extraction Approach,System Energy Efficiency,Synchronization,Convolutional Neural Network,Artificial Neural Network,Convolutional Layers,Utterances,Recurrent Neural Network,Cochlea,Cochlear Implant,Front End,Target Neurons,Dynamic Power,Speech Samples,Digital Circuits,Sparse Weight,Speech Detection,Analog Circuits
AI 理解论文
溯源树
样例
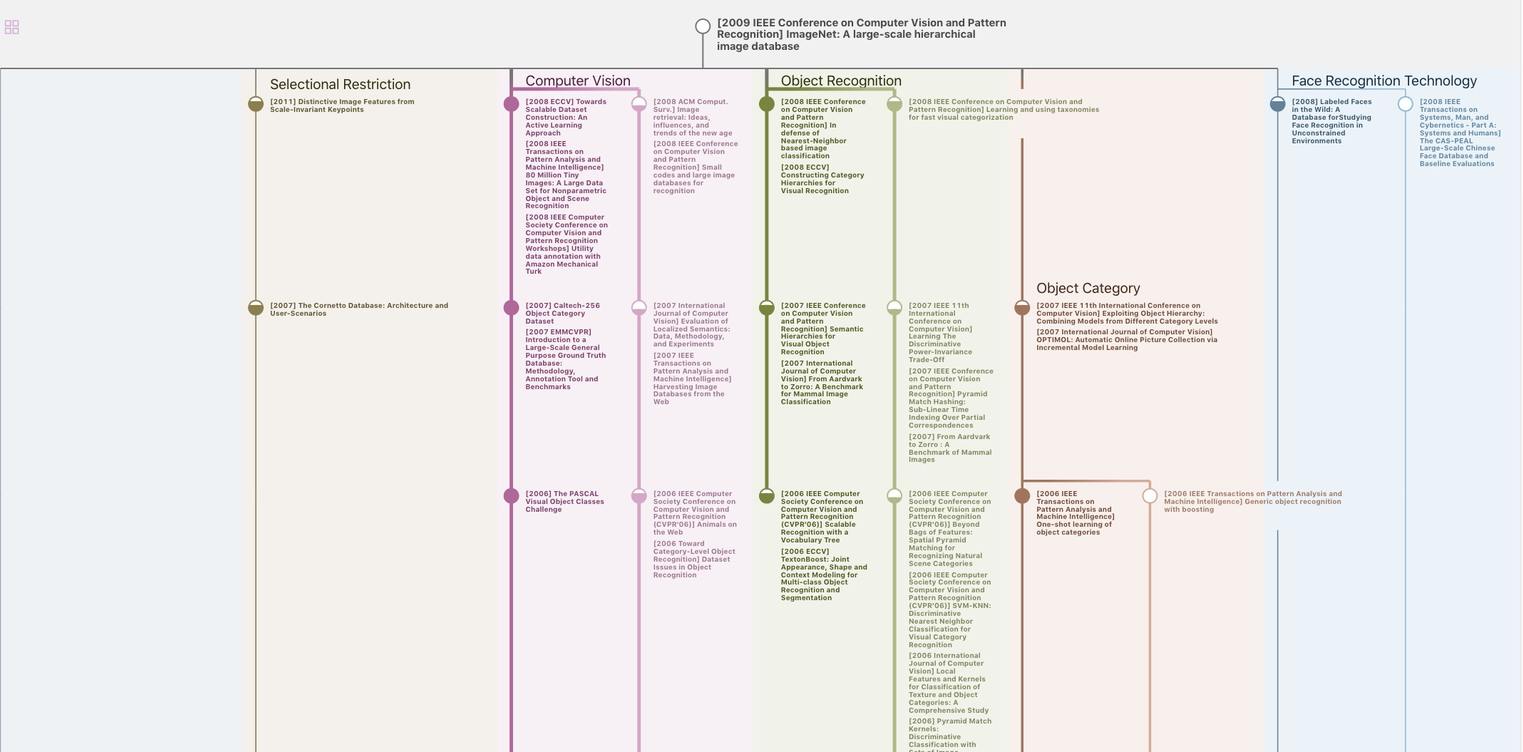
生成溯源树,研究论文发展脉络
数据免责声明
页面数据均来自互联网公开来源、合作出版商和通过AI技术自动分析结果,我们不对页面数据的有效性、准确性、正确性、可靠性、完整性和及时性做出任何承诺和保证。若有疑问,可以通过电子邮件方式联系我们:report@aminer.cn